Target-Driven One-Shot Unsupervised Domain Adaptation
IMAGE ANALYSIS AND PROCESSING, ICIAP 2023, PT I(2023)
摘要
In this paper, we introduce a novel framework for the challenging problem of One-Shot Unsupervised Domain Adaptation (OS-UDA), which aims to adapt to a target domain with only a single unlabeled target sample. Unlike existing approaches that rely on large labeled source and unlabeled target data, our Target-Driven One-Shot UDA (TOS-UDA) approach employs a learnable augmentation strategy guided by the target sample's style to align the source distribution with the target distribution. Our method consists of three modules: an augmentation module, a style alignment module, and a classifier. Unlike existing methods, our augmentation module allows for strong transformations of the source samples, and the style of the single target sample available is exploited to guide the augmentation by ensuring perceptual similarity. Furthermore, our approach integrates augmentation with style alignment, eliminating the need for separate pre-training on additional datasets. Our method outperforms or performs comparably to existing OS-UDA methods on the Digits and DomainNet benchmarks.
更多查看译文
关键词
Unsupervised domain adaptation,Data augmentation,One-Shot
AI 理解论文
溯源树
样例
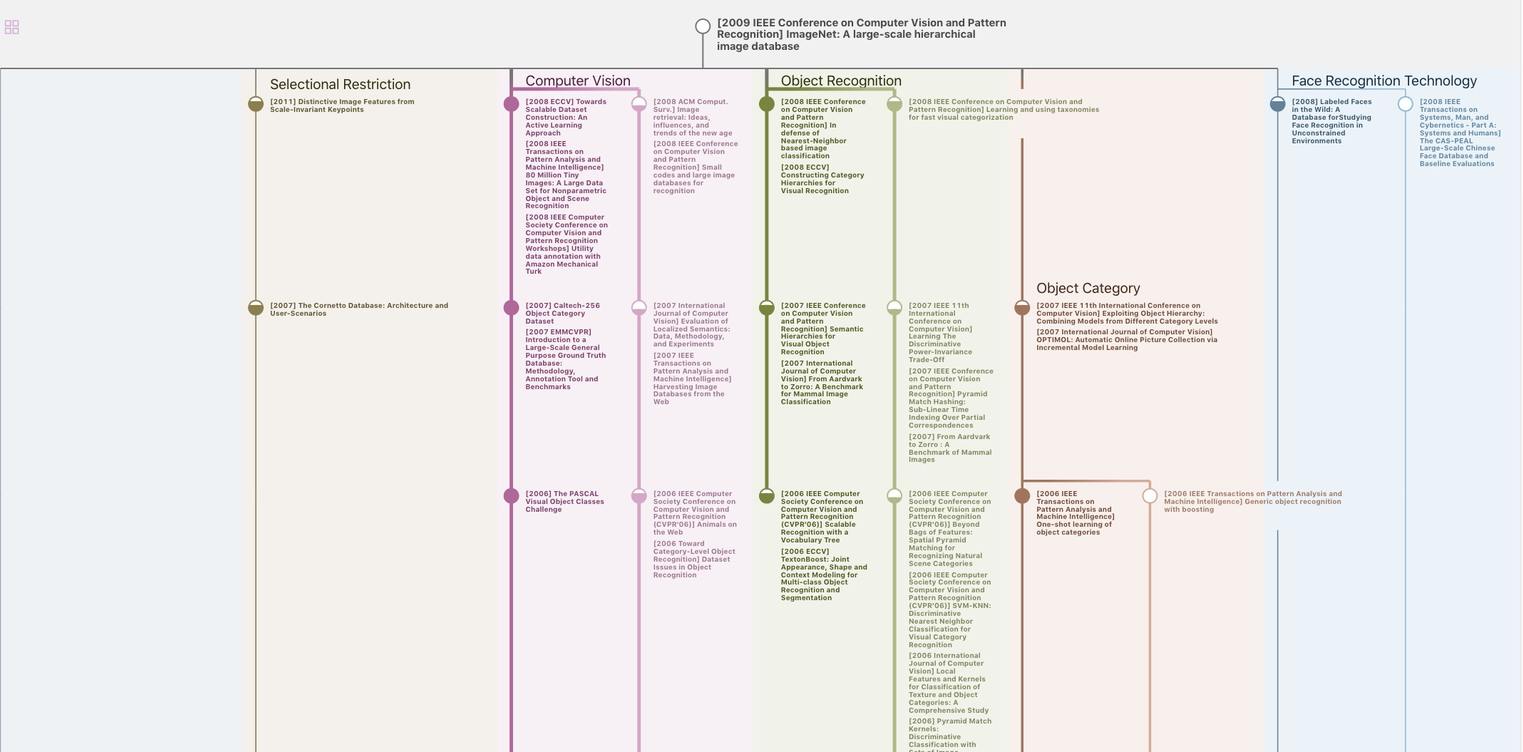
生成溯源树,研究论文发展脉络
Chat Paper
正在生成论文摘要