Verifiable Learning for Robust Tree Ensembles
PROCEEDINGS OF THE 2023 ACM SIGSAC CONFERENCE ON COMPUTER AND COMMUNICATIONS SECURITY, CCS 2023(2023)
摘要
Verifying the robustness of machine learning models against evasion attacks at test time is an important research problem. Unfortunately, prior work established that this problem is NP-hard for decision tree ensembles, hence bound to be intractable for specific inputs. In this paper, we identify a restricted class of decision tree ensembles, called large-spread ensembles, which admit a security verification algorithm running in polynomial time. We then propose a new approach called verifiable learning, which advocates the training of such restricted model classes which are amenable for efficient verification. We show the benefits of this idea by designing a new training algorithm that automatically learns a large-spread decision tree ensemble from labelled data, thus enabling its security verification in polynomial time. Experimental results on public datasets confirm that large-spread ensembles trained using our algorithm can be verified in a matter of seconds, using standard commercial hardware. Moreover, large-spread ensembles are more robust than traditional ensembles against evasion attacks, at the cost of an acceptable loss of accuracy in the non-adversarial setting.
更多查看译文
关键词
Machine Learning and Security,Robustness,Verification and Program Analysis for Machine Learning Models
AI 理解论文
溯源树
样例
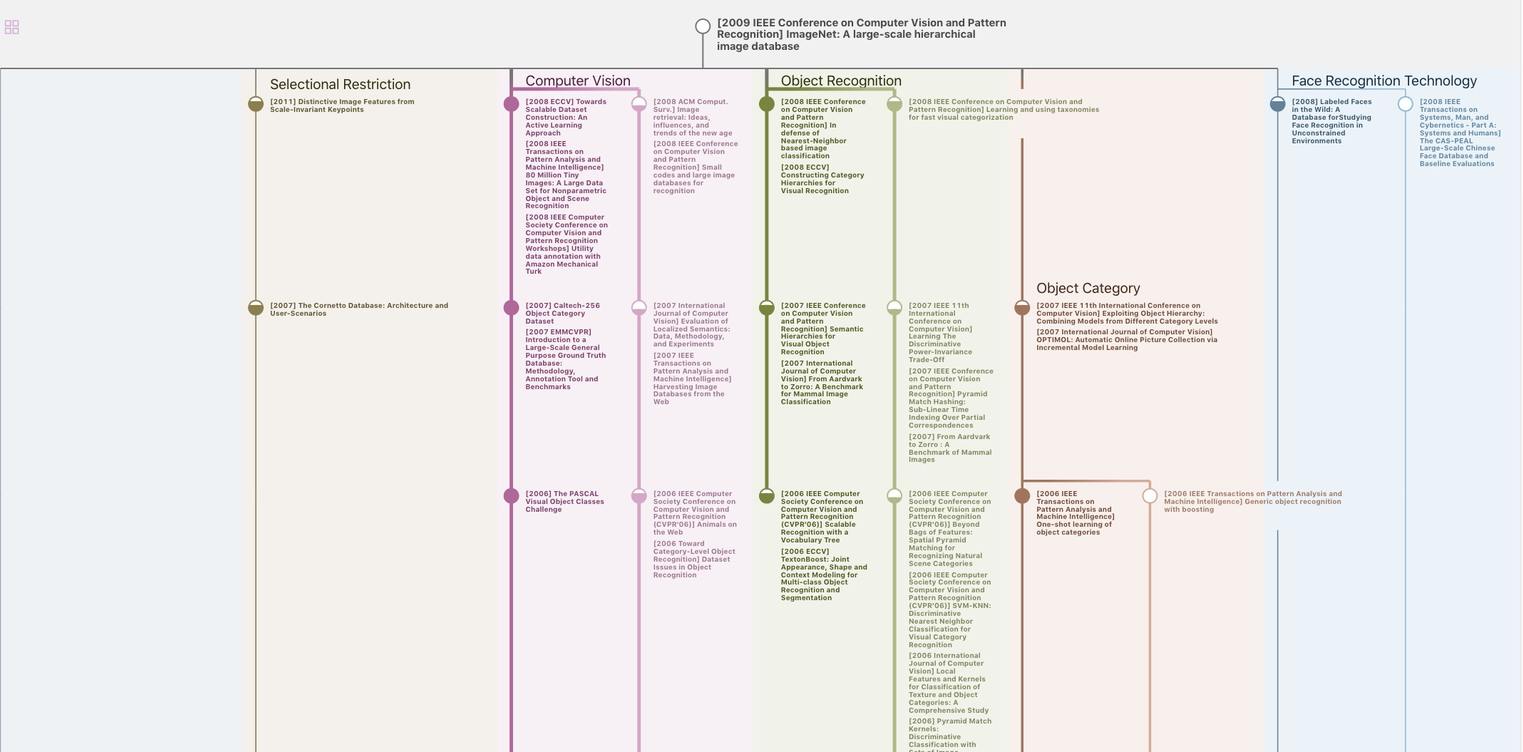
生成溯源树,研究论文发展脉络
Chat Paper
正在生成论文摘要