Data-driven classification of sheared stratified turbulence from experimental shadowgraphs
PHYSICAL REVIEW FLUIDS(2024)
摘要
We propose a dimensionality reduction and unsupervised clustering method for the automatic classification and reduced-order modeling of density-stratified turbulence in laboratory experiments. We apply this method to 113 long shadowgraph movies collected in a "stratified inclined duct" experiment, where turbulence is generated by instabilities arising from a sheared buoyancy-driven counterflow at Reynolds numbers Re approximate to 300-5000, tilt angles theta=1(degrees)-6(degrees), and Prandtl number Pr approximate to 700. The method automatically detects edges representative of discrete density interfaces, extracts a low-dimensional vector of statistics representative of their morphology, projects these statistics onto a two-dimensional phase space of principal coordinates, and applies a clustering algorithm. Five clusters are detected and interpreted physically based on their typical interface morphology and an examination of representative frames, revealing distinct types of turbulence and mixing: laminarizing, braided, overturning, granular, and unstructured, as well as some intermediate types. The ratio of time spent in each cluster varies gradually across the (Re,theta) space. At intermediate values of Re theta, intermittent turbulence cycles between clusters in phase space and reveals at least two distinct routes to stratified turbulence. These insights demonstrate the potential of this method to reveal the underlying physics of complex turbulent systems from large experimental datasets.
更多查看译文
关键词
stratified turbulence,classification,data-driven
AI 理解论文
溯源树
样例
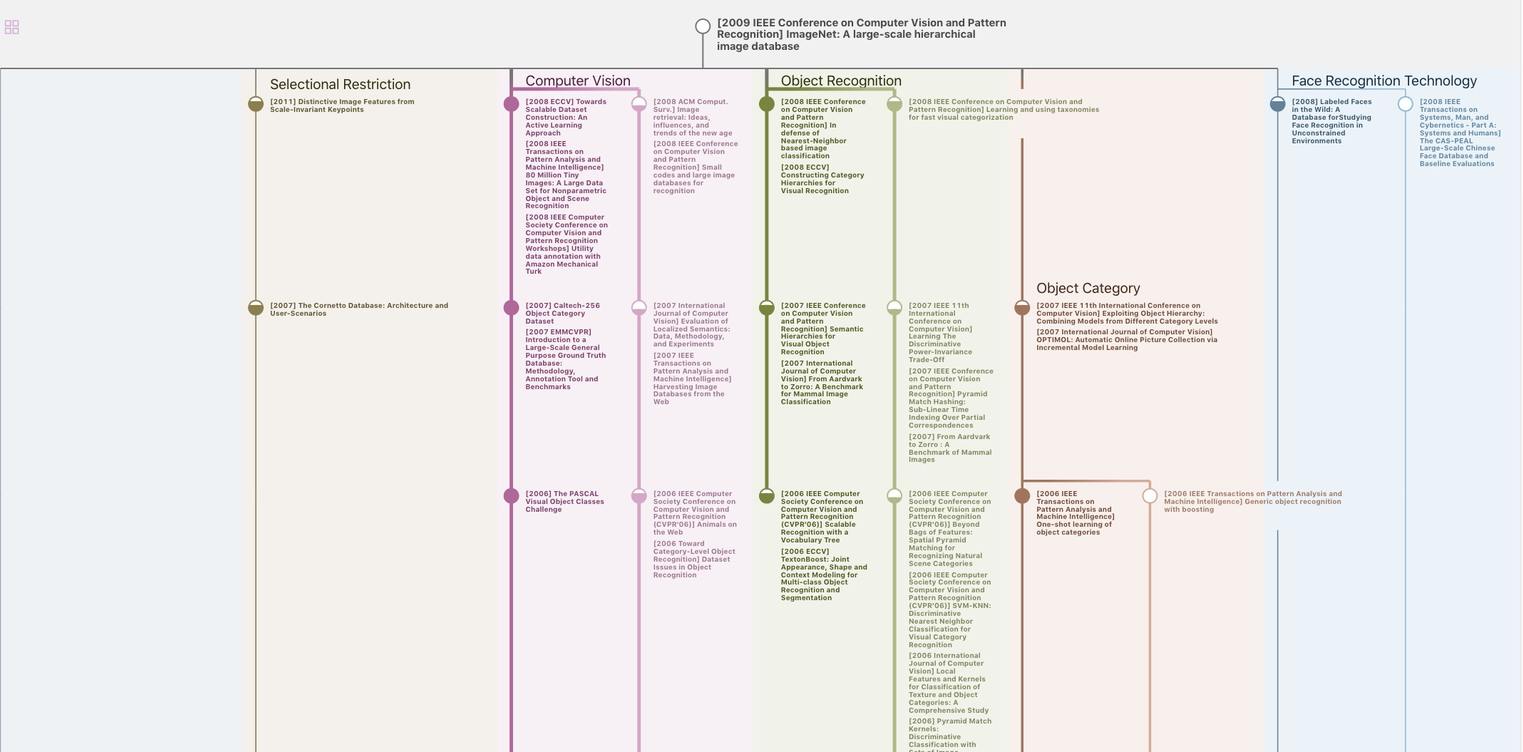
生成溯源树,研究论文发展脉络
Chat Paper
正在生成论文摘要