Dynamically Connected Graph Representation for Object Detection.
ICONIP (3)(2022)
摘要
Graph models have been applied to object detection task to implement relational reasoning in many works, achieving a tremendous advancement in performance. However, all these models focus on graph representation with shallow layers and the performance decreases with more graph layers. The most crucial problem lies in that deep graph models encounter the vanishing gradient problem, which causes over-smoothing and no diversity among the features of graph nodes. This paper proposes a novel Dynamically Connected Graph (DCG) representation mechanism to break the limitation of the vanishing gradient problem in deep graph-based detection models and make the graph models deeper and better. DCG adaptively learns the feature re-usage and new feature exploration for better optimization of the deep graph. Deep graph models still meet another general problem, i.e., the class imbalance of the original data distribution causes an imbalance in graph node learning. Thus, a Node Balanced Loss is further introduced to address this issue by normalizing and reassigning the weights of losses of various classes. Our method can be easily integrated into existing detection models without extra modification. Experiments prove that DCG significantly improves the performance of various baseline models on the challenging MS-COCO and PascalVOC.
更多查看译文
关键词
connected graph representation,connected graph,detection,object
AI 理解论文
溯源树
样例
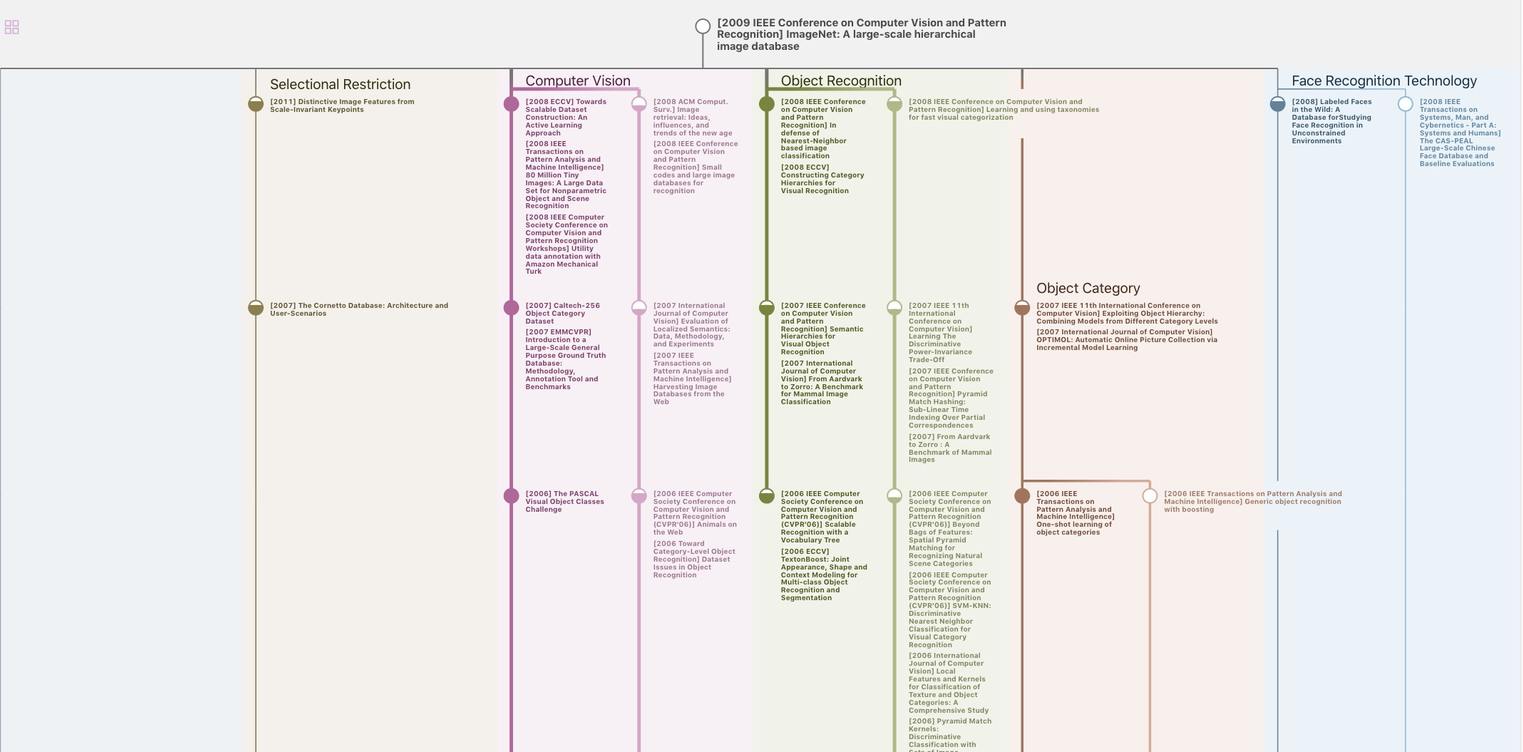
生成溯源树,研究论文发展脉络
Chat Paper
正在生成论文摘要