Keyword-Based Diverse Image Retrieval by Semantics-aware Contrastive Learning and Transformer
PROCEEDINGS OF THE 46TH INTERNATIONAL ACM SIGIR CONFERENCE ON RESEARCH AND DEVELOPMENT IN INFORMATION RETRIEVAL, SIGIR 2023(2023)
摘要
In addition to relevance, diversity is an important yet less studied performance metric of cross-modal image retrieval systems, which is critical to user experience. Existing solutions for diversity-aware image retrieval either explicitly post-process the raw retrieval results from standard retrieval systems or try to learn multi-vector representations of images to represent their diverse semantics. However, neither of them is good enough to balance relevance and diversity. On the one hand, standard retrieval systems are usually biased to common semantics and seldom exploit diversity-aware regularization in training, which makes it difficult to promote diversity by post-processing. On the other hand, multi-vector representation methods are not guaranteed to learn robust multiple projections. As a result, irrelevant images and images of rare or unique semantics may be projected inappropriately, which degrades the relevance and diversity of the results generated by some typical algorithms like top-k To cope with these problems, this paper presents a new method called CoLT that tries to generate much more representative and robust representations for accurately classifying images. Specifically, CoLT first extracts semantics-aware image features by enhancing the preliminary representations of an existing one-toone cross-modal system with semantics-aware contrastive learning. Then, a transformer-based token classifier is developed to subsume all the features into their corresponding categories. Finally, a post-processing algorithm is designed to retrieve images from each category to form the final retrieval result. Extensive experiments on two real-world datasets Div400 and Div150Cred show that CoLT can effectively boost diversity, and outperforms the existing methods as a whole (with a higher F1 score).
更多查看译文
关键词
Cross-modal retrieval,Keyword-based image retrieval,Diversification retrieval,Transformer
AI 理解论文
溯源树
样例
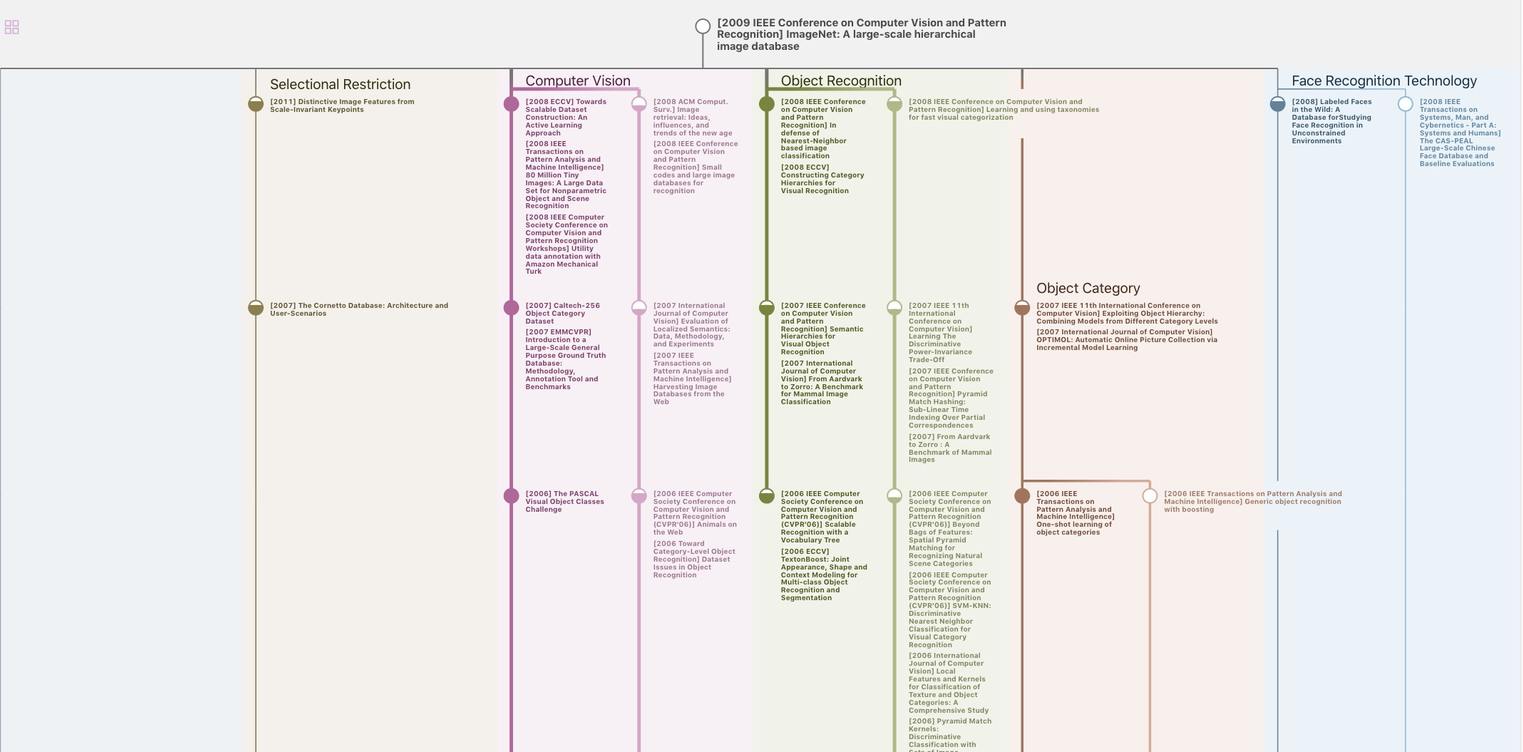
生成溯源树,研究论文发展脉络
Chat Paper
正在生成论文摘要