Network intrusion detection based on n-gram frequency and time-aware transformer.
Comput. Secur.(2023)
摘要
Network intrusion detection system plays a critical role in protecting the target network from attacks. However, most existing detection methods cannot fully utilize the information contained in raw network traffic, such as information loss in the feature extraction process and incomplete feature dimensions, which lead to performance bottlenecks. In this paper, we propose a novel intrusion detection model based on n-gram frequency and time-aware transformer called GTID. GTID can learn traffic features from packet-level and session-level hierarchically and can minimize information as much as possible. To ex-tract packet-level features effectively, GTID considers the different roles of packet header and payload, and processes them in different ways, where n-gram frequency is used to represent payload contextual information because of its conciseness. Then, GTID uses the proposed time-aware transformer to learn session-level features for intrusion detection. The time-aware transformer considers the time intervals between packets, and learns the temporal features of a session for classification. For evaluation, several solid experiments are conducted on the ISCX2012 dataset and the CICIDS2017 dataset, and the results show the effectiveness and robustness of GTID.(c) 2023 Elsevier Ltd. All rights reserved.
更多查看译文
关键词
Intrusion detection, Deep learning, Transformer, N -Gram
AI 理解论文
溯源树
样例
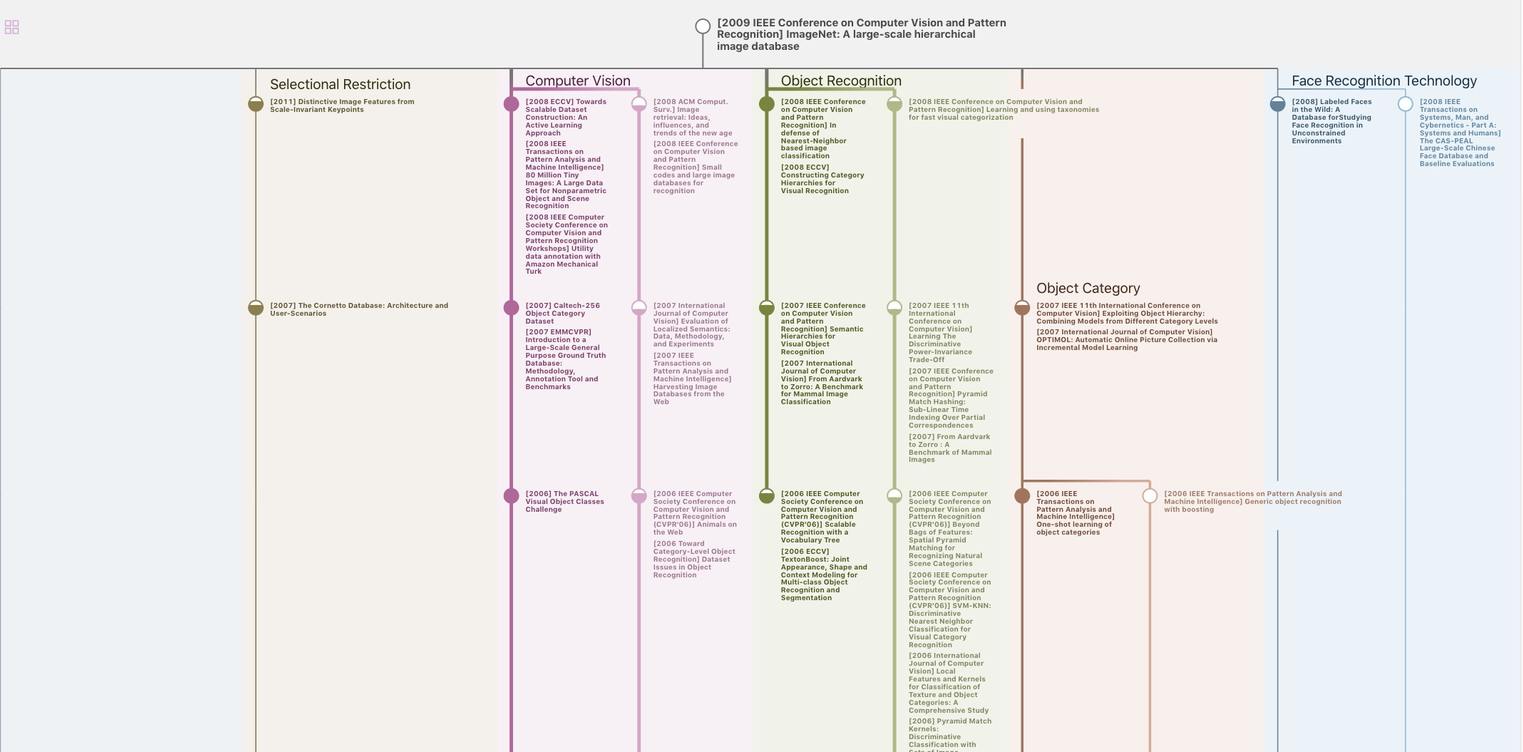
生成溯源树,研究论文发展脉络
Chat Paper
正在生成论文摘要