Scalable Optimal Transport Methods in Machine Learning: A Contemporary Survey.
IEEE transactions on pattern analysis and machine intelligence(2024)
摘要
Optimal Transport (OT) is a mathematical framework that first emerged in the eighteenth century and has led to a plethora of methods for answering many theoretical and applied questions. The last decade has been a witness to the remarkable contributions of this classical optimization problem to machine learning. This paper is about where and how optimal transport is used in machine learning with a focus on the question of scalable optimal transport. We provide a comprehensive survey of optimal transport while ensuring an accessible presentation as permitted by the nature of the topic and the context. First, we explain the optimal transport background and introduce different flavors (i.e. mathematical formulations), properties, and notable applications. We then address the fundamental question of how to scale optimal transport to cope with the current demands of big and high dimensional data. We conduct a systematic analysis of the methods used in the literature for scaling OT and present the findings in a unified taxonomy. We conclude with presenting some open challenges and discussing potential future research directions. A live repository of related OT research papers is maintained in https://github.com/abdelwahed/OT_for_big_data.git.
更多查看译文
关键词
earth mover's distance,optimal transport,Sinkhorn,domain adaptation,WGAN,sliced Wasserstein,generative models
AI 理解论文
溯源树
样例
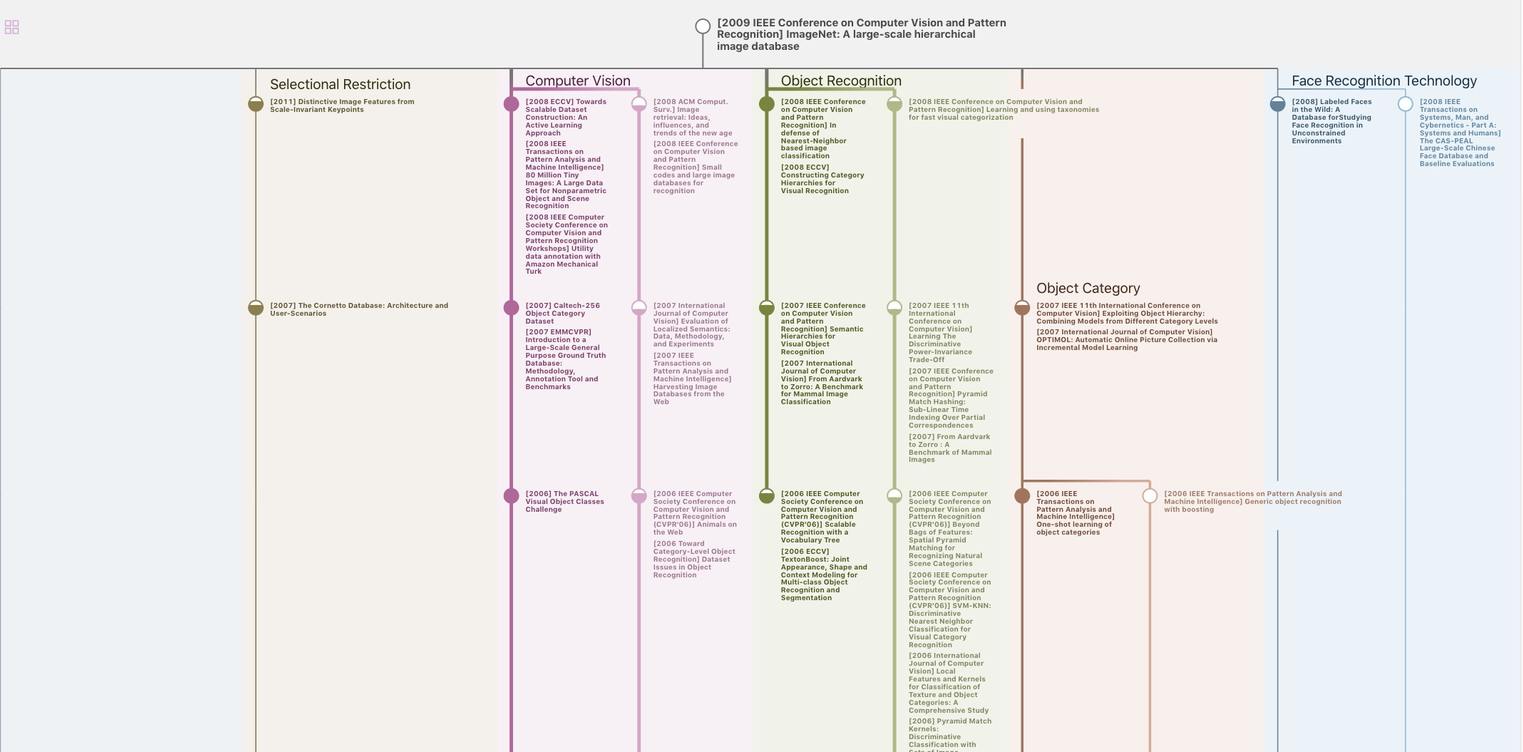
生成溯源树,研究论文发展脉络
Chat Paper
正在生成论文摘要