Low-cost predictive models of dementia risk using machine learning and exposome predictors
medRxiv (Cold Spring Harbor Laboratory)(2023)
摘要
Diagnosing dementia, a syndrome that currently affects more than 55 million people worldwide, remains a particularly challenging and costly task. It may involve undertaking several medical tests such as brain scans, cognitive tests and genetic tests to determine the presence and degree of cognitive decline. These procedures are associated with long procedures, subjective evaluations and high costs. As a result, patients are often diagnosed at a late stage, when symptoms become highly pronounced. Therefore, there is an urgent need for developing new strategies for early, accurate and cost-effective dementia screening and risk prediction. To overcome current limitations, we explored readily available exposome predictors for identifying individuals at risk of dementia and compared traditional statistical modeling and advanced machine learning. From approximately 500,000 participants from the UK Biobank, 1523 participants diagnosed with dementia after their baseline assessment visit were included in our study. An equal number of healthy participants were randomly selected as the control group by matching statistical age mean and sex distribution. This resulted in a total of 3046 participants being selected for our study; 2740 participants from 19 of the 22 UK Biobank assessment centers were used for internal validation, and 306 participants from the remaining three centers were selected for external validation. We include data from the participants' baseline visit and selected 128 low-cost exposome factors related to life course exposures that may be easily acquired through simple questionnaires. Subsequently, data imputation was performed to account for missing patient data. Two different predictive models were assessed for discriminating between participants that remained healthy and participants diagnosed with dementia after the baseline visit, i.e. (1) a classical logistic regression linear classifier and (2) a machine learning ensemble classifier based on XGBoost. We interpreted the results by estimating feature importance within the predictive models. Our results demonstrate that machine learning models based on exposome data can reliably identify individuals that will be diagnosed with dementia. The XGBoost based model outperforms logistic regression model, achieving a mean AUC of 0.88 in the external validation tests. We identified novel exposome factors that might be combined as potential markers for dementia, such as facial aging, the frequency of use of sun/ultraviolet light protection, and the length of mobile phone use. Finally, we propose a novel neurocognitive assessment test that could be used as an online tool to screen individuals at risk of dementia for enrolment in preventive interventions and future clinical trials. Keywords: Machine learning, dementia, risk prediction, exposome predictors, low-cost prediction.
### Competing Interest Statement
The authors have declared no competing interest.
### Funding Statement
This research has received funding from the European Union's Horizon 2020 research and innovation program under Grant Agreement Number 848158 (EarlyCause) and Grant Agreement Number 874739 (LongITools), and from the Spanish Ministry of Science, Innovation, and Universities within the framework of the 'Retos Investigacion' program, project RTI2018-099898-B-I00 (HeartBrainCom).
### Author Declarations
I confirm all relevant ethical guidelines have been followed, and any necessary IRB and/or ethics committee approvals have been obtained.
Yes
The details of the IRB/oversight body that provided approval or exemption for the research described are given below:
The UK Biobank study was approved by the National Information Governance Board for Health and Social Care and the National Health Service North West Multicentre Research Ethics Committee. All participants provided signed informed consent at enrolment and all research was performed in accordance with relevant guidelines/regulations. All data used in this analysis is available through application to the UK Biobank.
I confirm that all necessary patient/participant consent has been obtained and the appropriate institutional forms have been archived, and that any patient/participant/sample identifiers included were not known to anyone (e.g., hospital staff, patients or participants themselves) outside the research group so cannot be used to identify individuals.
Yes
I understand that all clinical trials and any other prospective interventional studies must be registered with an ICMJE-approved registry, such as ClinicalTrials.gov. I confirm that any such study reported in the manuscript has been registered and the trial registration ID is provided (note: if posting a prospective study registered retrospectively, please provide a statement in the trial ID field explaining why the study was not registered in advance).
Yes
I have followed all appropriate research reporting guidelines, such as any relevant EQUATOR Network research reporting checklist(s) and other pertinent material, if applicable.
Yes
The data that support the findings of this study are available from UK Biobank, but restrictions apply to the availability of these data, which were used under license for the current study, and so are not publicly available. Data are however available from the authors upon reasonable request and with permission of UK Biobank.
更多查看译文
关键词
dementia risk,exposome predictors,predictive models,machine learning,low-cost
AI 理解论文
溯源树
样例
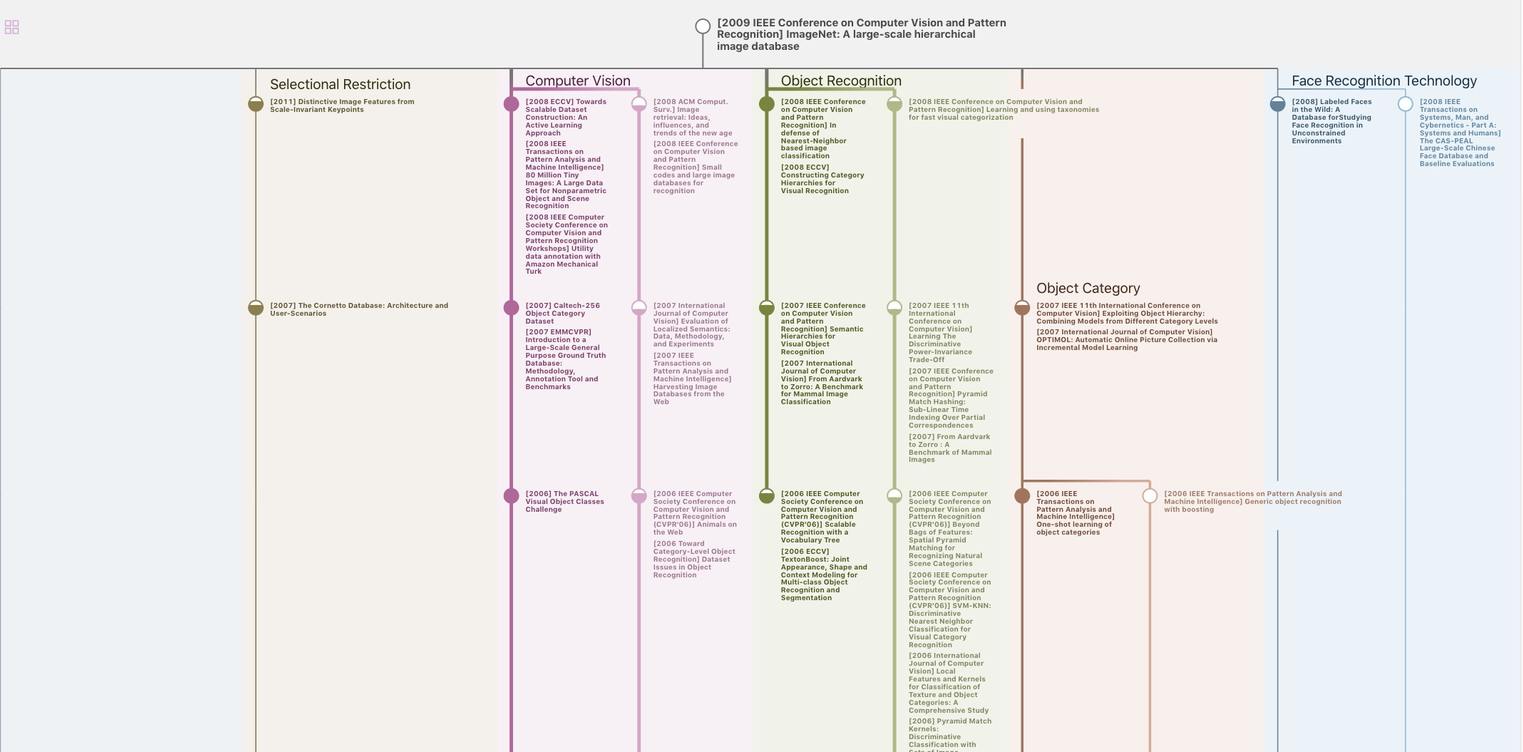
生成溯源树,研究论文发展脉络
Chat Paper
正在生成论文摘要