A Discriminative DeepLab Model (DDLM) for Surface Anomaly Detection and Localization.
CCWC(2023)
摘要
Recent reconstructive model-based visual anomaly detection (AD) methods have been shown to reconstruct the normal regions of the image but often struggle to detect the required anomalous deviations. These approaches are typically trained on images without anomalies and consequently require manual post-processing techniques to localize the anomalies. In this paper, we proposed a discriminative deeplab model that is trained discriminatively end-to-end on out-of-distribution simulated anomalies. Our proposed framework directly localizes anomalies without the need for complex post-processing via learning a combined representation of anomalous images and a decision boundary between anomalous and normal samples. The effectiveness of our DDLM is evaluated extensively on the MVTec AD dataset. Our results show that the DDLM framework outperformed state-of-the-art frameworks between 1.5% and 18.8% in AUROC on the anomaly detection challenge and between 20.2% and 22.9% with regards to the AP on the anomaly localization challenge.
更多查看译文
关键词
Anomaly Detection, Anomaly Localization, Reconstructive sub-network, Discriminative sub-network
AI 理解论文
溯源树
样例
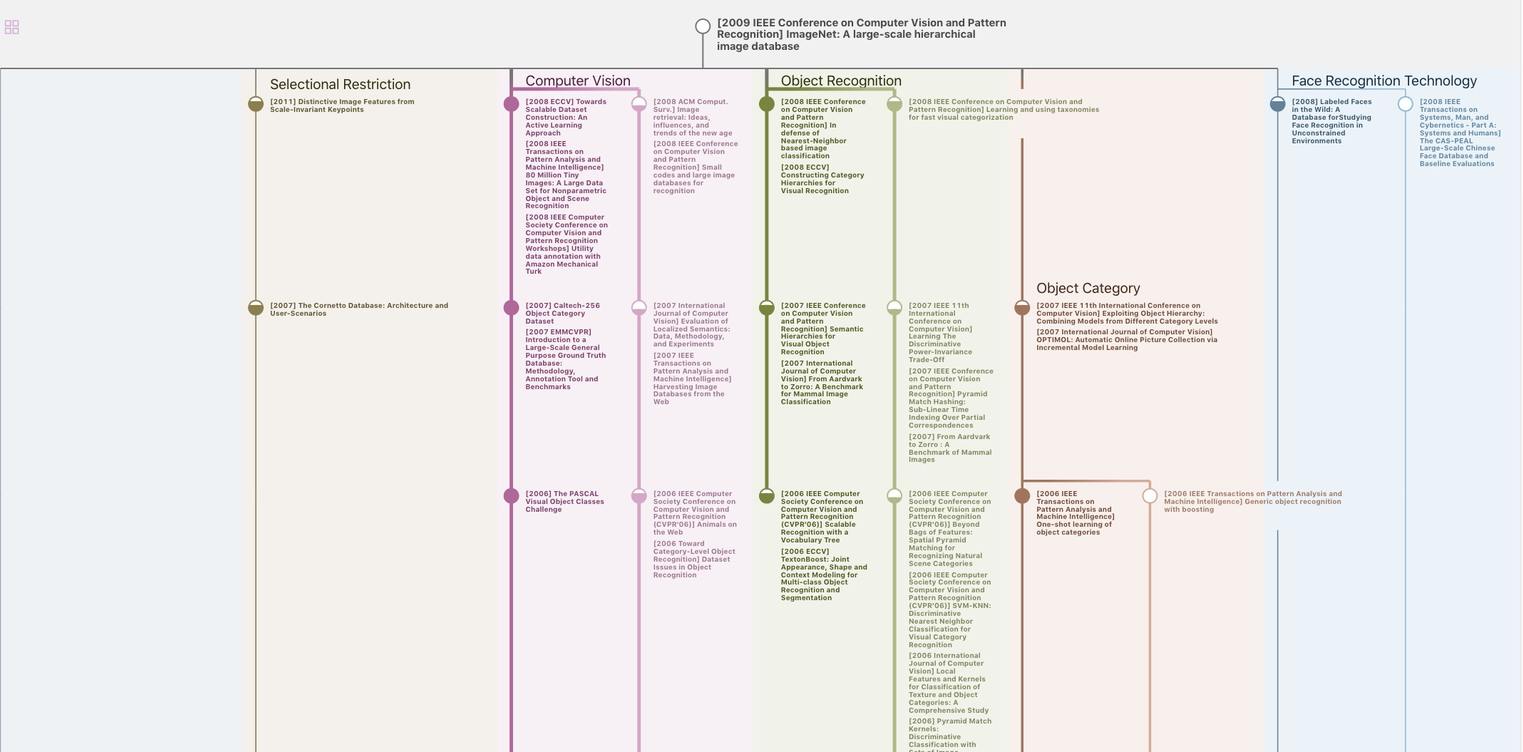
生成溯源树,研究论文发展脉络
Chat Paper
正在生成论文摘要