Learning Fluid Flow Visualizations From In-Flight Images With Tufts
IEEE Robotics and Automation Letters(2023)
摘要
To better understand fluid flows around aerial systems, strips of wire or rope, widely known as tufts, are often used to visualize the local flow direction. This letter presents a computer vision system that automatically extracts the shape of tufts from images, which have been collected during real flights of a helicopter and an unmanned aerial vehicle (UAV). As images from these aerial systems present challenges to both the model-based computer vision and the end-to-end supervised deep learning techniques, we propose a semantic segmentation pipeline that consists of three uncertainty-based modules namely, (a) active learning for object detection, (b) label propagation for object classification, and (c) weakly supervised instance segmentation. Overall, these probabilistic approaches facilitate the learning process without requiring any manual annotations of semantic segmentation masks. Empirically, we motivate our design choices through comparative assessments and provide real-world demonstrations of the proposed concept, for the first time to our knowledge.
更多查看译文
关键词
Aerial Systems: applications,computer vision for automation,object detection,segmentation and categorization,probability and statistical methods,aerodynamics
AI 理解论文
溯源树
样例
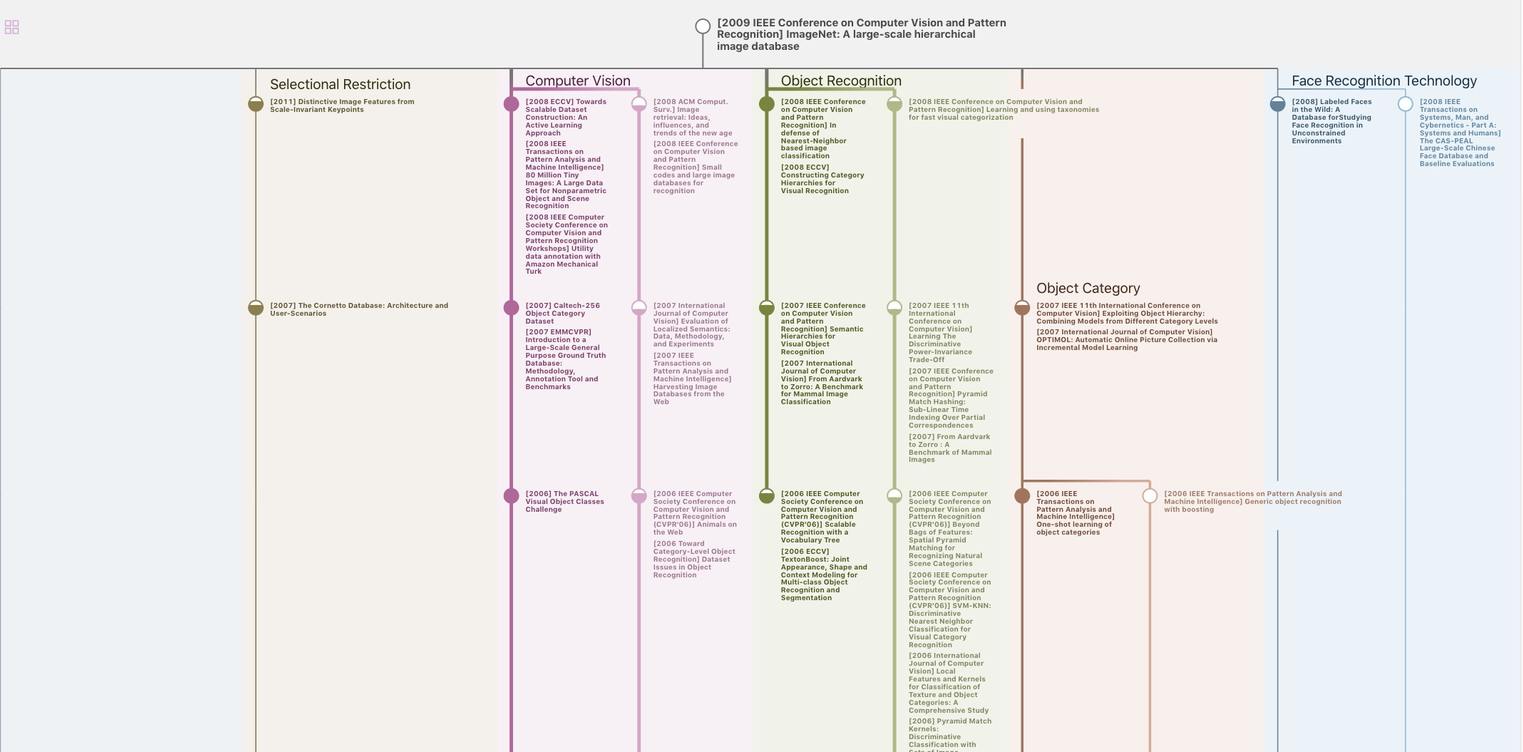
生成溯源树,研究论文发展脉络
Chat Paper
正在生成论文摘要