Semi-supervised multiple evidence fusion for brain tumor segmentation.
Neurocomputing(2023)
摘要
The performance of deep learning-based methods depends mainly on the availability of large-scale labeled learning data. However, obtaining precisely annotated examples is challenging in the medical domain. Although some semi-supervised deep learning methods have been proposed to train models with fewer labels, only a few studies have focused on the uncertainty caused by the low quality of the images and the lack of annotations. This paper addresses the above issues using Dempster-Shafer theory and deep learning: 1) a semi-supervised learning algorithm is proposed based on an image transforma-tion strategy; 2) a probabilistic deep neural network and an evidential neural network are used in parallel to provide two sources of segmentation evidence; 3) Dempster's rule is used to combine the two pieces of evidence and reach a final segmentation result. Results from a series of experiments on the BraTS2019 brain tumor dataset show that our framework achieves promising results when only some training data are labeled.(c) 2023 Elsevier B.V. All rights reserved.
更多查看译文
关键词
Machine learning,Medical image segmentation,Information fusion,Deep learning,Dempster-Shafer theory,Brain tumor segmentation
AI 理解论文
溯源树
样例
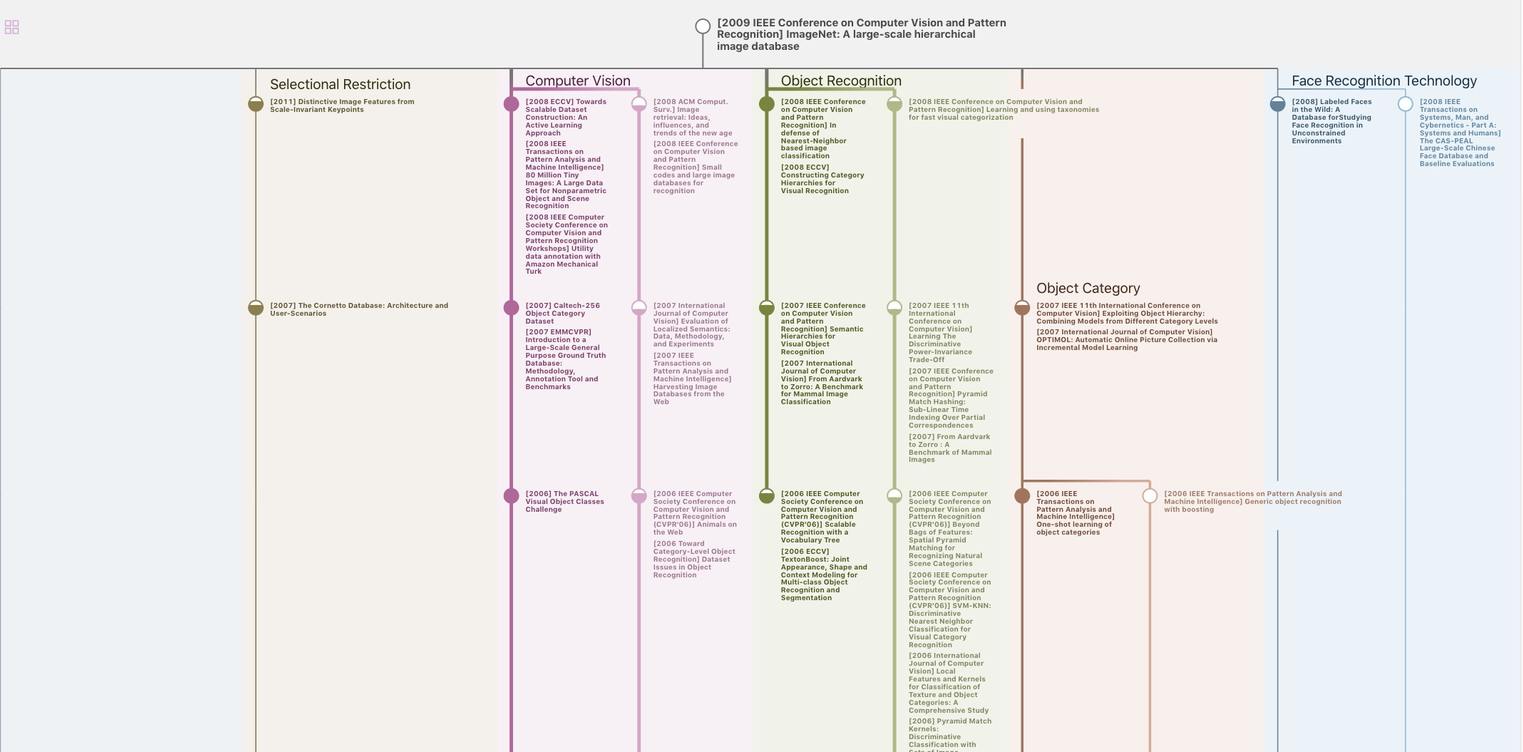
生成溯源树,研究论文发展脉络
Chat Paper
正在生成论文摘要