Can Diffusion Model Achieve Better Performance in Text Generation? Bridging the Gap between Training and Inference!
conf_acl(2023)
摘要
Diffusion models have been successfully adapted to text generation tasks by mapping the discrete text into the continuous space. However, there exist nonnegligible gaps between training and inference, owing to the absence of the forward process during inference. Thus, the model only predicts based on the previously generated reverse noise rather than the noise computed by the forward process. Besides, the widely-used downsampling strategy in speeding up the inference will cause the mismatch of diffusion trajectories between training and inference. To understand and mitigate the above two types of training-inference discrepancies, we launch a thorough preliminary study. Based on our observations, we propose two simple yet effective methods to bridge the gaps mentioned above, named Distance Penalty and Adaptive Decay Sampling. Extensive experiments on \textbf{6} generation tasks confirm the superiority of our methods, which can achieve $100\times \rightarrow 200\times$ speedup with better performance.
更多查看译文
关键词
text generation
AI 理解论文
溯源树
样例
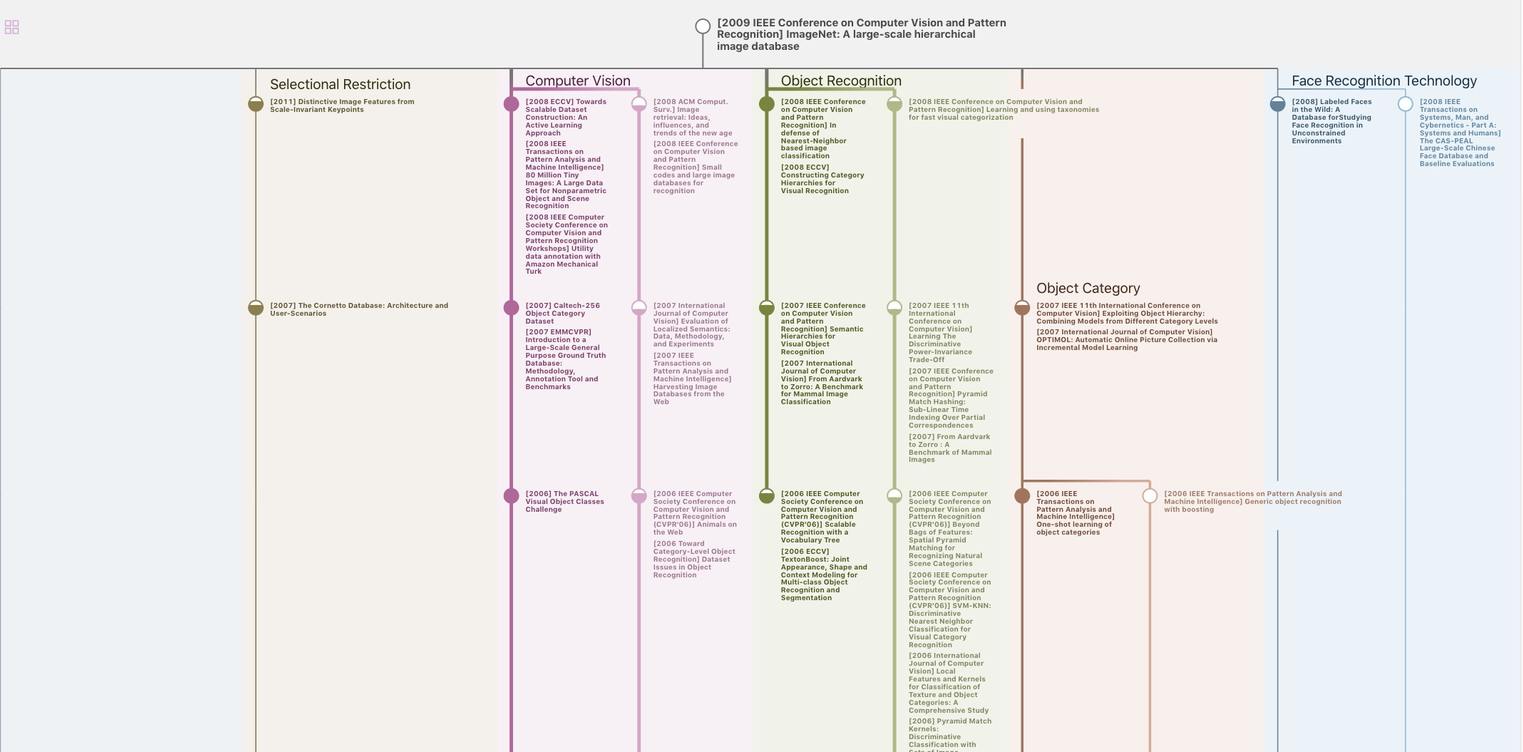
生成溯源树,研究论文发展脉络
Chat Paper
正在生成论文摘要