ECG, EEG, Breathing Signals, and Machine Learning: Computer-Aided Detection of Obstructive Sleep Apnea Syndrome and Depression.
CinC(2022)
摘要
Obstructive Sleep Apnea Syndrome (OSAS) and Major Depressive Disorder (MDD) are both common conditions associated with poor quality of life. We seek to classify OSAS and depression in OSAS patients, as well as sleep stages using multiple machine learning techniques. We have extracted features from 5-minute intervals of electrocardiograms (ECG), breathing signals, and electroen-cephalograms (EEG) recorded from a total of 118 subjects, of which 89 are used for training and 10-fold cross-validation and 29 are used for testing or a 75/25% split. The best classification performance of OSAS was obtained with light sleep and deep sleep with ReliefF using random forest and boosted trees, respectively. It has yielded an accuracy of 100.00%, F1-Score of 100.00%, Cohen's k Coefficient of 1.00, and a Matthews correlation coefficient (MCC) of 1.00. All sleep stages with 10 principal components using random forest yielded an accuracy of 77.50%, F1-Score of 78.05%, Cohen's k of 0.571, and an MCC of 0.632 for classification of depression in OSAS patients. Sleep staging was best done using bagged trees with features selected via sequential backward feature selection, yielding an accuracy of 76.90%, F1-Score of 75.90%, Cohen's k of 0.480, and an MCC of 0.634. These results show promise in detecting OSAS and depression in OSAS patients, particularly using light and deep sleep data.
更多查看译文
关键词
10-fold cross-validation,5-minute intervals,computer-aided detection,deep sleep,depression,ECG,F1-Score,light sleep,MCC,multiple machine learning techniques,obstructive Sleep Apnea Syndrome,Obstructive Sleep Apnea Syndrome,OSAS patients,random forest,sleep stages,Sleep staging
AI 理解论文
溯源树
样例
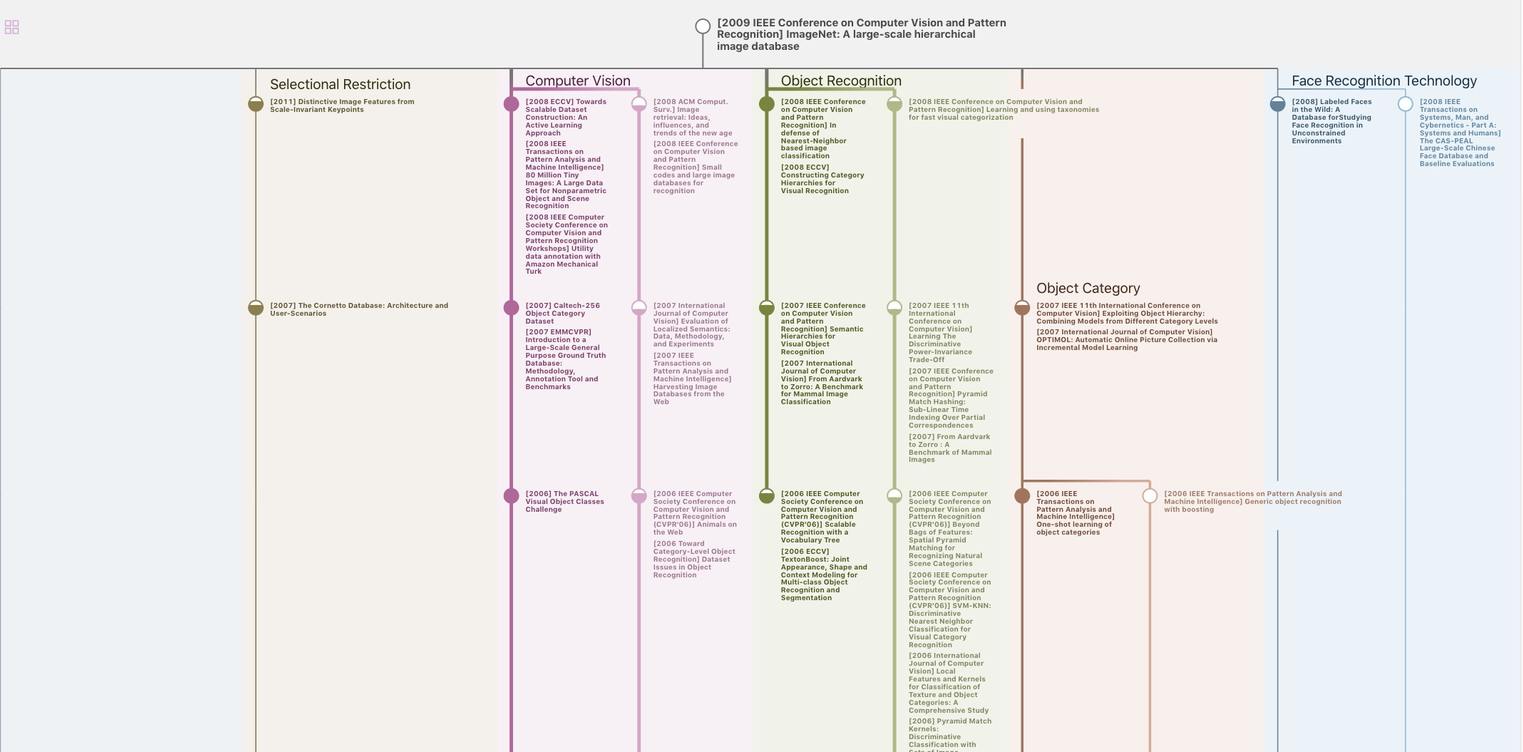
生成溯源树,研究论文发展脉络
Chat Paper
正在生成论文摘要