Deep 6-DoF camera relocalization in variable and dynamic scenes by multitask learning
Mach. Vis. Appl.(2023)
摘要
Recently, direct visual localization with convolutional neural networks has attracted researchers’ attention with achieving an end-to-end process. However, on the one side, the lack of using 3D information leads to imprecise accuracy. Meanwhile, the single input image confuses the relocalization in the scenes that keep similar views at different positions. On the other side, the relocalization problem in variable or dynamic scenes is still challenging. Concentrating on these concerns, we propose two multitask relocalization networks called MMLNet and MMLNet+ for obtaining the 6-DoF camera pose in static, variable and dynamic scenes. Firstly, addressing the dataset lack of variable scenes, we construct a variable scene dataset with a semiautomatic process combining SFM and MVS algorithms with a few manual labels. Based on the process, three scenes covering an office, a bedroom and a sitting room are gathered and generated. Secondly, to enhance the perception between 2D images and 3D poses, we design a multitask network called MMLNet that regresses both camera pose and scene point cloud. Meanwhile, the Chamfer distance is joined into the original pose loss to optimize MMLNet. Moreover, MMLNet learns the pose trajectory feature by using LSTM layers to the additional pose array input, which meanwhile breaks through the limitation of single image input. Based on the MMLNet, aiming at dynamic and variable scenes, MMLNet+ outputs the auxiliary segmentation branch that distinguishes fixed, changeable or dynamic parts of the input image. Furthermore, we define the feature fusion block to implement the feature sharing among three tasks, further promoting the performance in dynamic and variable environments. Finally, experiments on static, dynamic and our constructed variable datasets demonstrate state-of-the-art relocalization performances of MMLNet and MMLNet+. Simultaneously, the positive effects of the pose learning part, reconstruction branch and segmentation task are also illustrated.
更多查看译文
关键词
Image-based localization, Deep learning, Dynamic localization, Multitask learning
AI 理解论文
溯源树
样例
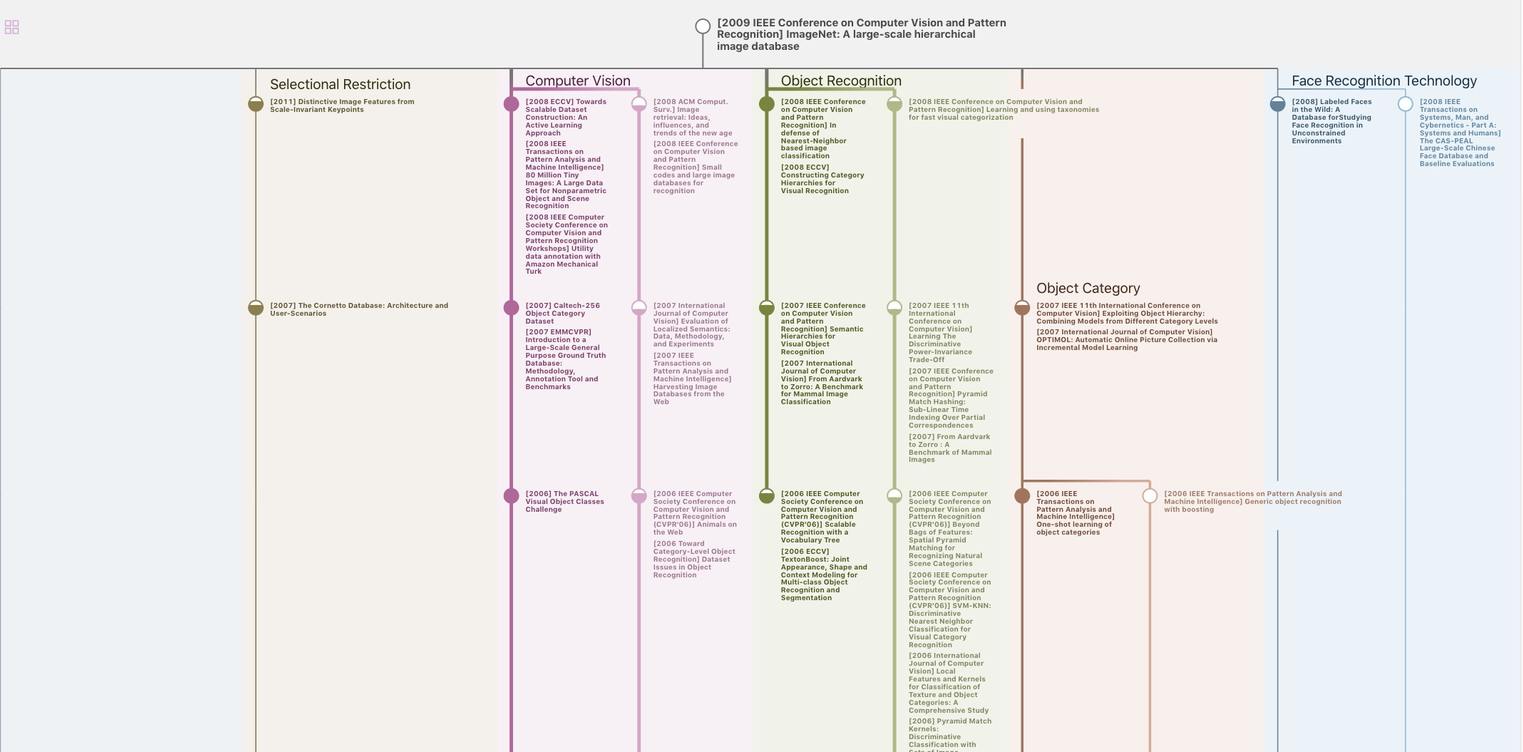
生成溯源树,研究论文发展脉络
Chat Paper
正在生成论文摘要