Learning Sentinel-2 reflectance dynamics for data-driven assimilation and forecasting
CoRR(2023)
摘要
Over the last few years, massive amounts of satellite multispectral and hyperspectral images covering the Earth's surface have been made publicly available for scientific purpose, for example through the European Copernicus project. Simultaneously, the development of self-supervised learning (SSL) methods has sparked great interest in the remote sensing community, enabling to learn latent representations from unlabeled data to help treating downstream tasks for which there is few annotated examples, such as interpolation, forecasting or unmixing. Following this line, we train a deep learning model inspired from the Koopman operator theory to model long-term reflectance dynamics in an unsupervised way. We show that this trained model, being differentiable, can be used as a prior for data assimilation in a straightforward way. Our datasets, which are composed of Sentinel-2 multispectral image time series, are publicly released with several levels of treatment.
更多查看译文
关键词
assimilation
AI 理解论文
溯源树
样例
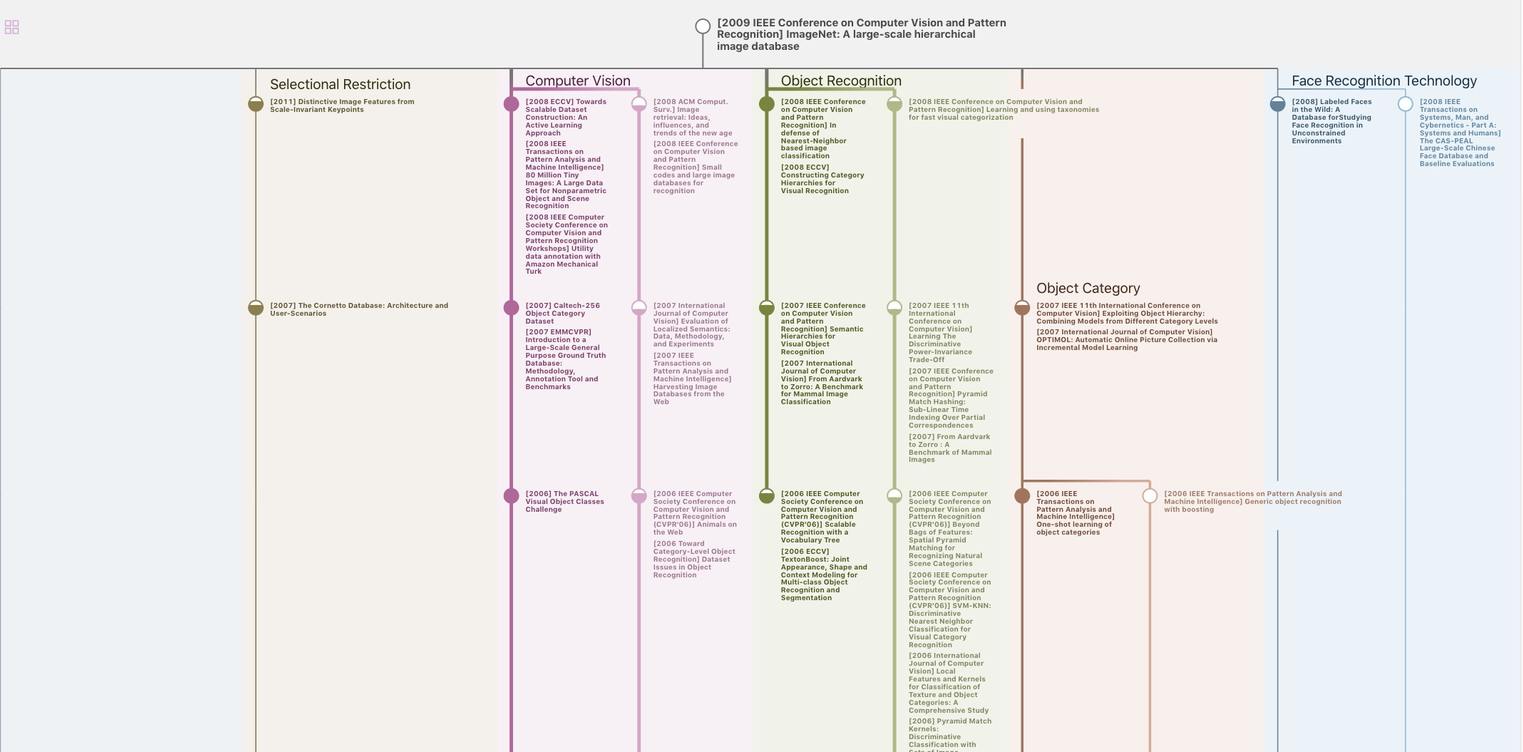
生成溯源树,研究论文发展脉络
Chat Paper
正在生成论文摘要