Medical Visual Question Answering via Targeted Choice Contrast and Multimodal Entity Matching.
ICONIP (2)(2022)
摘要
Although current methods have advanced the development of medical visual question answering (Med-VQA) task, two aspects remain to be improved, namely extracting high-level medical visual features from small-scale data and exploiting external knowledge. To strengt-hen the performance of Med-VQA, we propose a pre-training model called Targeted Choice Contrast (TCC) and a Multimodal Entity Matc-hing (MEM) module, and integrate them into an end-to-end framework. Specifically, the TCC model extracts deep visual features on the small-scale medical dataset by contrastive learning. It improves model robustness by a targeted selection of negative samples. The MEM module is dedicated to embedding knowledge representation into the framework more accurately. Besides, we apply a mixup strategy for data augmentation during the framework training process to make full use of the small-scale images. Experimental results demonstrate our framework outperforms state-of-the-art methods.
更多查看译文
关键词
choice contrast,medical
AI 理解论文
溯源树
样例
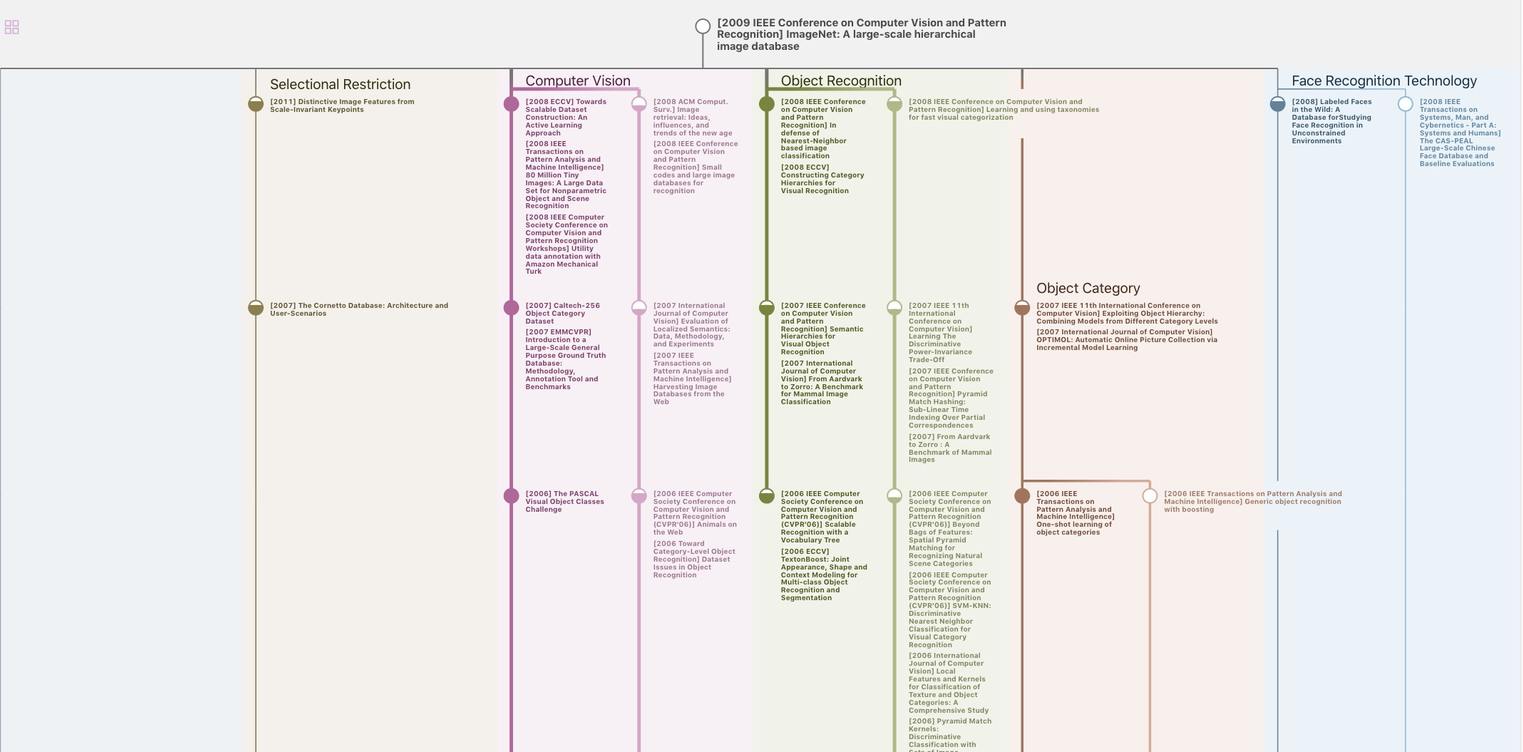
生成溯源树,研究论文发展脉络
Chat Paper
正在生成论文摘要