Augmented Large Language Models with Parametric Knowledge Guiding
CoRR(2023)
摘要
Large Language Models (LLMs) have significantly advanced natural language processing (NLP) with their impressive language understanding and generation capabilities. However, their performance may be suboptimal for long-tail or domain-specific tasks due to limited exposure to domain-specific knowledge and vocabulary. Additionally, the lack of transparency of most state-of-the-art (SOTA) LLMs, which can only be accessed via APIs, impedes further fine-tuning with custom data. Moreover, data privacy is a significant concern. To address these challenges, we propose the novel Parametric Knowledge Guiding (PKG) framework, which equips LLMs with a knowledge-guiding module to access relevant knowledge at runtime without altering the LLMs' parameters. Our PKG is based on open-source "white-box" small language models, allowing offline storage of any knowledge that LLMs require. We demonstrate that our PKG framework can enhance the performance of "black-box" LLMs on a range of long-tail and domain-specific downstream tasks requiring factual, tabular, medical, and multimodal knowledge.
更多查看译文
AI 理解论文
溯源树
样例
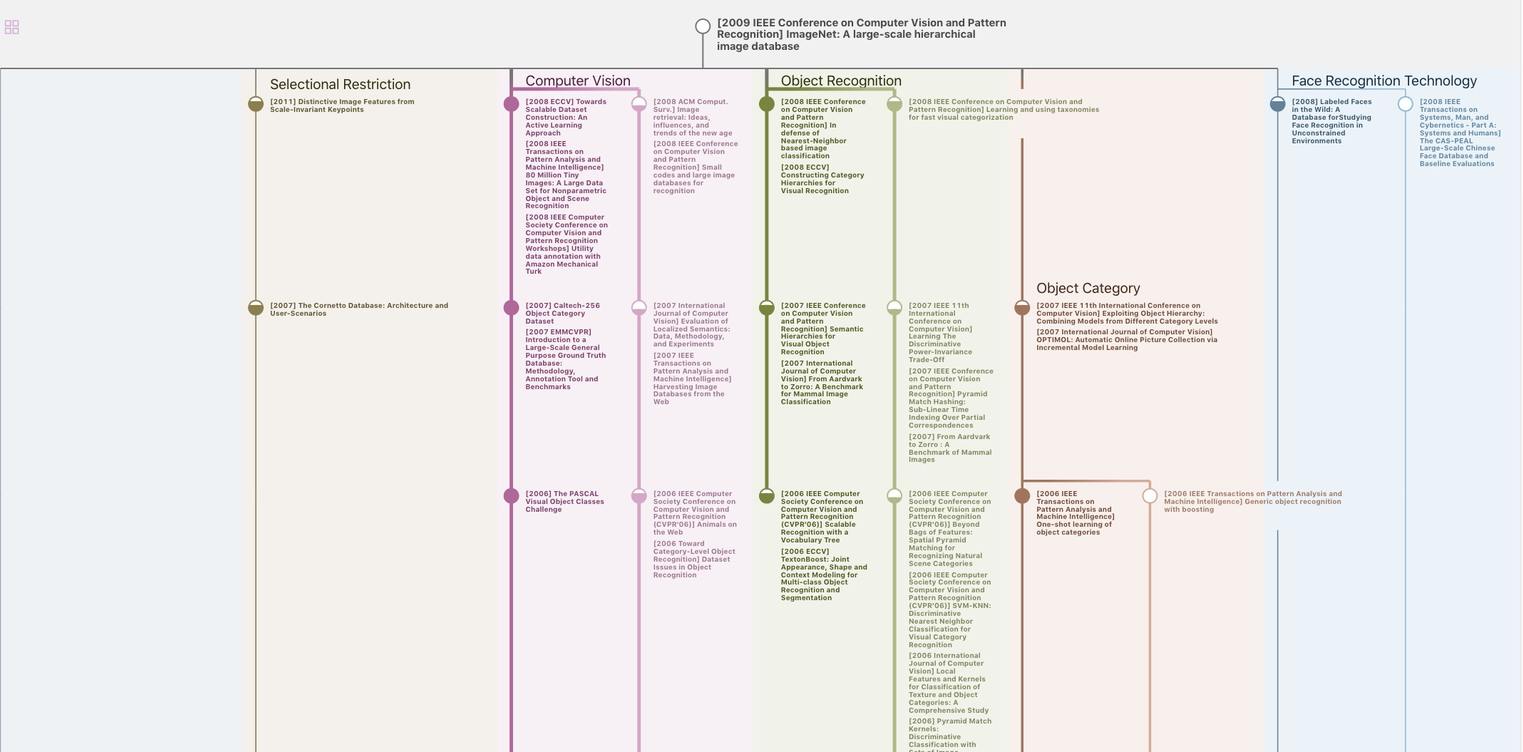
生成溯源树,研究论文发展脉络
Chat Paper
正在生成论文摘要