AMM: An Adaptive Online Map Matching Algorithm
IEEE Transactions on Intelligent Transportation Systems(2023)
摘要
Online map matching is essential for some location-based services, such as car navigation. However, due to GPS measurement errors and/or the lack of sufficient information, the performance of most existing online algorithms will degrade in the increasingly complex traffic environment. In this paper, we propose an adaptive online map matching algorithm called AMM. The basic idea is that AMM should be able to calibrate GPS observation data for various measurement errors and under complex urban conditions. First, we establish a collaborative evaluation model between GPS points and candidate points to effectively filter low-quality GPS measurement points, dynamically set the weights of different features and comprehensively select the best candidate points. Second, we propose a retrospective correction mechanism to correct the previous matching results when more information is available, which will help improve the accuracy of future GPS points. Furthermore, we define parameter self-tuning rules for AMM to enhance its portability by avoiding time-consuming parameter tuning steps. We conduct extensive experiments to evaluate the performance of AMM on real vehicle trajectory datasets. The experiment results show that AMM outperforms its counterparts by up to 32% in terms of accuracy and its performance in different traffic conditions is more stable.
更多查看译文
关键词
Map matching,GPS,traffic,adaptive
AI 理解论文
溯源树
样例
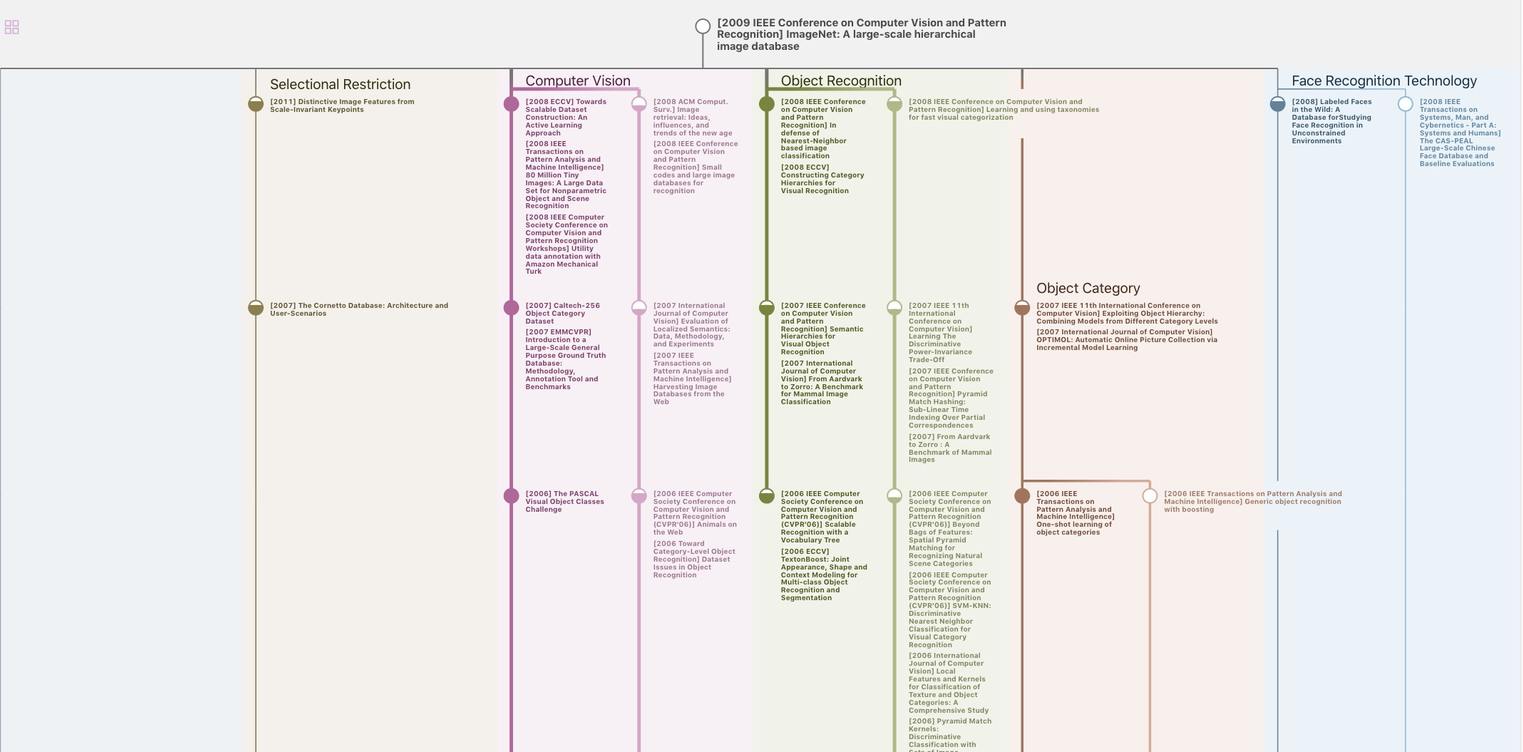
生成溯源树,研究论文发展脉络
Chat Paper
正在生成论文摘要