Additional Learning for Joint Probability Distribution Matching in BiGAN.
ICONIP (1)(2022)
摘要
Bidirectional Generative Adversarial Networks (BiGANs) is a generative model with an invertible mapping between latent and image space. The mapping allows us to encode real images into latent representations and reconstruct input images. However, from preliminary experiments, we found that the joint probability distributions learned by the generator and the encoder are inconsistent, leading to poor-quality mapping. Therefore, to solve this issue, we propose an architecture-agnostic additional learning method to make the two joint probability distributions closer. In the experiments, we evaluated the reconstruction quality on synthetic and natural image datasets and found that our additional learning improves the invertible mapping of BiGAN.
更多查看译文
关键词
joint probability distribution matching,learning
AI 理解论文
溯源树
样例
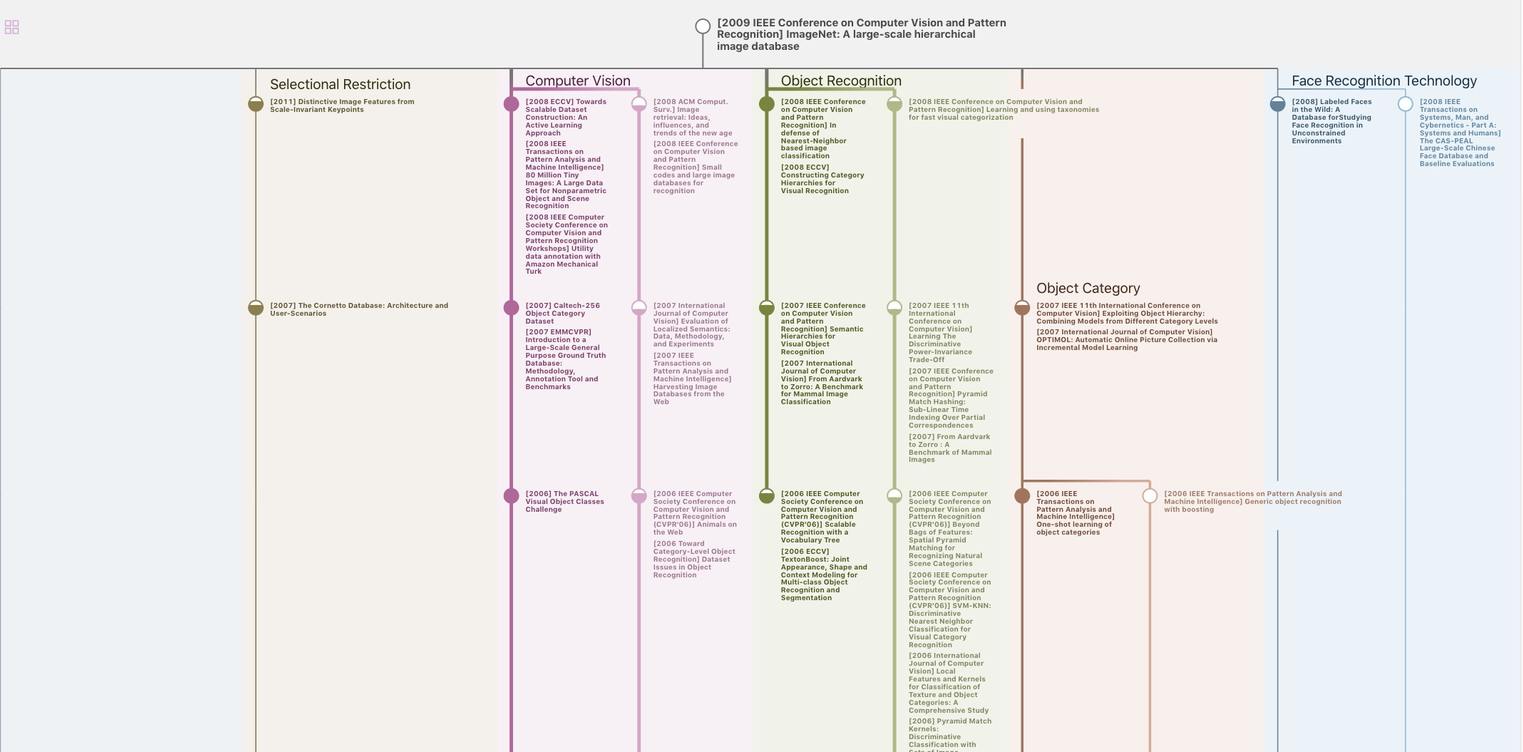
生成溯源树,研究论文发展脉络
Chat Paper
正在生成论文摘要