Few-shot Domain-Adaptive Visually-fused Event Detection from Text
CoRR(2023)
摘要
Incorporating auxiliary modalities such as images into event detection models has attracted increasing interest over the last few years. The complexity of natural language in describing situations has motivated researchers to leverage the related visual context to improve event detection performance. However, current approaches in this area suffer from data scarcity, where a large amount of labelled text-image pairs are required for model training. Furthermore, limited access to the visual context at inference time negatively impacts the performance of such models, which makes them practically ineffective in real-world scenarios. In this paper, we present a novel domain-adaptive visually-fused event detection approach that can be trained on a few labelled image-text paired data points. Specifically, we introduce a visual imaginator method that synthesises images from text in the absence of visual context. Moreover, the imaginator can be customised to a specific domain. In doing so, our model can leverage the capabilities of pre-trained vision-language models and can be trained in a few-shot setting. This also allows for effective inference where only single-modality data (i.e. text) is available. The experimental evaluation on the benchmark M2E2 dataset shows that our model outperforms existing state-of-the-art models, by up to 11 points.
更多查看译文
关键词
event detection,multimodal event detection,few-shot learning,image generation
AI 理解论文
溯源树
样例
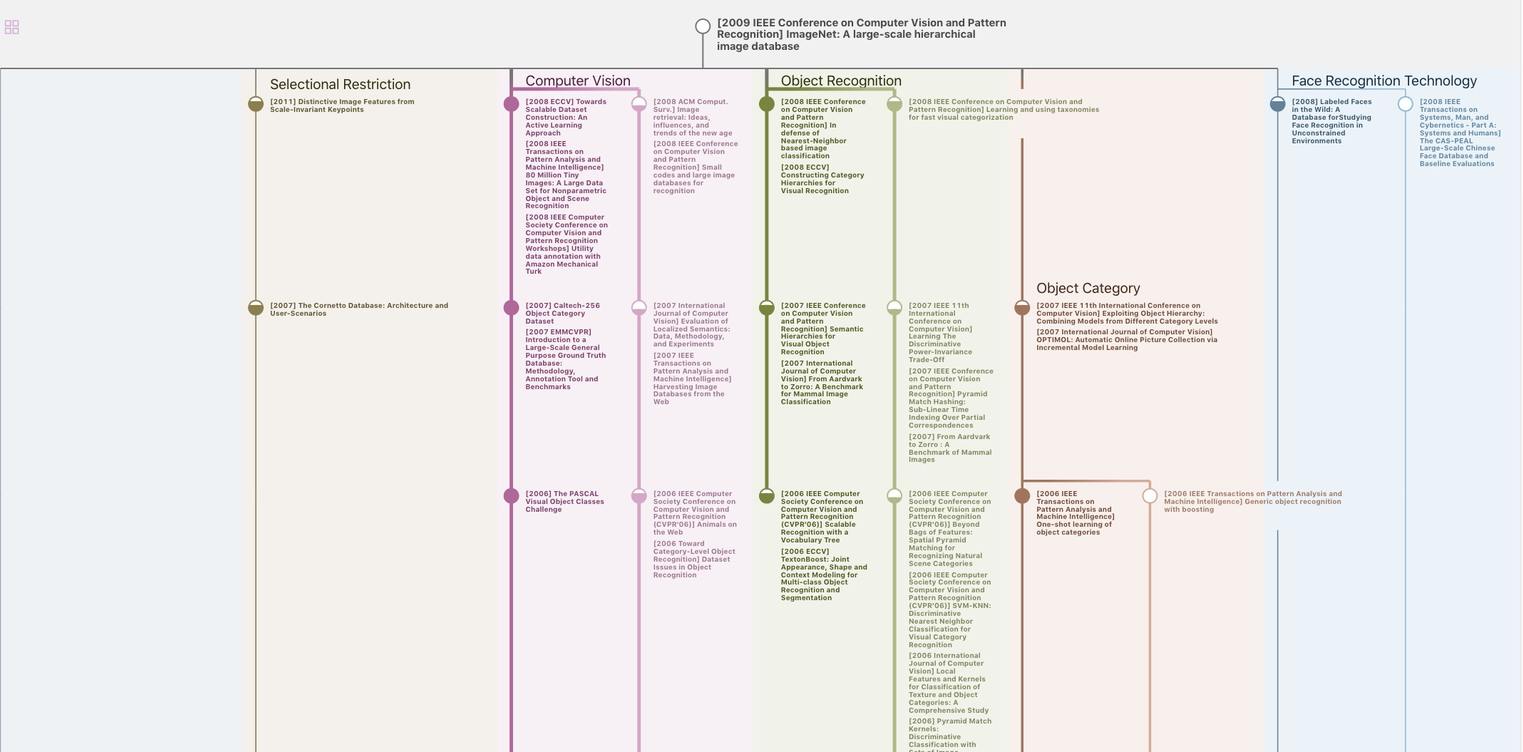
生成溯源树,研究论文发展脉络
Chat Paper
正在生成论文摘要