Self-attention Based Multi-scale Graph Convolutional Networks.
ICONIP (1)(2022)
摘要
Graph convolutional networks (GCNs) have achieved remarkable learning ability for dealing with various graph structural data recently. In general, GCNs have low expressive power due to their shallow structure. In this paper, to improve the expressive power of GCNs, we propose two multi-scale GCN frameworks by incorporating self-attention mechanism and multi-scale information into the design of GCNs. The self-attention mechanism allows us to adaptively learn the local structure of the neighborhood, and achieves more accurate predictions. Extensive experiments on both node classification and graph classification demonstrate the effectiveness of our approaches over several state-of-the-art GCNs.
更多查看译文
关键词
networks,self-attention,multi-scale
AI 理解论文
溯源树
样例
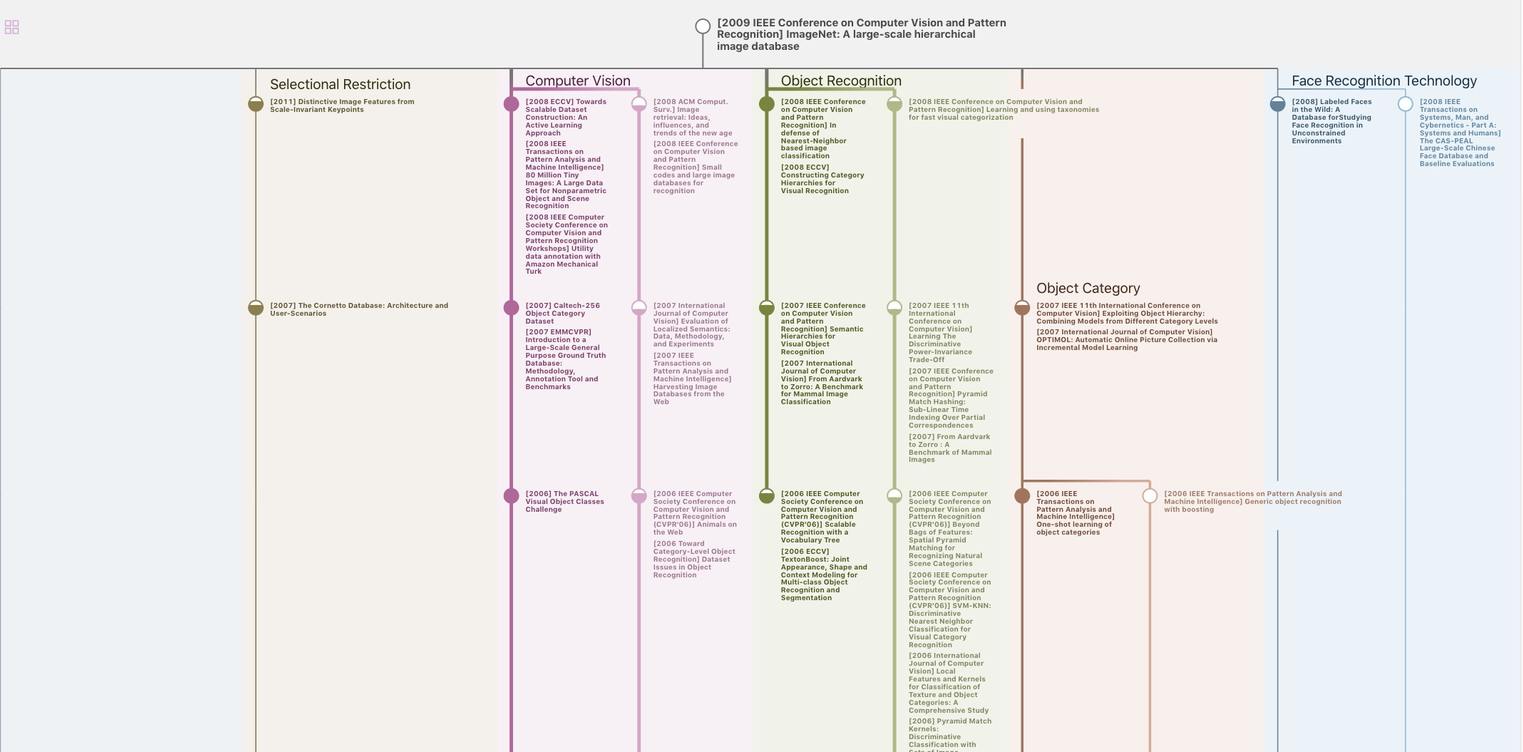
生成溯源树,研究论文发展脉络
Chat Paper
正在生成论文摘要