A novel bias-alleviated hybrid ensemble model based on over-sampling and post-processing for fair classification.
Connect. Sci.(2023)
摘要
With the rapid development of machine learning in the field of classification, the classification fairness has become the research emphasis second to prediction accuracy. However, the data bias and algorithmic discrimination that affect the fair classification of models have not been well resolved, which may damage or benefit the specific groups related to the sensitive attributes (e.g. age, race, and gender). To alleviate the unfairness of the classification model, this study proposes a novel bias-alleviated hybrid ensemble model (BAHEM) based on over-sampling and post-processing. First, a new clustering-based over-sampling method is proposed to reduce the data bias caused by the imbalance in label and sensitive attribute. Then, a stacking-based ensemble learning method is employed to obtain the higher performance and robustness of the BAHEM. Finally, a new classification with alternating normalisation (CAN)-based post-processing method is proposed to further improve the fairness and maintain the accuracy of the BAHEM. Three datasets with different sensitive attributes and four evaluation metrics were used to evaluate the prediction accuracy and fairness of the BAHEM. The experimental results verify the superior fairness of the BAHEM with little accuracy reduction.
更多查看译文
关键词
Machine learning,fair classification,over-sampling,ensemble learning,post-processing
AI 理解论文
溯源树
样例
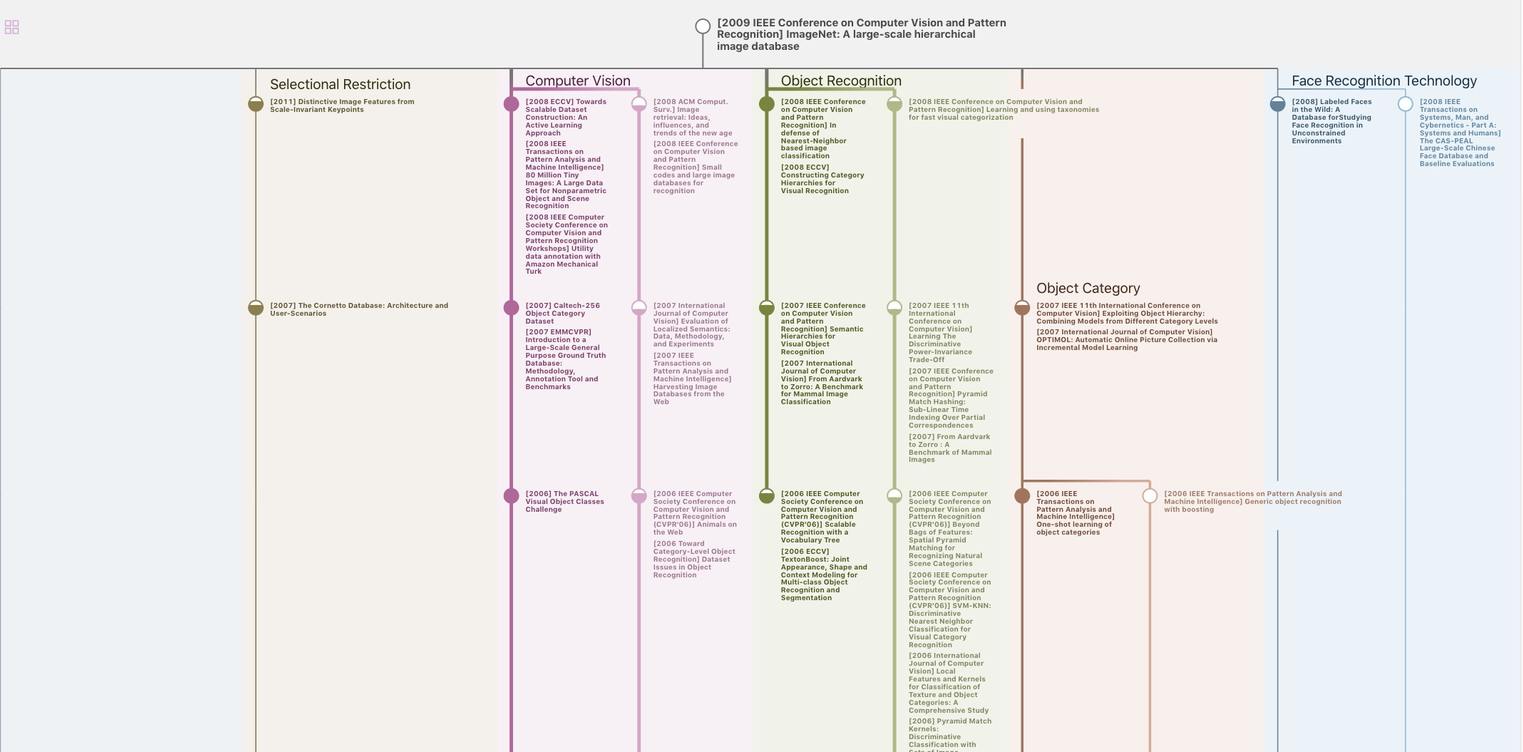
生成溯源树,研究论文发展脉络
Chat Paper
正在生成论文摘要