Genetic justification of COVID-19 patient outcomes using DERGA, a novel data ensemble refinement greedy algorithm
JOURNAL OF CELLULAR AND MOLECULAR MEDICINE(2024)
摘要
Complement inhibition has shown promise in various disorders, including COVID-19. A prediction tool including complement genetic variants is vital. This study aims to identify crucial complement-related variants and determine an optimal pattern for accurate disease outcome prediction. Genetic data from 204 COVID-19 patients hospitalized between April 2020 and April 2021 at three referral centres were analysed using an artificial intelligence-based algorithm to predict disease outcome (ICU vs. non-ICU admission). A recently introduced alpha-index identified the 30 most predictive genetic variants. DERGA algorithm, which employs multiple classification algorithms, determined the optimal pattern of these key variants, resulting in 97% accuracy for predicting disease outcome. Individual variations ranged from 40 to 161 variants per patient, with 977 total variants detected. This study demonstrates the utility of alpha-index in ranking a substantial number of genetic variants. This approach enables the implementation of well-established classification algorithms that effectively determine the relevance of genetic variants in predicting outcomes with high accuracy.
更多查看译文
关键词
artificial intelligence,classification algorithms,COVID-19,DERGA,genetic,SARS-CoV2,variants
AI 理解论文
溯源树
样例
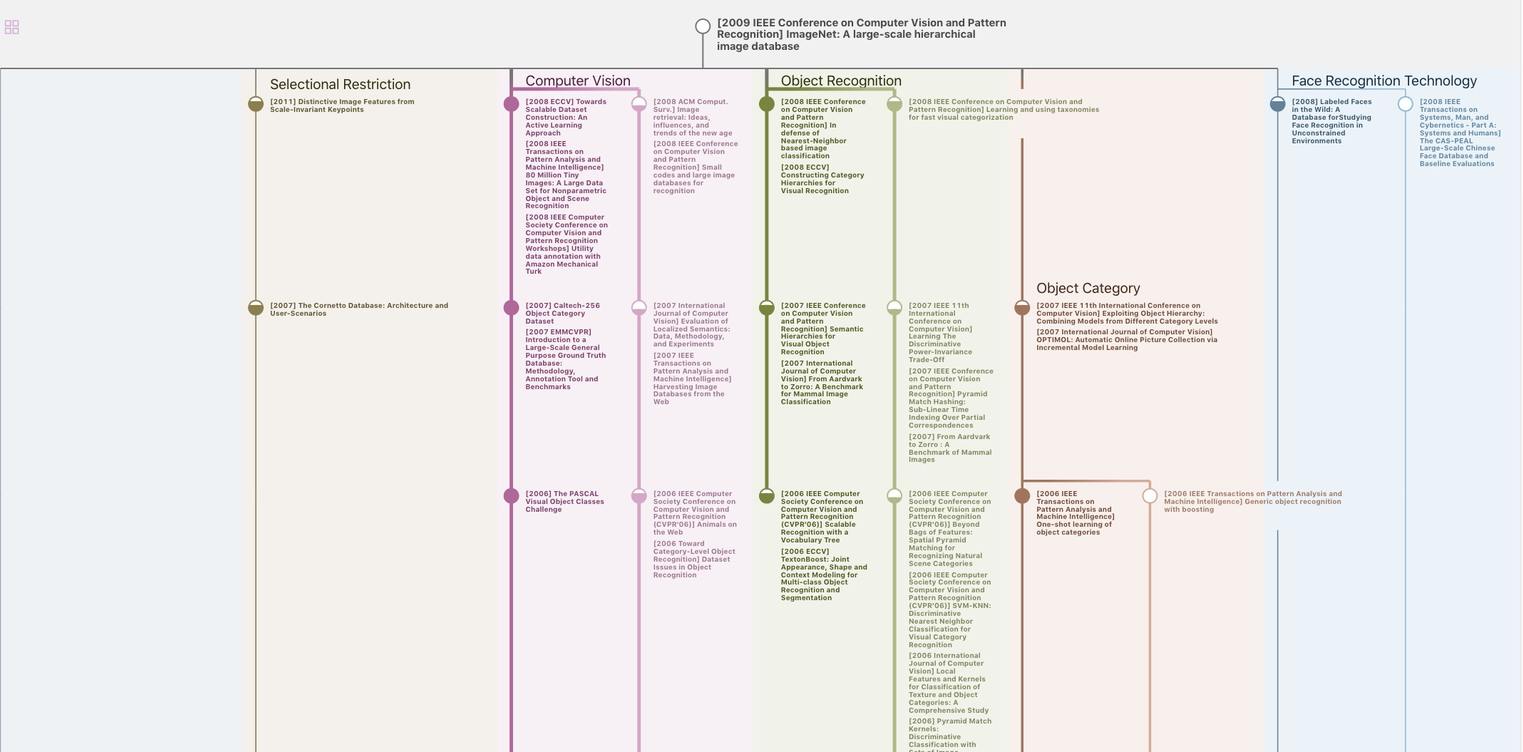
生成溯源树,研究论文发展脉络
Chat Paper
正在生成论文摘要