A multi-view contrastive learning for heterogeneous network embedding
Scientific Reports(2023)
摘要
Graph contrastive learning has been developed to learn discriminative node representations on homogeneous graphs. However, it is not clear how to augment the heterogeneous graphs without substantially altering the underlying semantics or how to design appropriate pretext tasks to fully capture the rich semantics preserved in heterogeneous information networks (HINs). Moreover, early investigations demonstrate that contrastive learning suffer from sampling bias, whereas conventional debiasing techniques (e.g., hard negative mining) are empirically shown to be inadequate for graph contrastive learning. How to mitigate the sampling bias on heterogeneous graphs is another important yet neglected problem. To address the aforementioned challenges, we propose a novel multi-view heterogeneous graph contrastive learning framework in this paper. We use metapaths, each of which depicts a complementary element of HINs, as the augmentation to generate multiple subgraphs (i.e., multi-views), and propose a novel pretext task to maximize the coherence between each pair of metapath-induced views. Furthermore, we employ a positive sampling strategy to explicitly select hard positives by jointly considering semantics and structures preserved on each metapath view to alleviate the sampling bias. Extensive experiments demonstrate MCL consistently outperforms state-of-the-art baselines on five real-world benchmark datasets and even its supervised counterparts in some settings.
更多查看译文
关键词
Applied mathematics,Computer science,Information technology,Science,Humanities and Social Sciences,multidisciplinary
AI 理解论文
溯源树
样例
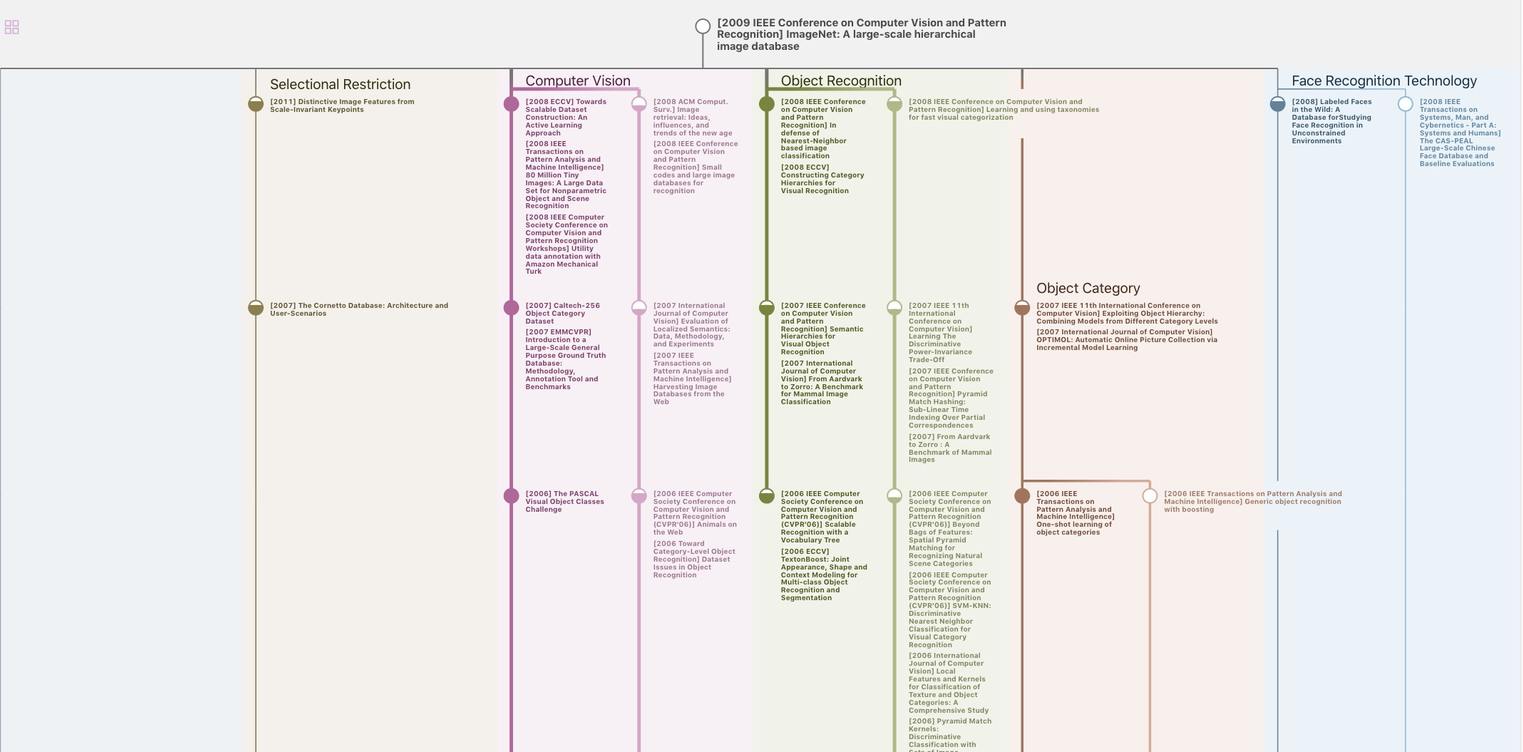
生成溯源树,研究论文发展脉络
Chat Paper
正在生成论文摘要