Sparse Sliced Inverse Regression via Random Projection
arxiv(2023)
摘要
We propose a novel sparse sliced inverse regression method based on random projections in a large $p$ small $n$ setting. Embedded in a generalized eigenvalue framework, the proposed approach finally reduces to parallel execution of low-dimensional (generalized) eigenvalue decompositions, which facilitates high computational efficiency. Theoretically, we prove that this method achieves the minimax optimal rate of convergence under suitable assumptions. Furthermore, our algorithm involves a delicate reweighting scheme, which can significantly enhance the identifiability of the active set of covariates. Extensive numerical studies demonstrate high superiority of the proposed algorithm in comparison to competing methods.
更多查看译文
AI 理解论文
溯源树
样例
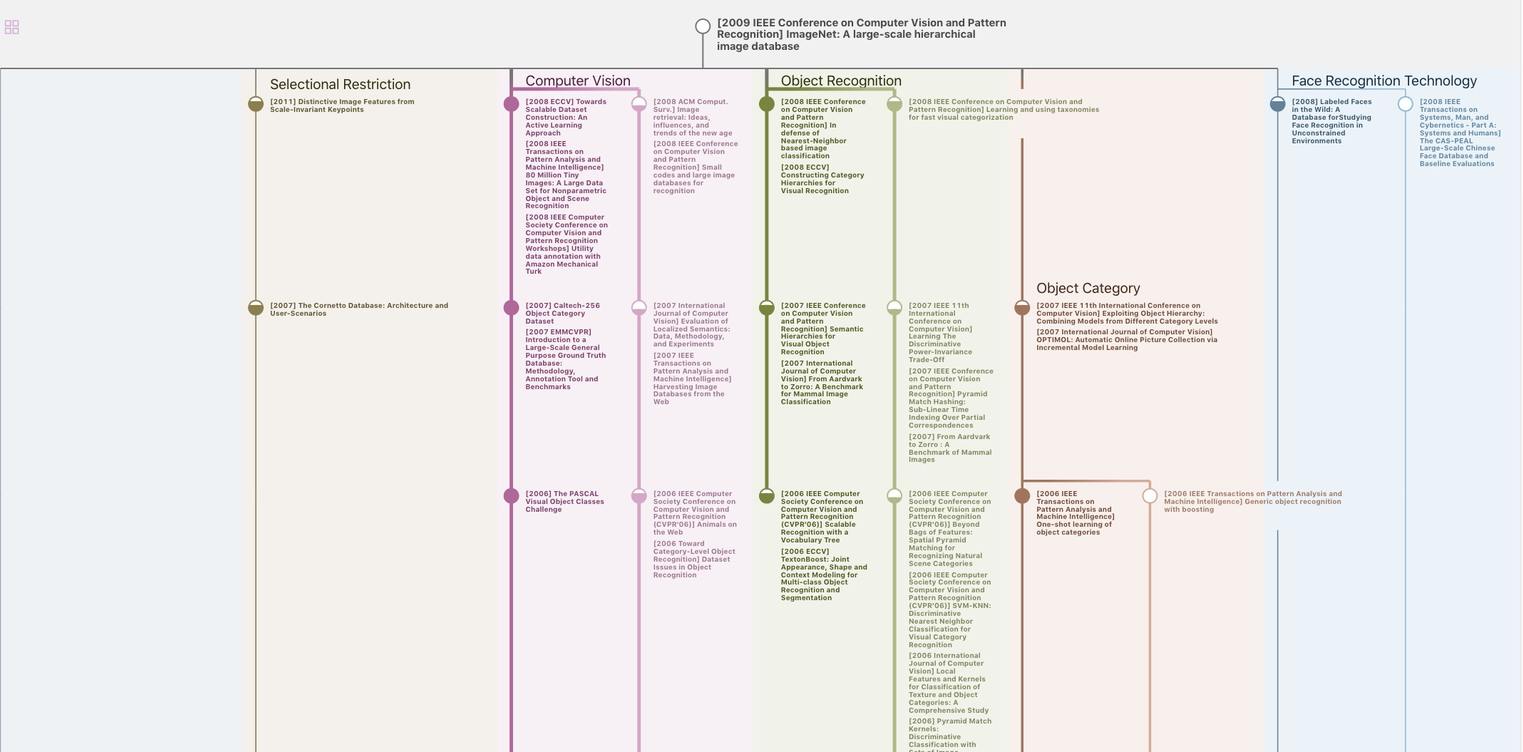
生成溯源树,研究论文发展脉络
Chat Paper
正在生成论文摘要