NNBits: Bit Profiling with a Deep Learning Ensemble Based Distinguisher.
CT-RSA(2023)
摘要
We introduce a deep learning ensemble (NNBits) as a tool for bit-profiling and evaluation of cryptographic (pseudo) random bit sequences. On the one hand, we show how to use NNBits ensemble to explain parts of the seminal work of Gohr [16]: Gohr’s depth-1 neural distinguisher reaches a test accuracy of 78.3% in round 6 for SPECK32/64 [3]. Using the bit-level information provided by NNBits we can partially explain the accuracy obtained by Gohr (78.1% vs. 78.3%). This is achieved by constructing a distinguisher which only uses the information about correct or incorrect predictions on the single bit level and which achieves 78.1% accuracy. We also generalize two heuristic aspects in the construction of Gohr’s network: i) the particular input structure, which reflects expert knowledge of SPECK32/64, as well as ii) the cyclic learning rate. On the other hand, we extend Gohr’s work as a statistical test on avalanche datasets of SPECK32/64, SPECK64/128, SPECK96/144, SPECK128/128, and AES-128. In combination with NNBits ensemble we use the extended version of Gohr’s neural network to draw a comparison with the NIST Statistical Test Suite (NIST STS) on the previously mentioned avalanche datasets. We compare NNBits in conjunction with Gohr’s generalized network to the NIST STS and conclude that the NNBits ensemble performs either as good as the NIST STS or better. Furthermore, we demonstrate cryptanalytic insights that result from bit-level profiling with NNBits, for example, we show how to infer the strong input difference
$$(0x0040, 0x0000)$$
for SPECK32/64 or infer a signature of the multiplication in the Galois field of AES-128.
更多查看译文
关键词
nnbits profiling,deep learning
AI 理解论文
溯源树
样例
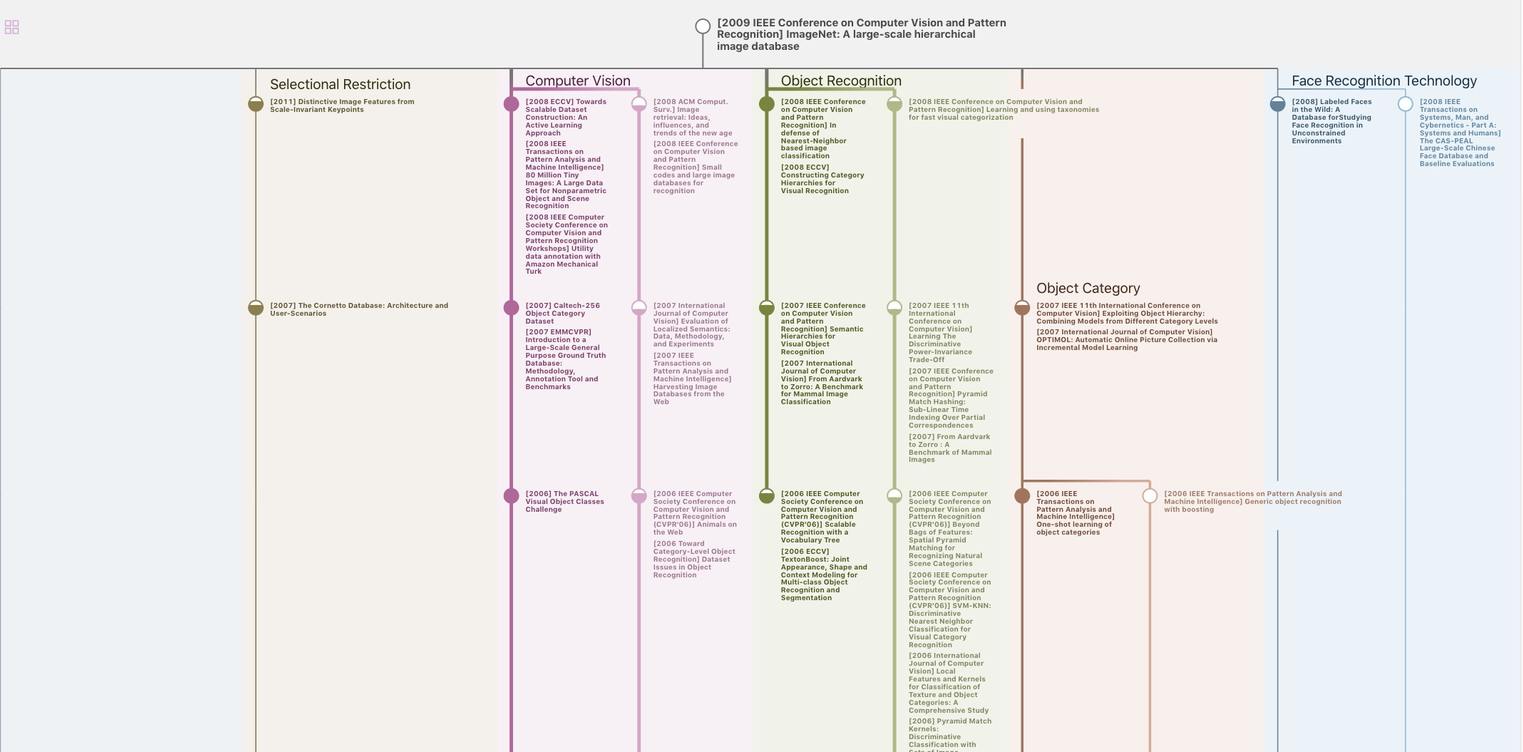
生成溯源树,研究论文发展脉络
Chat Paper
正在生成论文摘要