Graph clustering under weight-differential privacy
HPCC/DSS/SmartCity/DependSys(2022)
摘要
In this paper, we consider the weight privacy problem in the undirected weighted graph where the graph structure is public and the edge-weights are private in the weightprivacy model. Specificially, we study the k-median and k-center problems based on the shortest path with weight-differential privacy, which aims to partition the graph vertex set into k clusters to minimize the average and maximum distance of vertex to its cluster center. For the k-median problem, we reformulate it into the equivalent submodular problem and provide a
$(1-\frac{1}{e})$
approximate guarantee with an additive error. For the k-center problem, our private algorithms offer 2-approximations with an additive error. Moreover, our private algorithms achieve an almost linear number of function evaluations by applying the sampling technique. Finally, we evaluate the performance of our algorithms against the existing work and the experimental results demonstrate the effectiveness of our algorithms.
更多查看译文
关键词
differential privacy,graph clustering,weighted graph
AI 理解论文
溯源树
样例
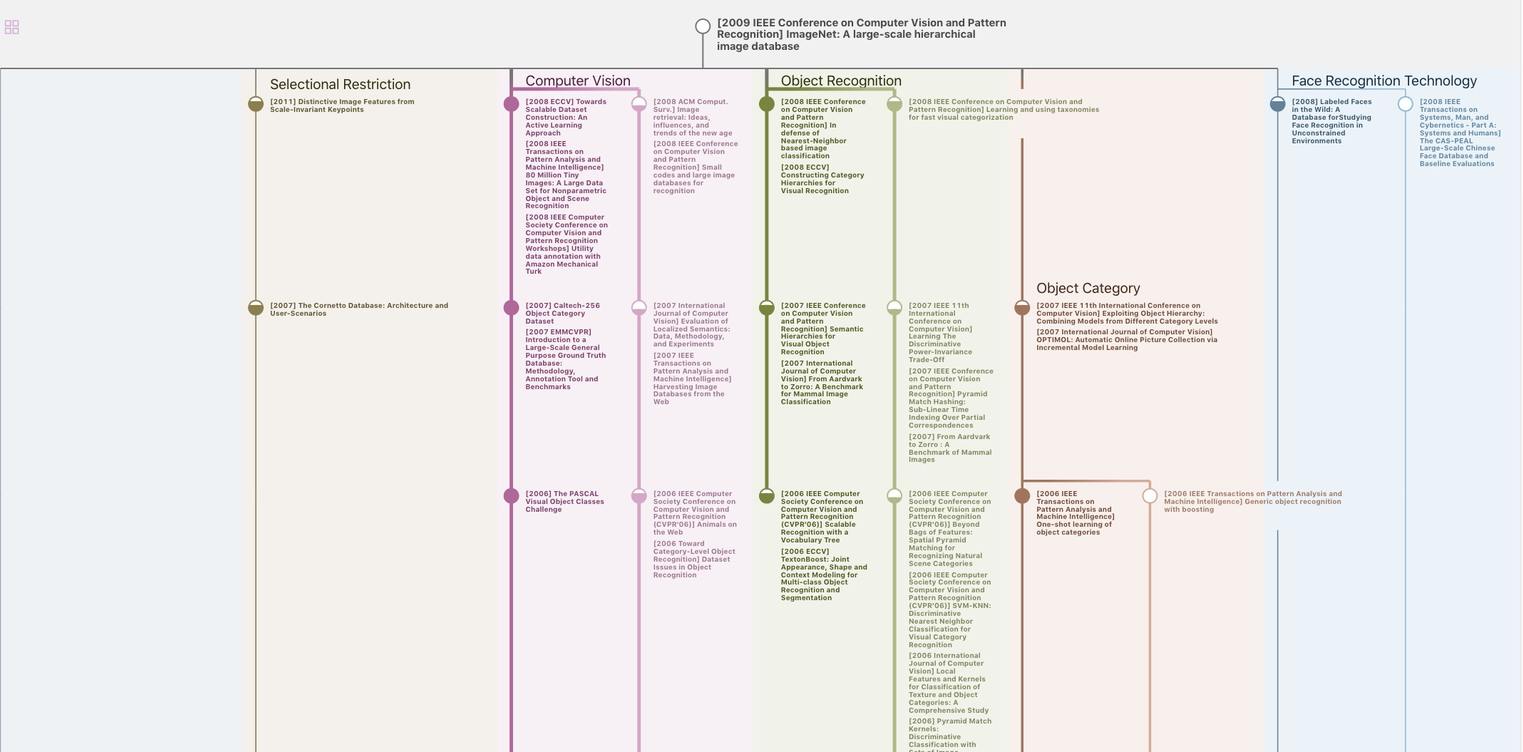
生成溯源树,研究论文发展脉络
Chat Paper
正在生成论文摘要