Overcoming the Pitfalls of HPC-based Cryptojacking Detection in Presence of GPUs.
CODASPY(2023)
摘要
With the rising number of devices connected to the internet, the number of cyber-attacks on these devices increases in parallel. There are several strategies that an attacker can pursue, like stealing intellectual property of a victim or encrypting data to demand ransom for the decryption. In this work, we are focusing on the detection of so called cryptojacking attacks, in which an attacker that gained access to a system, then introduces programs that use the processing power of the victim device to mine cryptocurrencies. The presence of such an attack is not obvious right away and the longer an attacker manages to remain undetected, the longer they can profit having the victim foot the power bill. In this study, we combine previous approaches to demonstrate that cryptojacking attacks can be detected with an accuracy of 96% by leveraging hardware performance counters on the Windows operating system. Further, we present a method to determine which performance events result in the best detection rates, thus allowing the selection of a few performance events that can be monitored simultaneously by modern consumer CPUs. In a next step, we show that the CPU counters-based detection mechanism fails when an attacker switches from using the CPU resources to GPUs for the mining tasks. Based on these findings we then improve the previous detection approaches by extending the CPU performance counters with GPU-specific metrics resulting in 99.86% accuracy for the GPU-based cryptojacking attack class. In addition to a high detection rate the presented approach only causes a negligible performance loss while monitoring the whole system, which allows for continuous monitoring of live systems.
更多查看译文
AI 理解论文
溯源树
样例
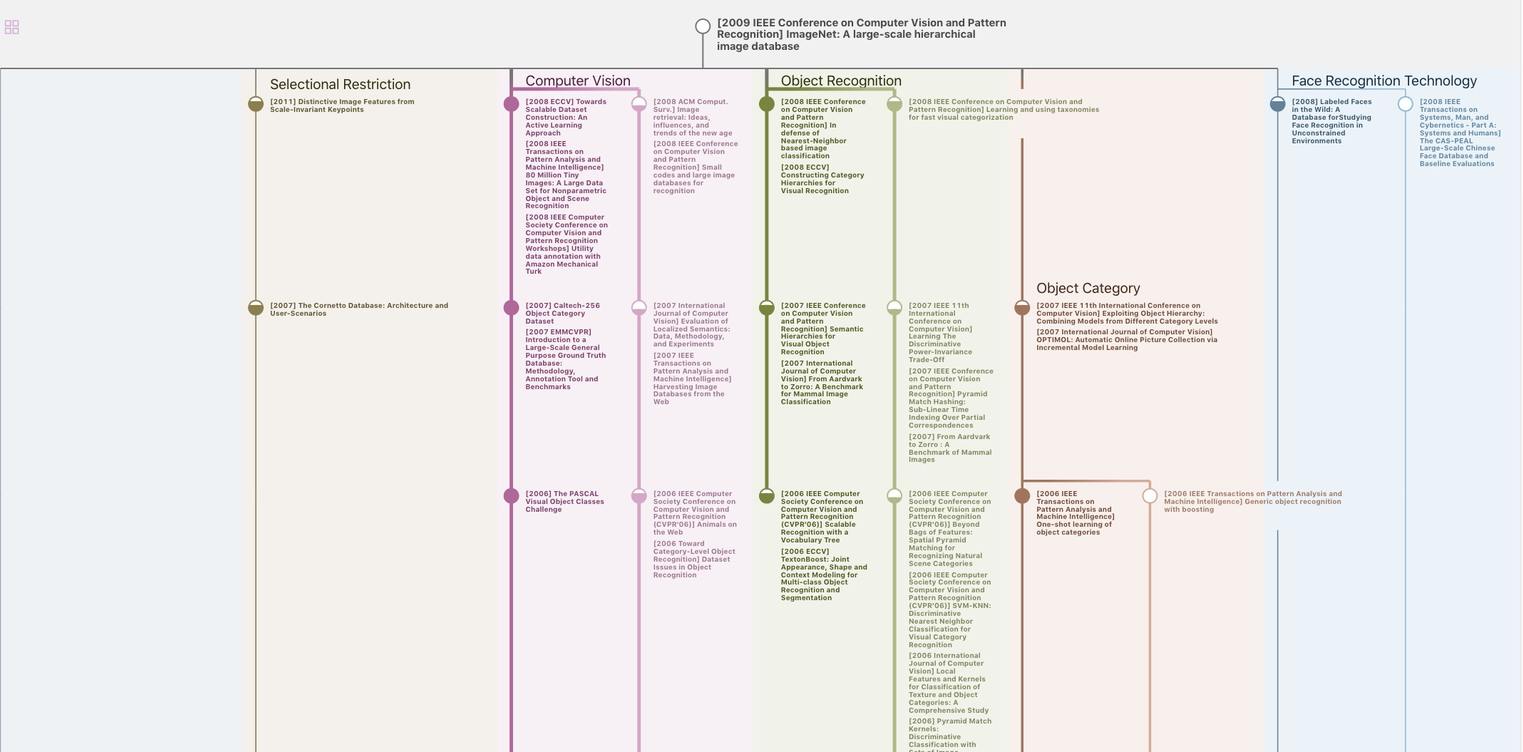
生成溯源树,研究论文发展脉络
Chat Paper
正在生成论文摘要