Trustworthy multi-phase liver tumor segmentation via evidence-based uncertainty
Engineering Applications of Artificial Intelligence(2024)
摘要
Multi-phase liver contrast-enhanced computed tomography (CECT) images convey the complementary multi-phase information for liver tumor segmentation (LiTS), which are crucial to assist the diagnosis of liver cancer clinically. However, the performances of existing multi-phase liver tumor segmentation (MPLiTS)-based methods suffer from redundancy and weak interpretability, resulting in the implicit unreliability of clinical applications. In this paper, we propose a novel trustworthy multi-phase liver tumor segmentation (TMPLiTS), which is a unified framework jointly conducting segmentation and uncertainty estimation. The trustworthy results could assist the clinicians to make a reliable diagnosis. Specifically, Dempster–Shafer Evidence Theory (DST) is introduced to parameterize the segmentation and uncertainty with evidence following Dirichlet distribution. The reliability of segmentation results among multi-phase CECT images is quantified explicitly. Meanwhile, a multi-expert mixture scheme (MEMS) is proposed to fuse the multi-phase evidences, which can guarantee the effect of fusion procedure based on theoretical analysis. Experimental results demonstrate the superiority of TMPLiTS compared with the state-of-the-art methods. Meanwhile, the robustness of TMPLiTS is verified, where the reliable performance can be guaranteed against the perturbations.
更多查看译文
关键词
Evidential neural network,Uncertainty estimation,Multi-phase computed tomography,Liver tumor segmentation,Trustworthy assessment
AI 理解论文
溯源树
样例
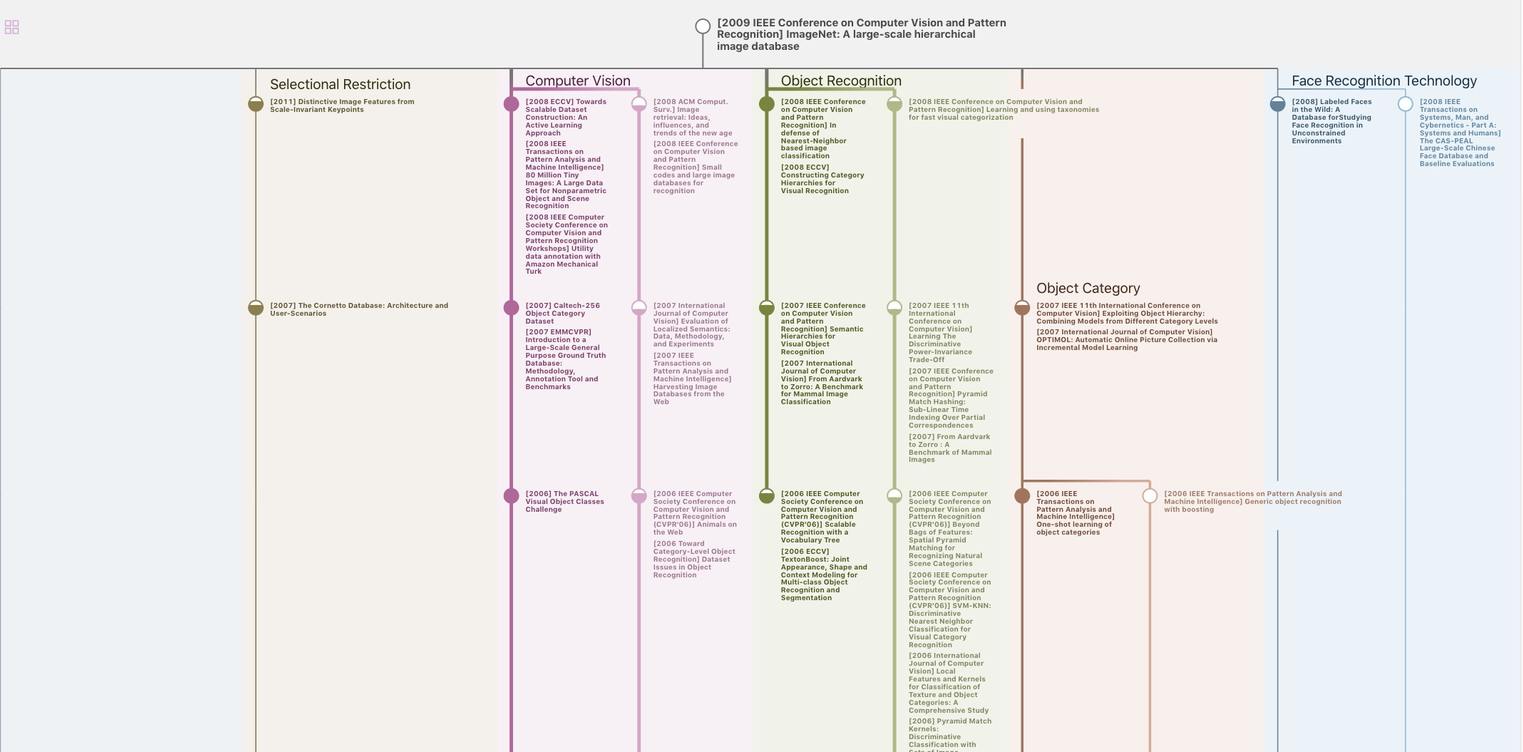
生成溯源树,研究论文发展脉络
Chat Paper
正在生成论文摘要