High-throughput Cotton Phenotyping Big Data Pipeline Lambda Architecture Computer Vision Deep Neural Networks
CoRR(2023)
摘要
In this study, we propose a big data pipeline for cotton bloom detection using a Lambda architecture, which enables real-time and batch processing of data. Our proposed approach leverages Azure resources such as Data Factory, Event Grids, Rest APIs, and Databricks. This work is the first to develop and demonstrate the implementation of such a pipeline for plant phenotyping through Azure's cloud computing service. The proposed pipeline consists of data preprocessing, object detection using a YOLOv5 neural network model trained through Azure AutoML, and visualization of object detection bounding boxes on output images. The trained model achieves a mean Average Precision (mAP) score of 0.96, demonstrating its high performance for cotton bloom classification. We evaluate our Lambda architecture pipeline using 9000 images yielding an optimized runtime of 34 minutes. The results illustrate the scalability of the proposed pipeline as a solution for deep learning object detection, with the potential for further expansion through additional Azure processing cores. This work advances the scientific research field by providing a new method for cotton bloom detection on a large dataset and demonstrates the potential of utilizing cloud computing resources, specifically Azure, for efficient and accurate big data processing in precision agriculture.
更多查看译文
关键词
deep neural networks,neural networks,high-throughput
AI 理解论文
溯源树
样例
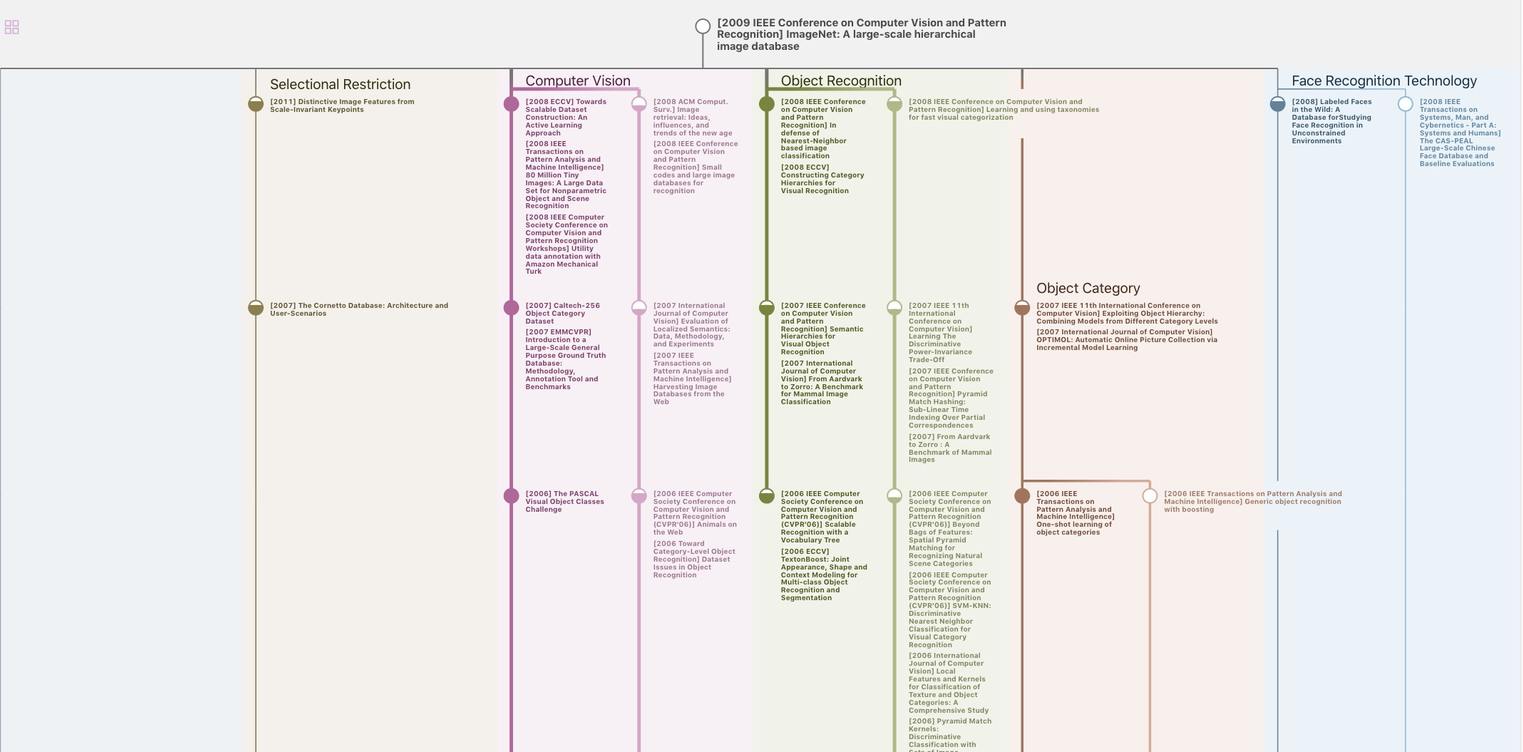
生成溯源树,研究论文发展脉络
Chat Paper
正在生成论文摘要