When a CBR in Hand is Better than Twins in the Bush
CoRR(2023)
摘要
AI methods referred to as interpretable are often discredited as inaccurate by supporters of the existence of a trade-off between interpretability and accuracy. In many problem contexts however this trade-off does not hold. This paper discusses a regression problem context to predict flight take-off delays where the most accurate data regression model was trained via the XGBoost implementation of gradient boosted decision trees. While building an XGB-CBR Twin and converting the XGBoost feature importance into global weights in the CBR model, the resultant CBR model alone provides the most accurate local prediction, maintains the global importance to provide a global explanation of the model, and offers the most interpretable representation for local explanations. This resultant CBR model becomes a benchmark of accuracy and interpretability for this problem context, and hence it is used to evaluate the two additive feature attribute methods SHAP and LIME to explain the XGBoost regression model. The results with respect to local accuracy and feature attribution lead to potentially valuable future work.
更多查看译文
关键词
cbr,twins
AI 理解论文
溯源树
样例
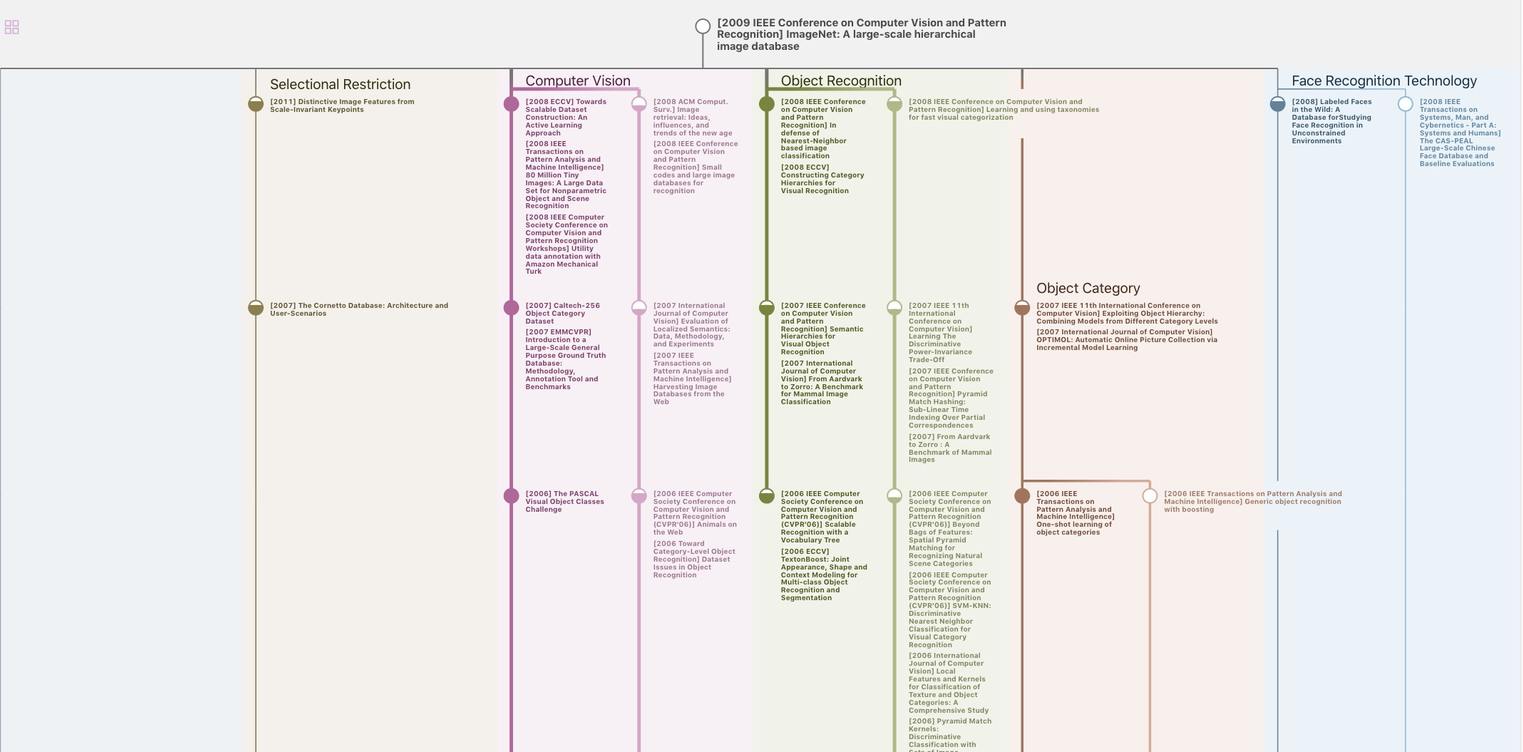
生成溯源树,研究论文发展脉络
Chat Paper
正在生成论文摘要