Synthetic Query Generation for Privacy-Preserving Deep Retrieval Systems using Differentially Private Language Models
CoRR(2023)
摘要
We address the challenge of ensuring differential privacy (DP) guarantees in
training deep retrieval systems. Training these systems often involves the use
of contrastive-style losses, which are typically non-per-example decomposable,
making them difficult to directly DP-train with since common techniques require
per-example gradient. To address this issue, we propose an approach that
prioritizes ensuring query privacy prior to training a deep retrieval system.
Our method employs DP language models (LMs) to generate private synthetic
queries representative of the original data. These synthetic queries can be
used in downstream retrieval system training without compromising privacy. Our
approach demonstrates a significant enhancement in retrieval quality compared
to direct DP-training, all while maintaining query-level privacy guarantees.
This work highlights the potential of harnessing LMs to overcome limitations in
standard DP-training methods.
更多查看译文
关键词
synthetic query generation
AI 理解论文
溯源树
样例
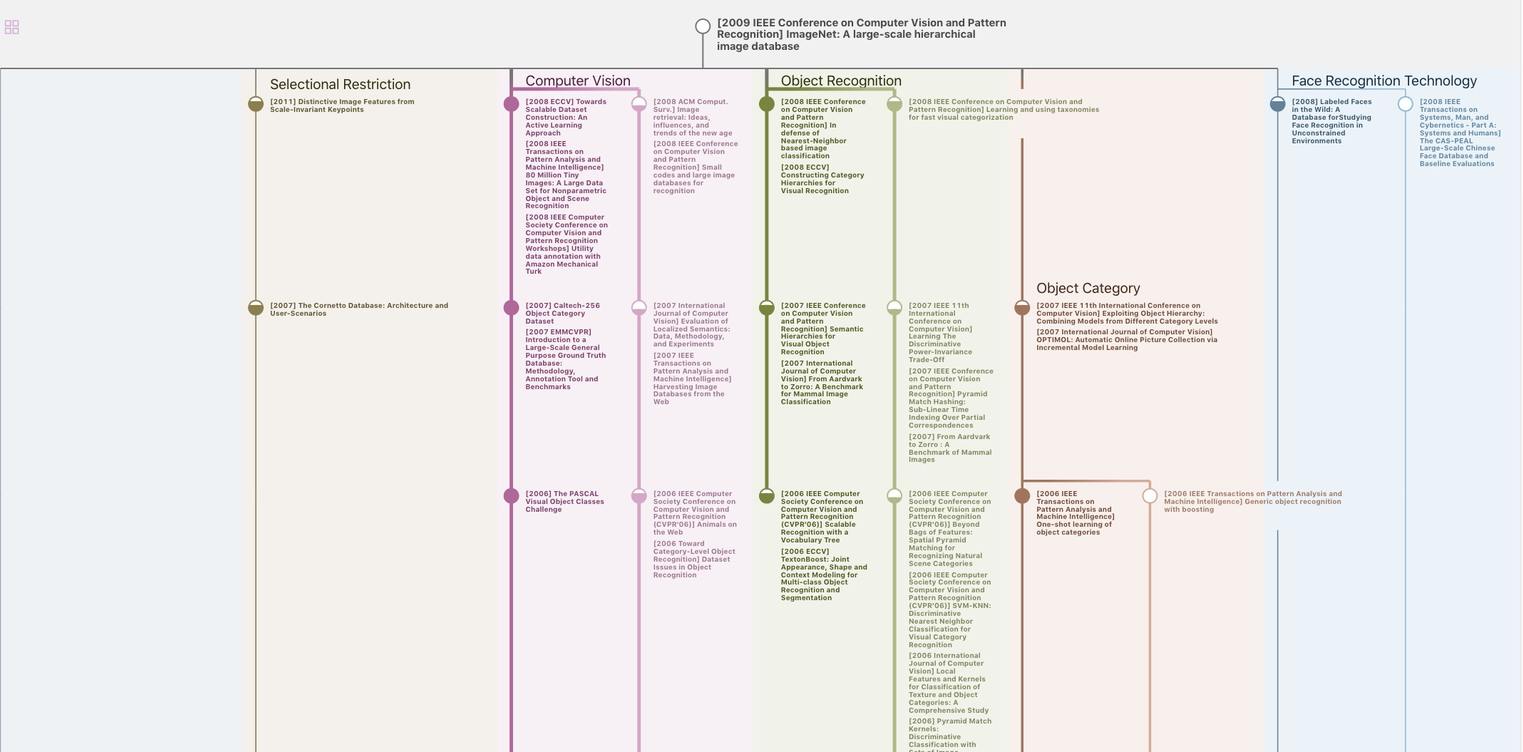
生成溯源树,研究论文发展脉络
Chat Paper
正在生成论文摘要