SST-ReversibleNet: Reversible-prior-based Spectral-Spatial Transformer for Efficient Hyperspectral Image Reconstruction
CoRR(2023)
摘要
Spectral image reconstruction is an important task in snapshot compressed imaging. This paper aims to propose a new end-to-end framework with iterative capabilities similar to a deep unfolding network to improve reconstruction accuracy, independent of optimization conditions, and to reduce the number of parameters. A novel framework called the reversible-prior-based method is proposed. Inspired by the reversibility of the optical path, the reversible-prior-based framework projects the reconstructions back into the measurement space, and then the residuals between the projected data and the real measurements are fed into the network for iteration. The reconstruction subnet in the network then learns the mapping of the residuals to the true values to improve reconstruction accuracy. In addition, a novel spectral-spatial transformer is proposed to account for the global correlation of spectral data in both spatial and spectral dimensions while balancing network depth and computational complexity, in response to the shortcomings of existing transformer-based denoising modules that ignore spatial texture features or learn local spatial features at the expense of global spatial features. Extensive experiments show that our SST-ReversibleNet significantly outperforms state-of-the-art methods on simulated and real HSI datasets, while requiring lower computational and storage costs. https://github.com/caizeyu1992/SST
更多查看译文
关键词
efficient hyperspectral image reconstruction,sst-reversiblenet,reversible-prior-based,spectral-spatial
AI 理解论文
溯源树
样例
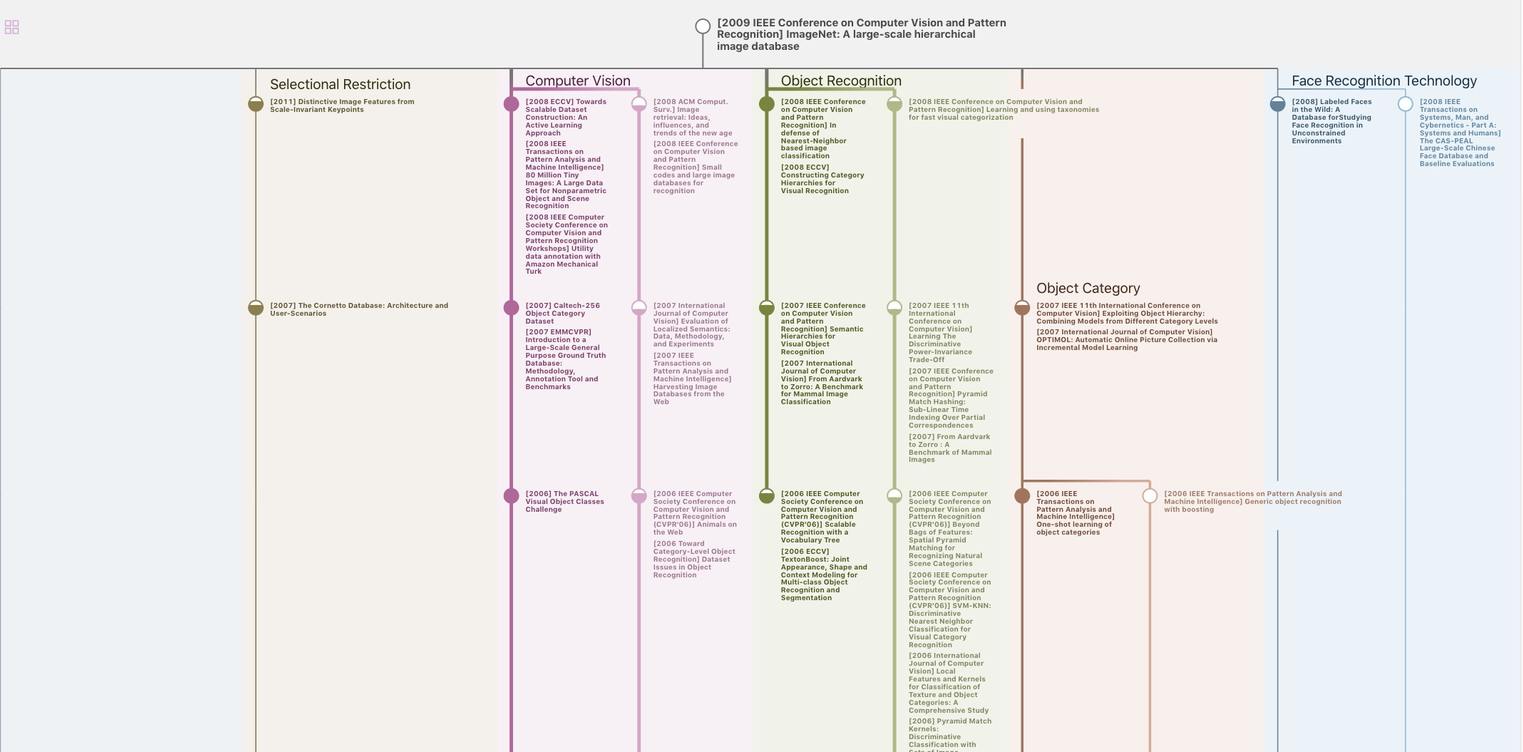
生成溯源树,研究论文发展脉络
Chat Paper
正在生成论文摘要