SupportHDC: Hyperdimensional Computing with Scalable Hypervector Sparsity
NICE(2023)
摘要
Hyperdimensional Computing (HDC) is an emerging brain-inspired machine learning method that is recently gaining much attention for performing tasks such as pattern recognition and bio-signal classification with ultra-low energy and area overheads when implemented in hardware. HDC relies on the encoding of input signals into binary or few-bit Hypervectors (HVs) and performs low-complexity manipulations on HVs in order to classify the input signals. In this context, the sparsity of HVs directly impacts energy consumption, since the sparser the HVs, the more zero-valued computations can be skipped. This short paper introduces SupportHDC, a novel HDC design framework that can jointly optimize system accuracy and sparsity in an automated manner, in order to trade off classification performance and hardware implementation overheads. We illustrate the inner working of the framework on two bio-signal classification tasks: cancer detection and arrhythmia detection. We show that SupportHDC can reach a higher accuracy compared to the conventional splatter-code architectures used in many works, while enabling the system designer to choose the final design solution from the accuracy-sparsity trade-off curve produced by the framework. We release the source code for reproducing our experiments with the hope of being beneficial to future research.
更多查看译文
关键词
Hyperdimensional computing, sparsity-aware computing, ultra-low-power computing, automated system design
AI 理解论文
溯源树
样例
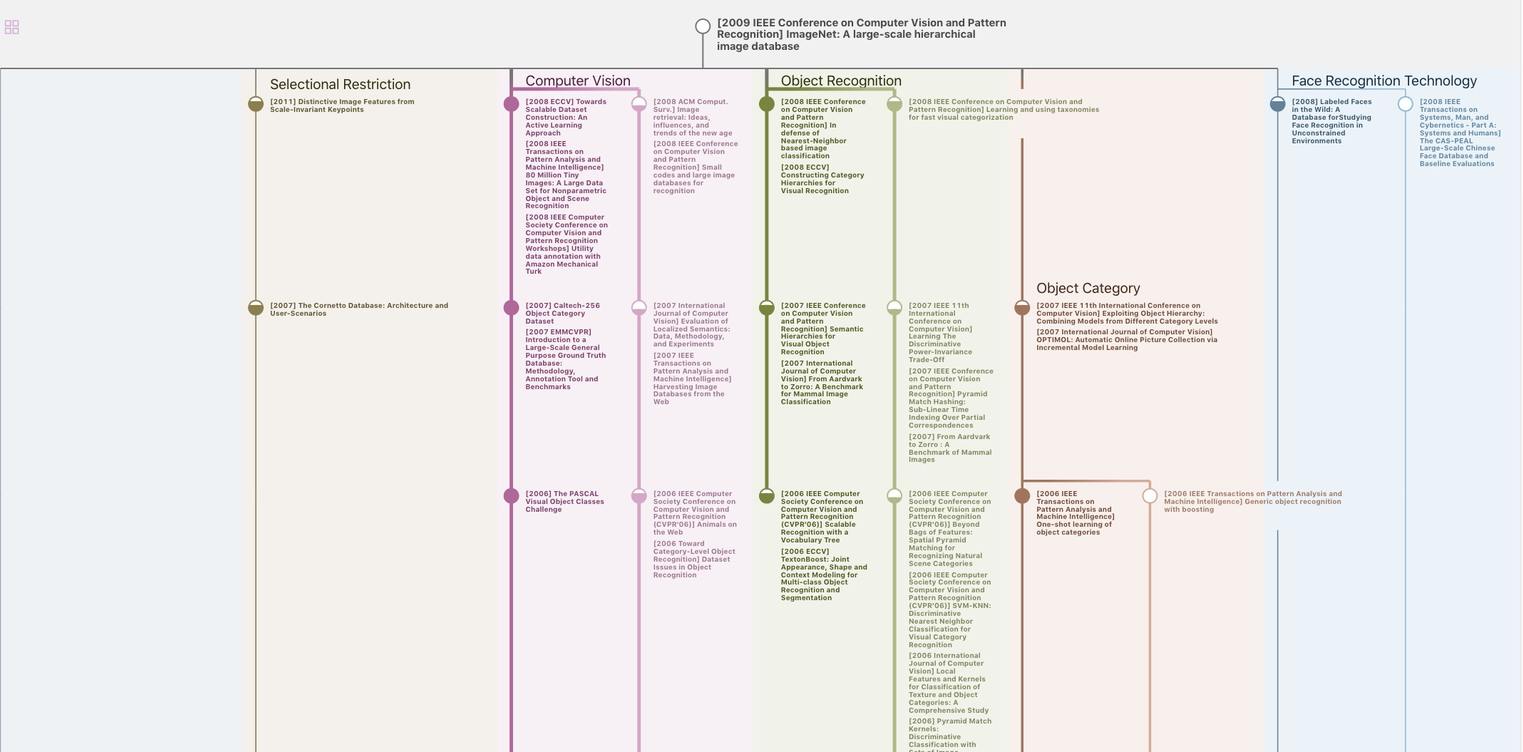
生成溯源树,研究论文发展脉络
Chat Paper
正在生成论文摘要