A Computational Model of Children's Learning and Use of Probabilities Across Different Ages
arXiv (Cornell University)(2023)
摘要
Recent empirical work has shown that human children are adept at learning and reasoning with probabilities. Here, we model a recent experiment investigating the development of school-age children's non-symbolic probability reasoning ability using the Neural Probability Learner and Sampler (NPLS) system. We demonstrate that NPLS can accurately simulate children's probability judgments at different ages, tasks and difficulty levels to discriminate two probabilistic choices through accurate probability learning and sampling. An extension of NPLS using a skewed heuristic distribution can also model children's tendency to wrongly select the outcome with more favorable items but less likely to draw the favorable ones when the probabilistic choices are similar. We discuss the roles of two model parameters that can be adjusted to simulate the probability matching versus probability maximization phenomena in children, and why frequency biases children's probabilistic judgments.
更多查看译文
关键词
different ages,probabilities
AI 理解论文
溯源树
样例
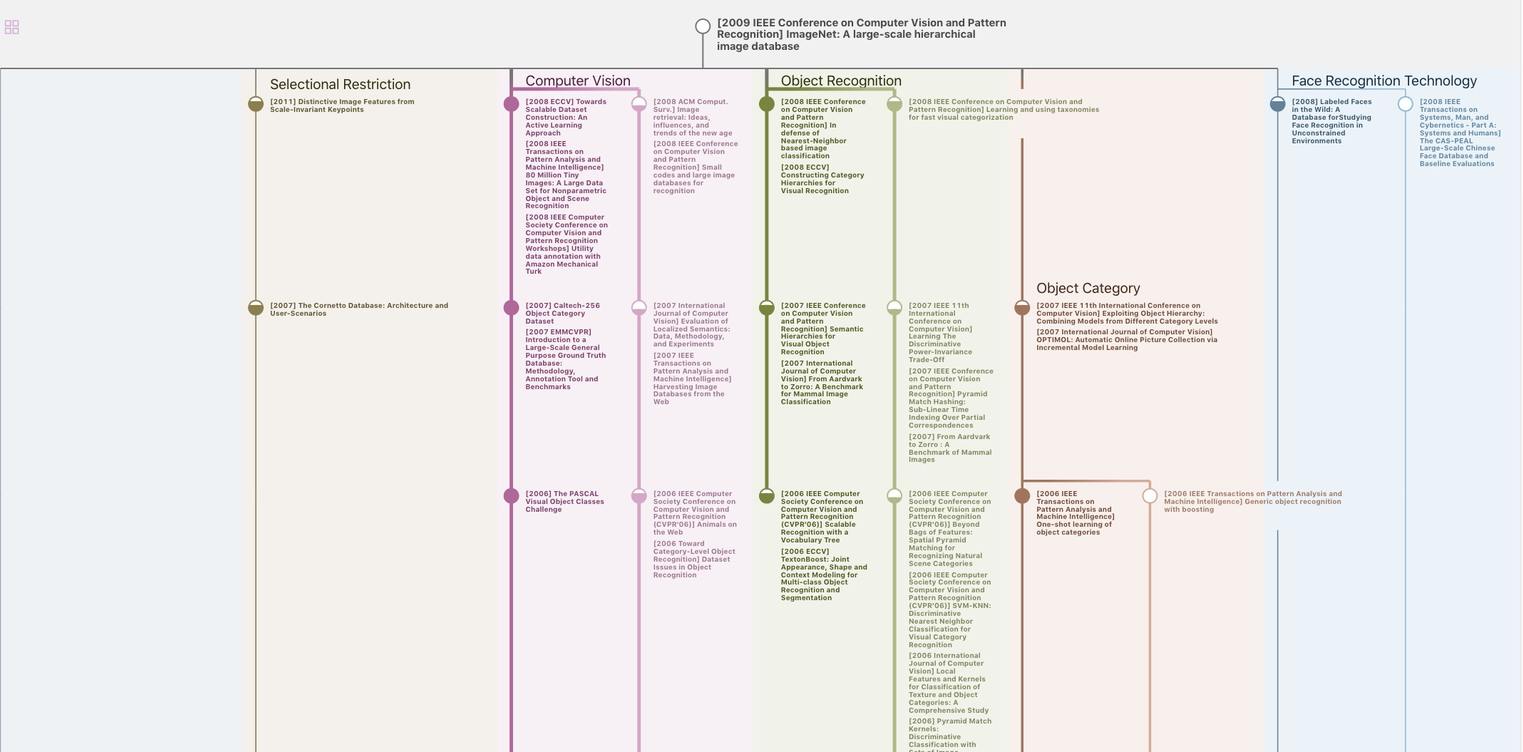
生成溯源树,研究论文发展脉络
Chat Paper
正在生成论文摘要