Is dataset condensation a silver bullet for healthcare data sharing?
CoRR(2023)
摘要
Safeguarding personal information is paramount for healthcare data sharing, a challenging issue without any silver bullet thus far. We study the prospect of a recent deep-learning advent, dataset condensation (DC), in sharing healthcare data for AI research, and the results are promising. The condensed data abstracts original records and irreversibly conceals individual-level knowledge to achieve a bona fide de-identification, which permits free sharing. Moreover, the original deep-learning utilities are well preserved in the condensed data with compressed volume and accelerated model convergences. In PhysioNet-2012, a condensed dataset of 20 samples can orient deep models attaining 80.3% test AUC of mortality prediction (versus 85.8% of 5120 original records), an inspiring discovery generalised to MIMIC-III and Coswara datasets. We also interpret the inhere privacy protections of DC through theoretical analysis and empirical evidence. Dataset condensation opens a new gate to sharing healthcare data for AI research with multiple desirable traits.
更多查看译文
关键词
healthcare dataset sharing,dataset condensation
AI 理解论文
溯源树
样例
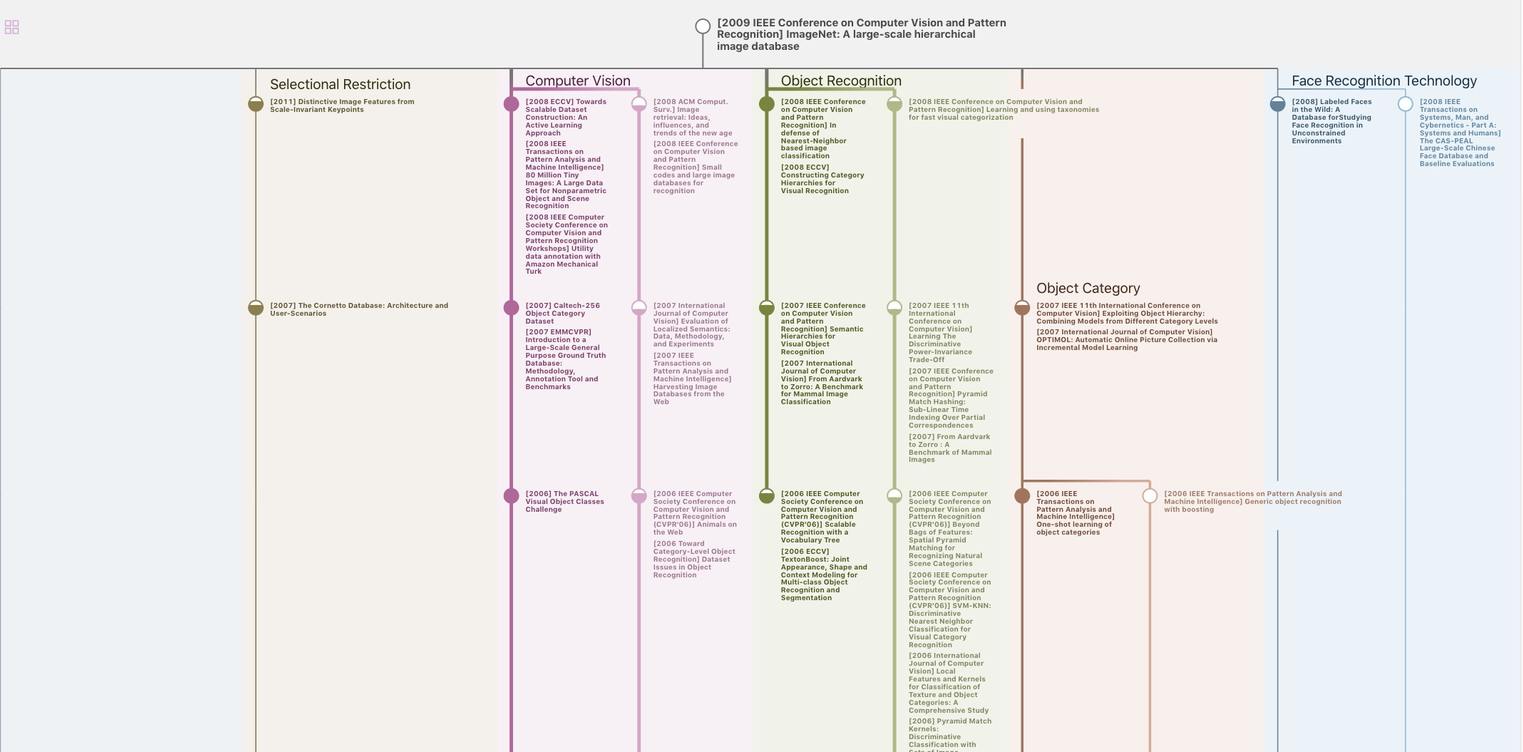
生成溯源树,研究论文发展脉络
Chat Paper
正在生成论文摘要