Ultrasound image-based deep features and radiomics for the discrimination of small fat-poor angiomyolipoma and small renal cell carcinoma
ULTRASOUND IN MEDICINE AND BIOLOGY(2023)
摘要
We evaluated the performance of ultrasound image -based deep features and radiomics for differentiating small fat-poor angiomyolipoma (sfp-AML) from small renal cell carcinoma (SRCC). This retrospective study included 194 patients with pathologically proven small renal masses (diameter <4 cm; 67 in the sfp-AML group and 127 in the SRCC group). We obtained 206 and 364 images from the sfp-AML and SRCC groups with experienced radiologist identification, respectively. We extracted 4024 deep features from the autoencoder neural network and 1497 radiomics features from the Pyradiomics toolbox; the latter included first-order, shape, high order, Laplacian of Gaussian and Wavelet features. All subjects were allocated to the training and testing sets with a ratio of 3:1 using stratified sampling. The least absolute shrinkage and selection operator (LASSO) regression model was applied to select the most diagnostic features. Support vector machine (SVM) was adopted as the discriminative classifier. An optimal feature subset including 45 deep and 7 radiomics features was screened by the LASSO model. The SVM classifier achieved good performance in discriminating between sfp-AMLs and SRCCs, with areas under the curve (AUCs) of 0.96 and 0.85 in the training and testing sets, respectively. The classifier built using deep and radiomics features can accurately differentiate sfp-AMLs from SRCCs on ultrasound imaging. (E-mail: shuminwang2014@163.com) (c) 2022 World Federation for Ultrasound in Medicine & Biology. All rights reserved.
更多查看译文
关键词
small renal cell carcinoma,ultrasound,radiomics,deep features,fat-poor
AI 理解论文
溯源树
样例
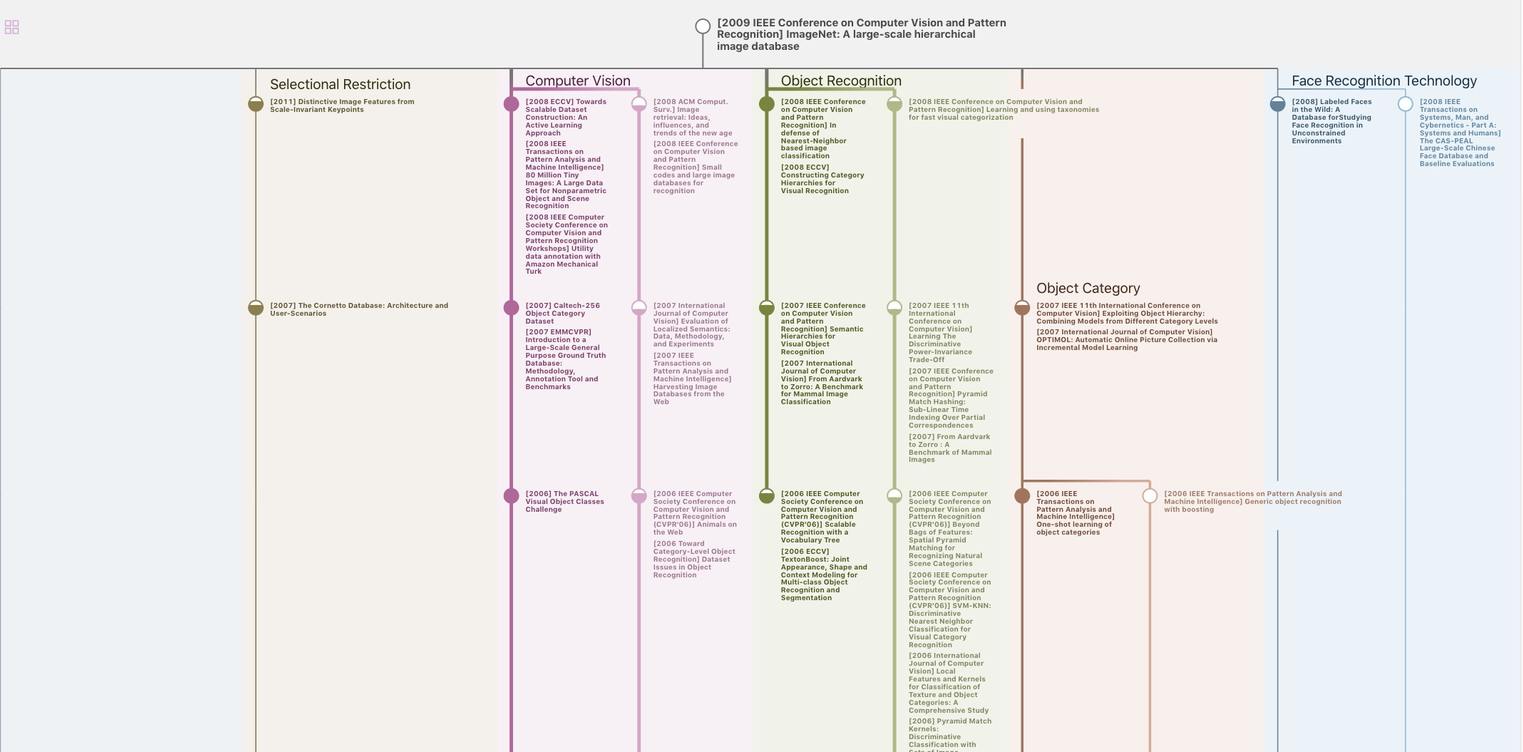
生成溯源树,研究论文发展脉络
Chat Paper
正在生成论文摘要