Causal Information Splitting: Engineering Proxy Features for Robustness to Distribution Shifts
CoRR(2023)
摘要
Statistical prediction models are often trained on data that is drawn from different probability distributions than their eventual use cases. One approach to proactively prepare for these shifts harnesses the intuition that causal mechanisms should remain invariant between environments. Here we focus on a challenging setting in which the causal and anticausal variables of the target are unobserved. Leaning on information theory, we develop feature selection and engineering techniques for the observed downstream variables that act as proxies. We identify proxies that help to build stable models and moreover utilize auxiliary training tasks to extract stability-enhancing information from proxies. We demonstrate the effectiveness of our techniques on synthetic and real data.
更多查看译文
关键词
causal information splitting,engineering proxy features,robustness
AI 理解论文
溯源树
样例
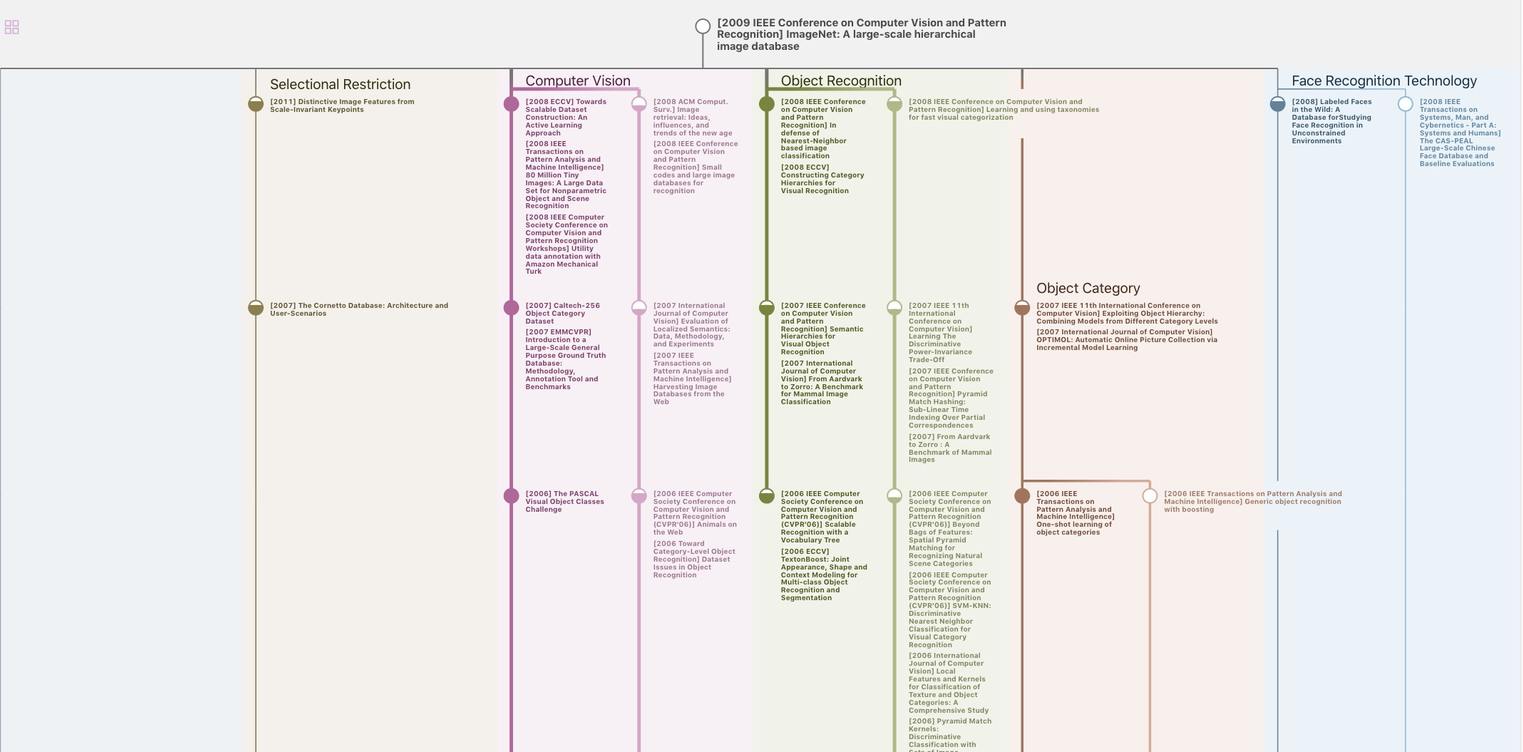
生成溯源树,研究论文发展脉络
Chat Paper
正在生成论文摘要