Improving Knowledge Graph Embedding Using Dynamic Aggregation of Neighbor Information.
ICONIP (4)(2022)
摘要
Knowledge graph embedding represents the embedding of entities and relations in the knowledge graph into a low-dimensional vector space to accomplish the knowledge graph complementation task. Most existing knowledge graph embedding models such as TransE and RotatE based on translational distance models only consider triple-level information. Considering the rich contextual information of entities in the graph, we propose a new approach named DAN, which is able to dynamically aggregate neighbor information. Firstly, the relation is regarded as a transformation operation on heterogeneous neighbors. Neighborhood information is transformed into an homogeneous space with the central node. This solves the problem of transformation between heterogeneous nodes in the knowledge graph. Then, by dynamically aggregating neighbor information, the same entity owns different information embedding in different triples. This method enriches the representation of entity information and can be combined with other embedding models as a general method. We combine our approach with TransE and RotatE models. The experimental results show that DAN can improve the accuracy of the original model. Our best result outperforms the existing state-of-the-art models in link prediction. While our method has a faster convergence rate and higher accuracy with a slightly increased number of parameters.
更多查看译文
关键词
Knowledge Graph, Link Prediction, Graph Learning
AI 理解论文
溯源树
样例
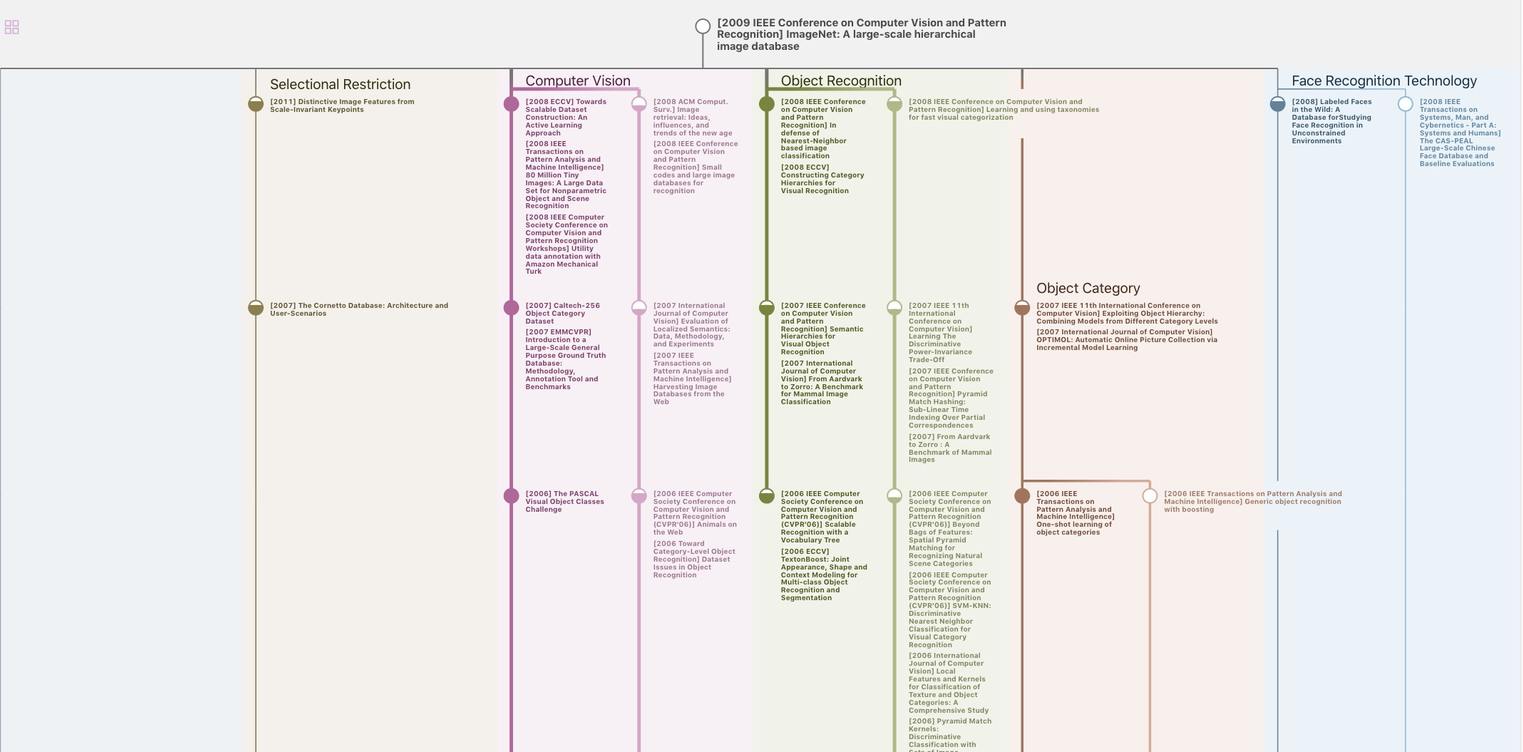
生成溯源树,研究论文发展脉络
Chat Paper
正在生成论文摘要