Learning Local Representation by Gradient-Isolated Memorizing of Spiking Neural Network.
HPCC/DSS/SmartCity/DependSys(2022)
摘要
Spiking neural networks (SNNs) show promise in implementing low-power AI hardware due to their event-driven sparsity and leaky integrate-and-fire (LIF) neurons with binary spikes, rather than traditional activation functions with analog output. However, SNNs have a low capacity to process the local input due to their spike encoding paradigm. To address these problems, we propose a novel SNN architecture with a gradient-isolated memorizing mechanism, horizontal LIF, and vertical LIF neurons to learn local representation. Moreover, to take full advantage of local representations and global representations, we design a concurrent SNN architecture within the local-global joint representation. For proof-of-concept, we evaluate our proposal on MNIST, Fashion MNIST, and CIFAR-10 datasets, which achieve 98.68% and 90.37%, and 87.19% accuracy with 5 time steps, respectively, while maintaining low computational complexity.
更多查看译文
关键词
Spiking neural network,leaky integrate and fire neuron,gradient-isolated memorizing,local representation
AI 理解论文
溯源树
样例
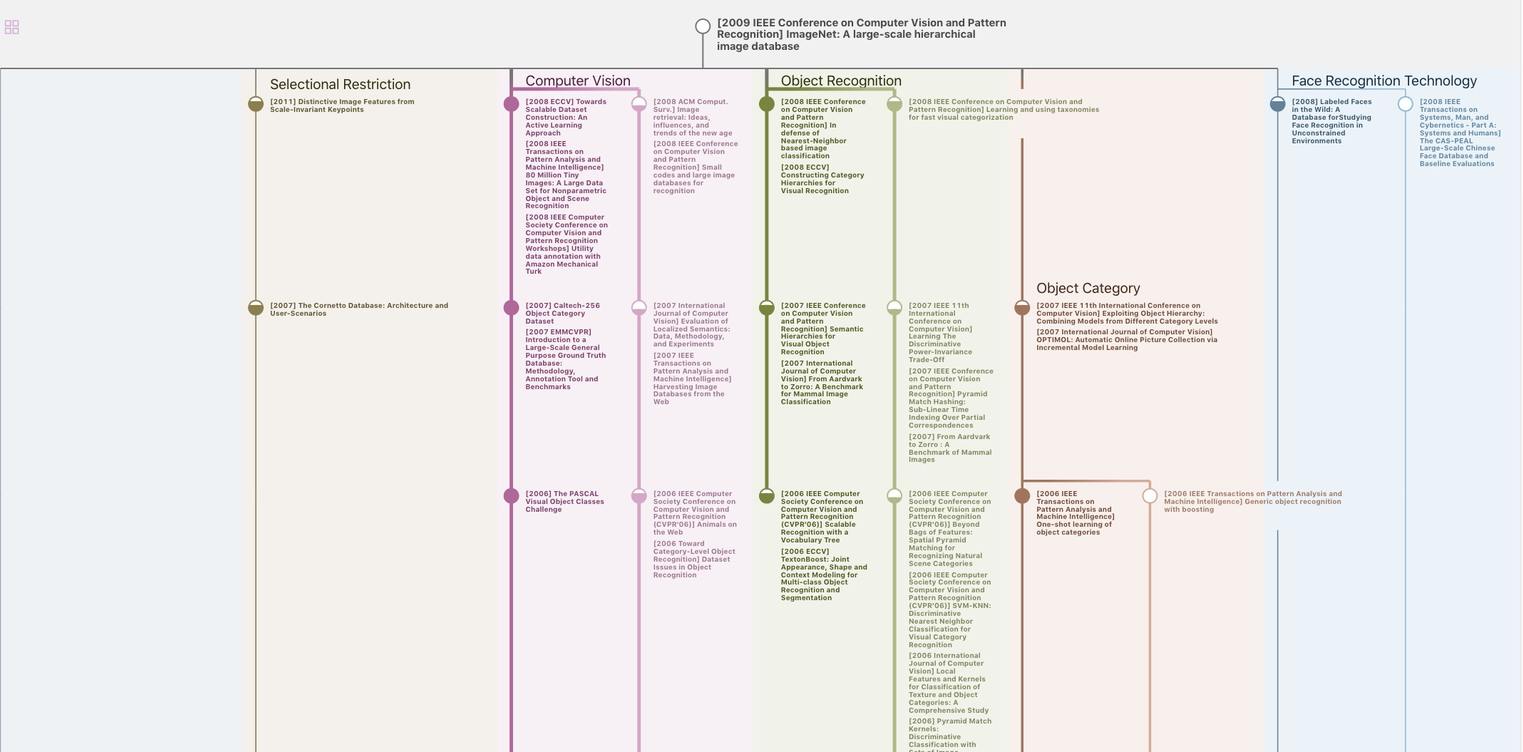
生成溯源树,研究论文发展脉络
Chat Paper
正在生成论文摘要