Few Shot Feature Extraction Network Based on Autoencoder.
RICAI(2022)
摘要
Few Shot Object Detection is becoming a hot issue in the problem of deep learning object detection. Its main goal is to use very few samples for training and learning to obtain a model with good detection speed and accuracy. However, the most significant problem of few shot object detection detection is that the extraction of target feature information is insufficient and incomplete due to the sparse sample data. The solution to this problem in main methods is often to increase the number of samples through data enhancement, which can greatly enhance sample feature information. However, there is still the problem of inaccurate extraction of target feature information. This paper proposes a feature extraction network based on an autoencoder, that is, under the framework of autoEncode, refer to the network structure of Vgg-16 and integrate the SeNet channel attention mechanism to adjust the channel feature information weight parameters in the feature extraction network, and then design the similarity evaluation method between the sample input image and the reconstructed image based on the autoencoder is used to quantify the index representation feature extraction ability. Finally, the experiments show, compared with other main feature extraction algorithms, our method proposed in this paper has a better performance on the feature extraction ability index on the small-sample fine-grained dataset Oxford-102 Flower, and the speed reaches the level of main algorithm, which shows that the scheme proposed in this paper effectively improves the feature learning and extraction ability in the condition of FSOD.
更多查看译文
AI 理解论文
溯源树
样例
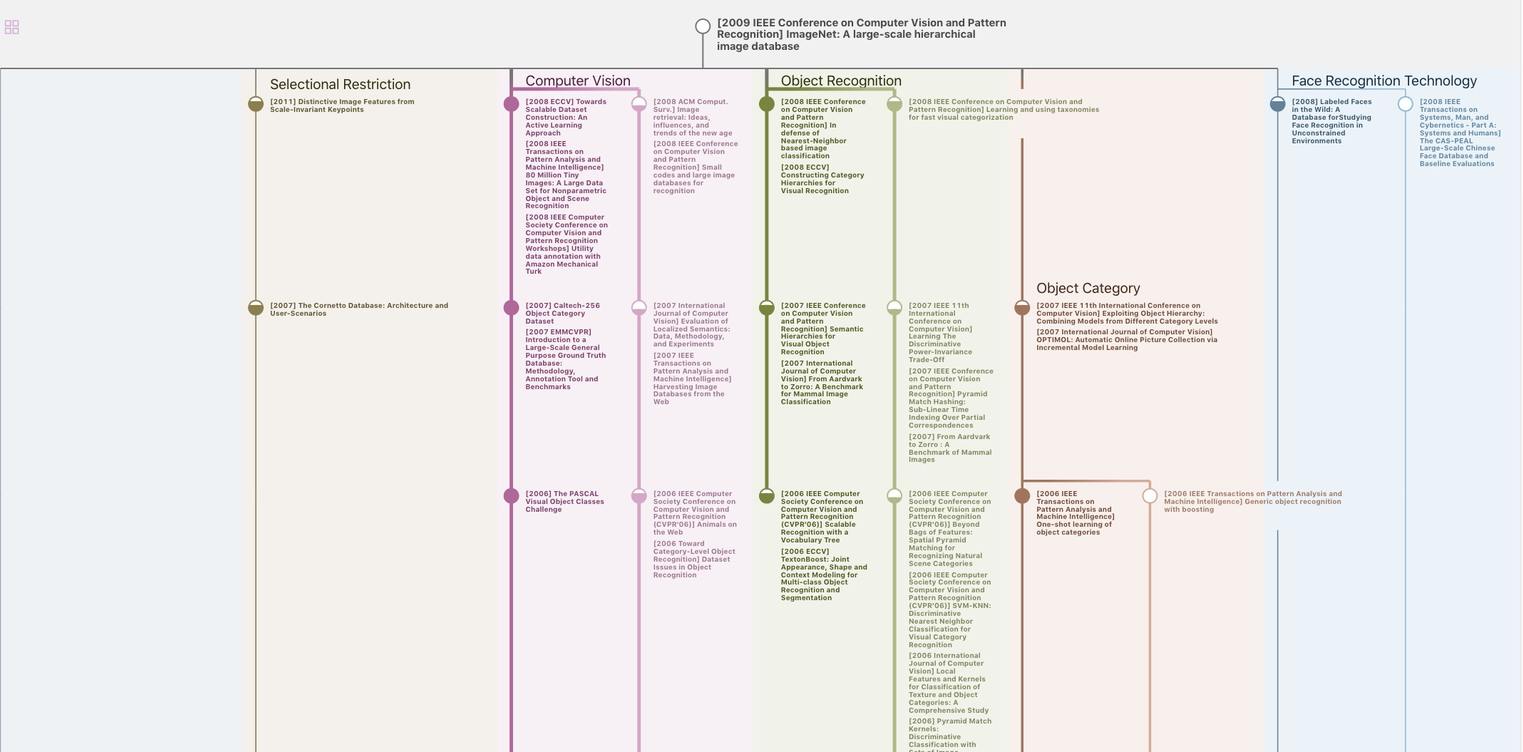
生成溯源树,研究论文发展脉络
Chat Paper
正在生成论文摘要