A general framework of online updating variable selection for generalized linear models with streaming datasets
JOURNAL OF STATISTICAL COMPUTATION AND SIMULATION(2023)
摘要
In the era of big data, one of the important issues is how to recover the sets of true features when the data sets arrive sequentially. The paper presents a general framework for online updating variable selection and parameter estimation in generalized linear models with streaming datasets. This is a type of online updating penalized likelihoods with differentiable or non-differentiable penalty functions. An online updating coordinate descent algorithm is proposed for solving the online updating optimization problem. Moreover, a tuning parameter selection is suggested in an online updating way. The selection and estimation consistencies and the oracle property are established, theoretically. Our methods are further examined and illustrated by various numerical examples from both simulation experiments and a real data analysis.
更多查看译文
关键词
Streaming data environment, penalized likelihoods, online updating criterion, oracle property
AI 理解论文
溯源树
样例
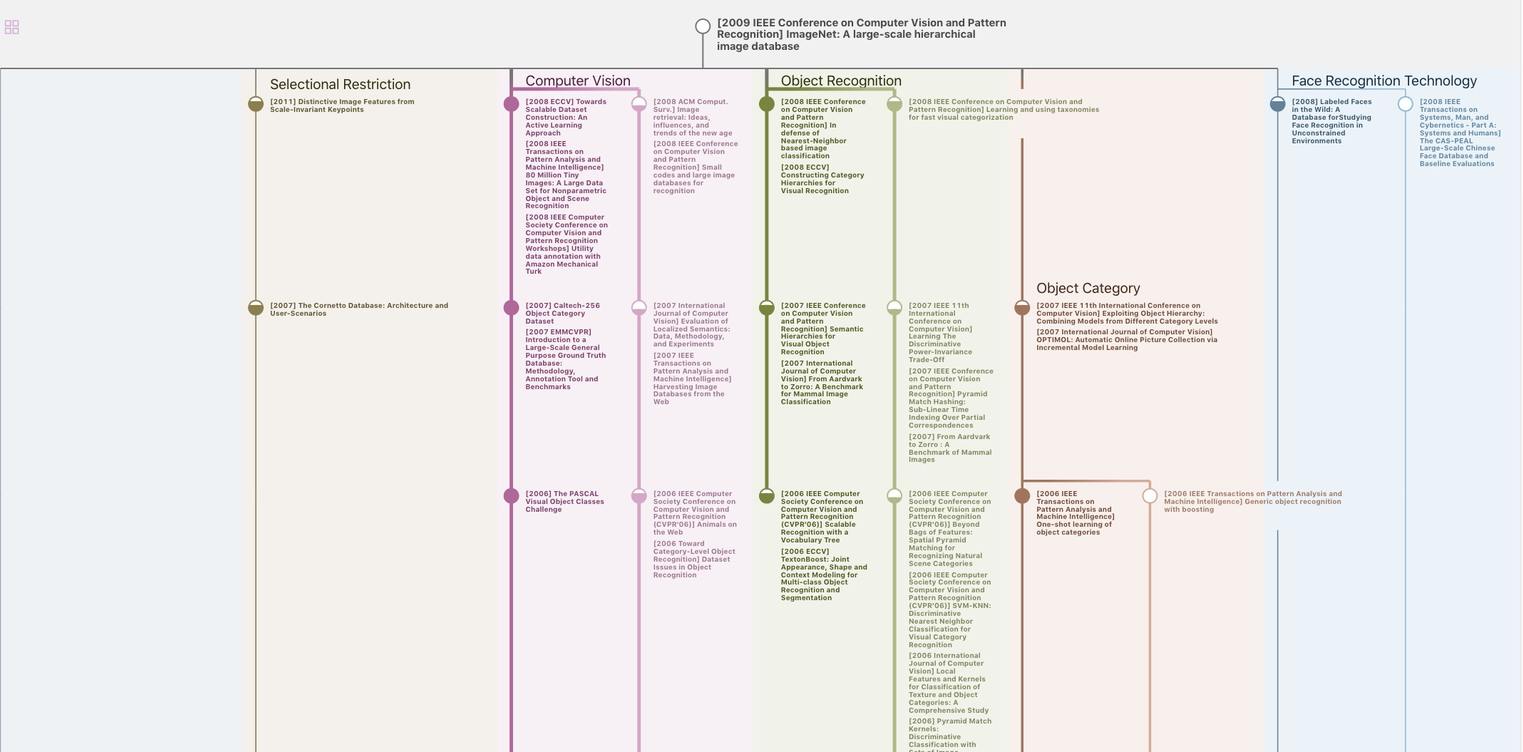
生成溯源树,研究论文发展脉络
Chat Paper
正在生成论文摘要