Using Double Deep Q-Learning to learn Attitude Control of Fixed-Wing Aircraft
SITIS(2022)
摘要
These last few years have been big for the field of Reinforcement Learning. While is was long thought to be impossible for Reinforcement Learning to master complex environments, recent research has seen Reinforcement Learning agent master highly complex tasks like Atari Games, Go, Dota 2, Autonomous transportation among many others. This has sparked a new wave of interest into Reinforcement Learning, with most of that focus directed towards Deep Reinforcement Learning algorithms. This paper will explore the realm of aviation and apply Deep Reinforcement learning to it. To be more specific, we will train an agent with Double Deep Q-Learning to learn how to control the planes attitude control. The QPlane toolkit will be used for this, and we will utilize both simulators provided. The methodology and results will be presented in this paper.
更多查看译文
关键词
Reinforcement Learning, Deep Reinforcement Learning, Deep Learning, Double Deep Q-Learning, Deep Q-Learning, Q-Learning, DQN, DDQN, Fixed-Wing, Attitude Control
AI 理解论文
溯源树
样例
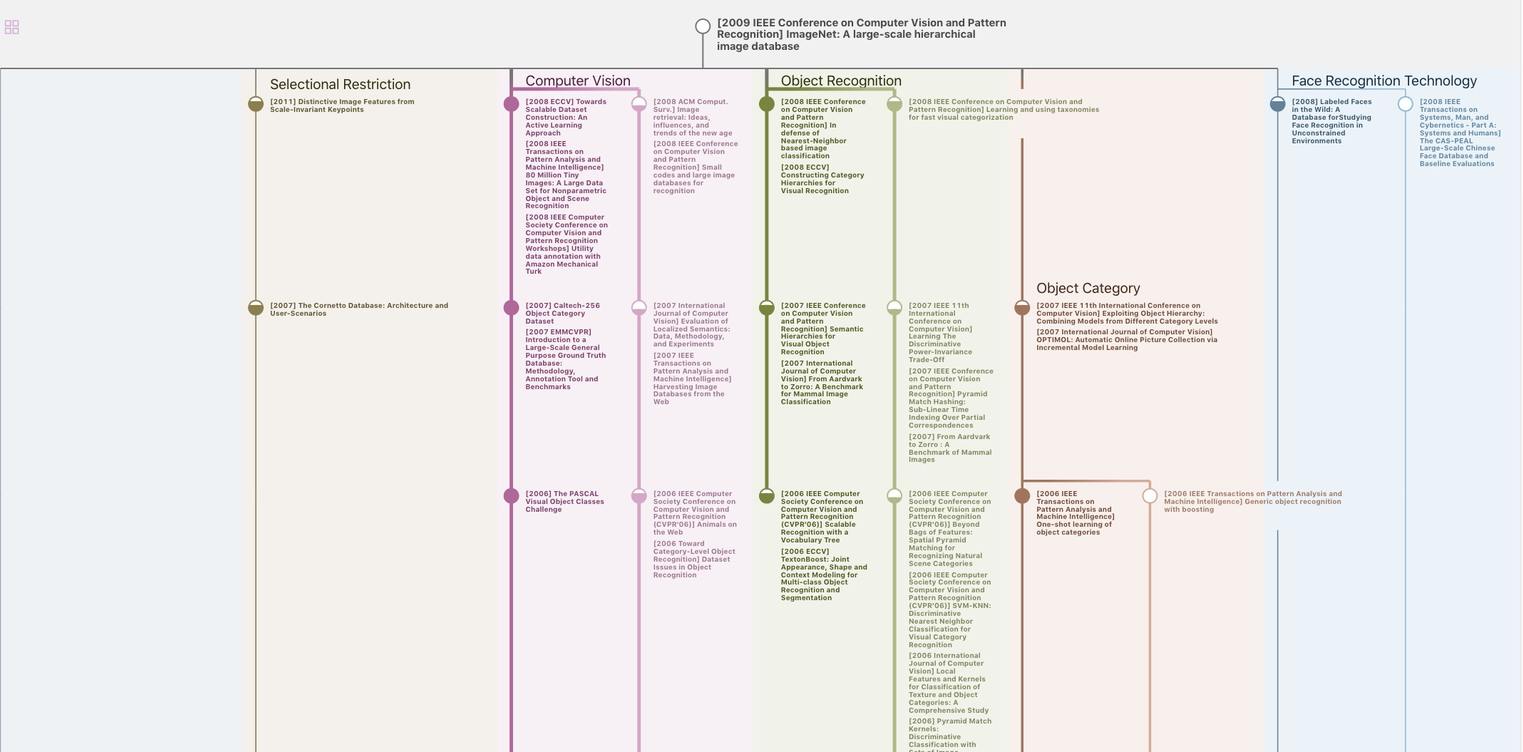
生成溯源树,研究论文发展脉络
Chat Paper
正在生成论文摘要