Metric Space Magnitude and Generalisation in Neural Networks
CoRR(2023)
摘要
Deep learning models have seen significant successes in numerous applications, but their inner workings remain elusive. The purpose of this work is to quantify the learning process of deep neural networks through the lens of a novel topological invariant called magnitude. Magnitude is an isometry invariant; its properties are an active area of research as it encodes many known invariants of a metric space. We use magnitude to study the internal representations of neural networks and propose a new method for determining their generalisation capabilities. Moreover, we theoretically connect magnitude dimension and the generalisation error, and demonstrate experimentally that the proposed framework can be a good indicator of the latter.
更多查看译文
关键词
metric space magnitude,neural networks,generalisation
AI 理解论文
溯源树
样例
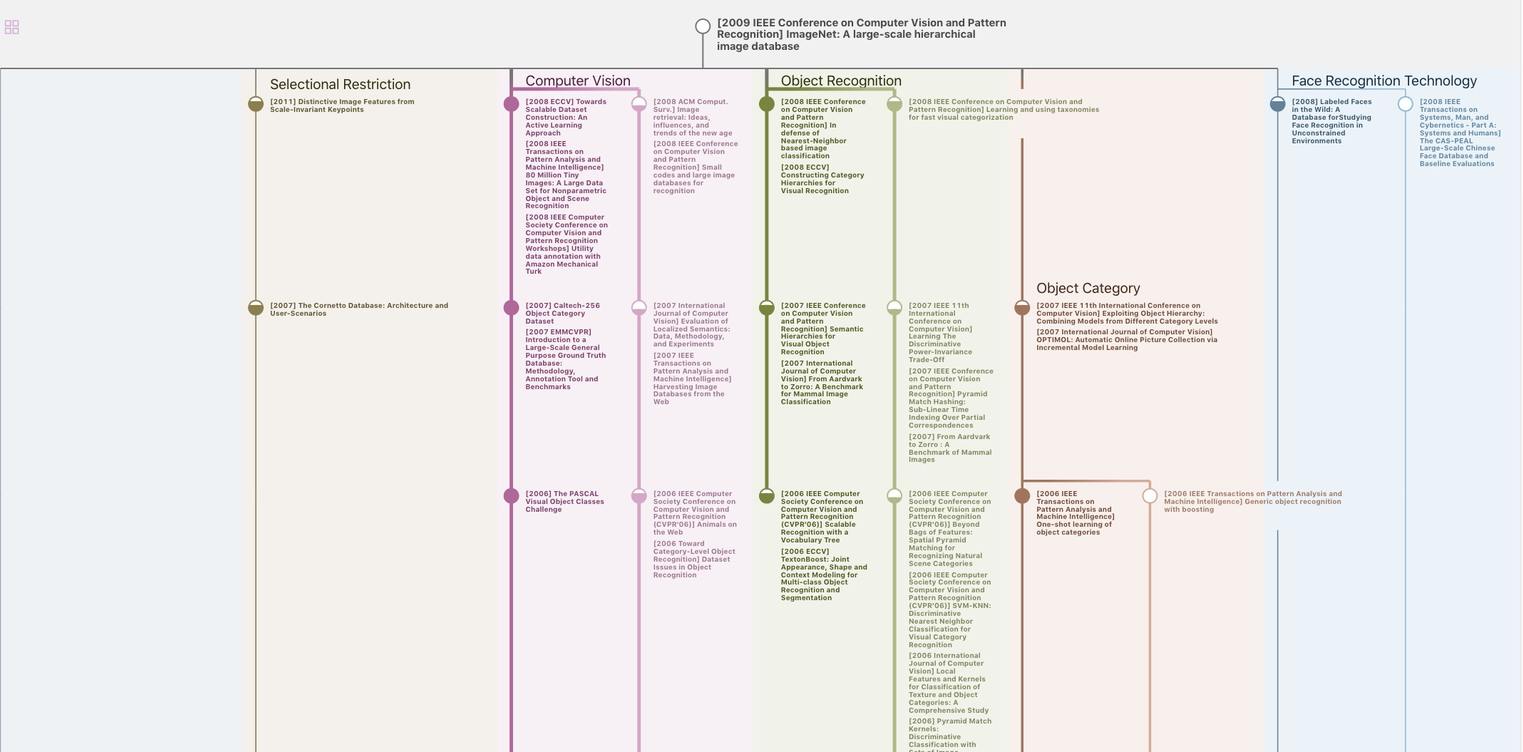
生成溯源树,研究论文发展脉络
Chat Paper
正在生成论文摘要