Multi-hop Commonsense Knowledge Injection Framework for Zero-Shot Commonsense Question Answering
CoRR(2023)
摘要
Commonsense question answering (QA) research requires machines to answer questions based on commonsense knowledge. However, this research requires expensive labor costs to annotate data as the basis of research, and models that rely on fine-tuning paradigms only apply to specific tasks, rather than learn a general commonsense reasoning ability. As a more robust method, zero-shot commonsense question answering shows a good prospect. The current zero-shot framework tries to convert triples in commonsense knowledge graphs (KGs) into QA-form samples as the pre-trained data source to incorporate commonsense knowledge into the model. However, this method ignores the multi-hop relationship in the KG, which is also an important central problem in commonsense reasoning. In this paper, we propose a novel multi-hop commonsense knowledge injection framework. Specifically, it explores multi-hop reasoning paradigm in KGs that conform to linguistic logic, and we further propose two multi-hop QA generation methods based on KGs. Then, we utilize contrastive learning to pre-train the model with the synthetic QA dataset to inject multi-hop commonsense knowledge. Extensive experiments on five commonsense question answering benchmarks demonstrate that our framework achieves state-of-art performance.
更多查看译文
关键词
commonsense question answering,question answering,knowledge,multi-hop,zero-shot
AI 理解论文
溯源树
样例
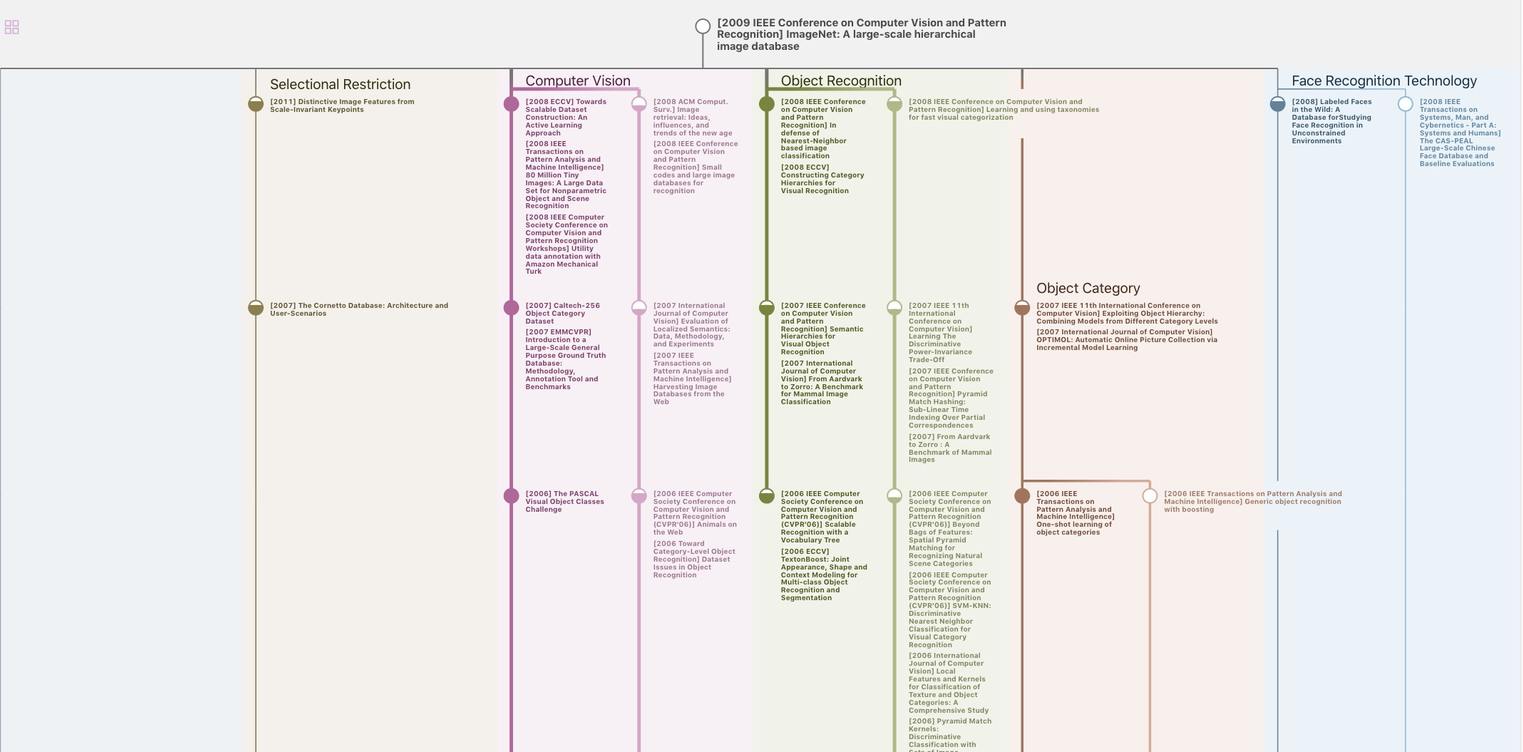
生成溯源树,研究论文发展脉络
Chat Paper
正在生成论文摘要