Wasserstein distance bounds on the normal approximation of empirical autocovariances and cross-covariances under non-stationarity and stationarity
JOURNAL OF TIME SERIES ANALYSIS(2023)
摘要
The autocovariance and cross-covariance functions naturally appear in many time series procedures (e.g. autoregression or prediction). Under assumptions, empirical versions of the autocovariance and cross-covariance are asymptotically normal with covariance structure depending on the second- and fourth-order spectra. Under non-restrictive assumptions, we derive a bound for the Wasserstein distance of the finite-sample distribution of the estimator of the autocovariance and cross-covariance to the Gaussian limit. An error of approximation to the second-order moments of the estimator and an m-dependent approximation are the key ingredients to obtain the bound. As a worked example, we discuss how to compute the bound for causal autoregressive processes of order 1 with different distributions for the innovations. To assess our result, we compare our bound to Wasserstein distances obtained via simulation.
更多查看译文
关键词
Autocovariance,time series,Wasserstein distance,Stein's method
AI 理解论文
溯源树
样例
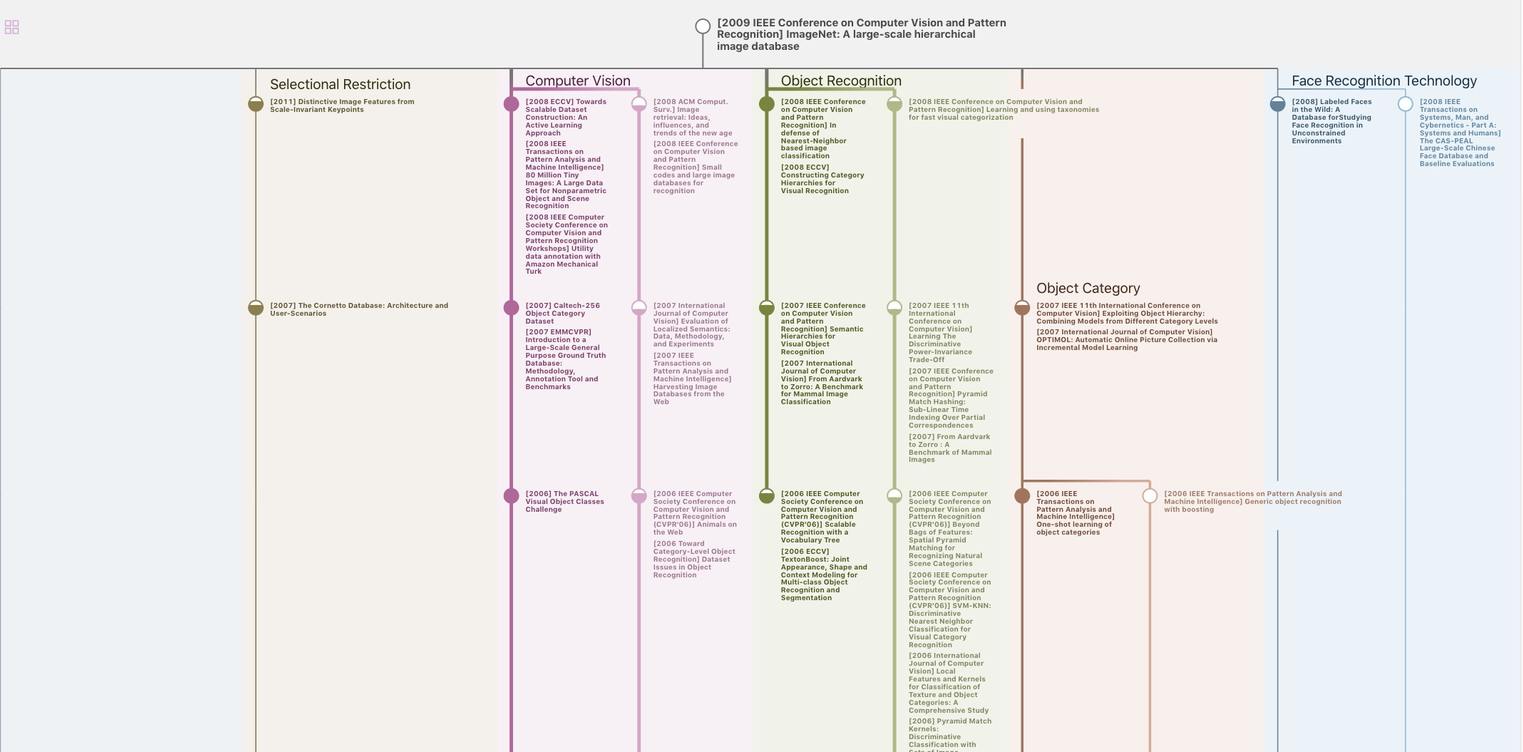
生成溯源树,研究论文发展脉络
Chat Paper
正在生成论文摘要