Conversational Semantic Parsing using Dynamic Context Graphs.
CoRR(2023)
摘要
In this paper we consider the task of conversational semantic parsing over general purpose knowledge graphs (KGs) with millions of entities, and thousands of relation-types. We are interested in developing models capable of interactively mapping user utterances into executable logical forms (e.g., SPARQL) in the context of the conversational history. Our key idea is to represent information about an utterance and its context via a subgraph which is created dynamically, i.e., the number of nodes varies per utterance. Moreover, rather than treating the subgraph as a sequence we exploit its underlying structure, and thus encode it using a graph neural network which further allows us to represent a large number of (unseen) nodes. Experimental results show that modeling context dynamically is superior to static approaches, delivering performance improvements across the board (i.e., for simple and complex questions). Our results further confirm that modeling the structure of context is better at processing discourse information, (i.e., at handling ellipsis and resolving coreference) and longer interactions.
更多查看译文
关键词
context,dynamic
AI 理解论文
溯源树
样例
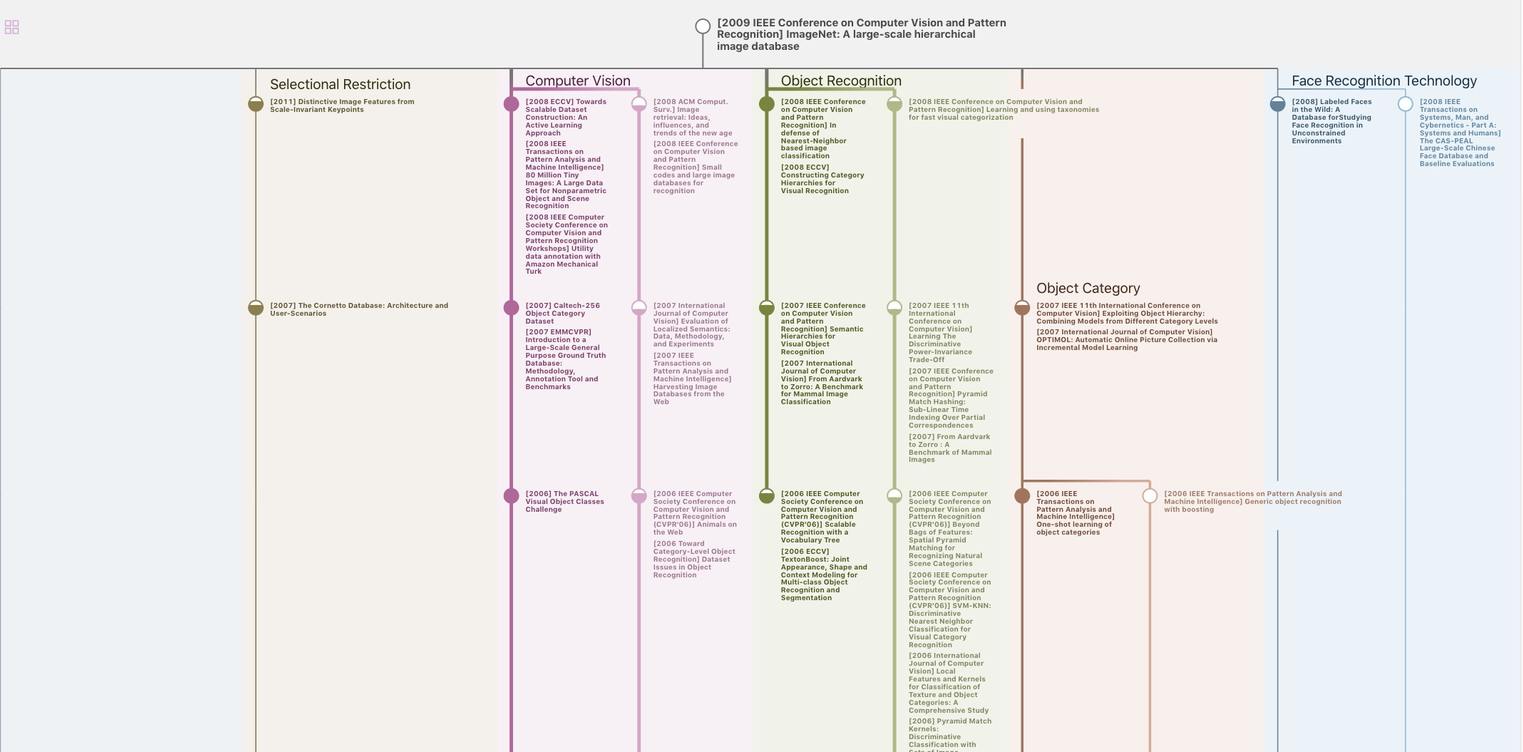
生成溯源树,研究论文发展脉络
Chat Paper
正在生成论文摘要