Dual-Task Interactive Learning for Unsupervised Spatio-Temporal–Spectral Fusion of Remote Sensing Images
IEEE Trans. Geosci. Remote. Sens.(2023)
摘要
Spatio-temporal–spectral fusion aims to produce high spatio-temporal–spectral resolution images by integrating the complementary spatial, temporal, and spectral advantages of multisource remote sensing images. However, on one hand, the existing spatio-temporal–spectral fusion methods are insufficient to exploit the inherent complex nonlinear spatial, temporal, and spectral relationship among multisource and multitemporal observations. On the other hand, since the unavailability of real high spatio-temporal–spectral resolution images, it is difficult to adopt deep learning (DL) methods with supervised training. In this article, we propose an effective unsupervised spatio-temporal–spectral fusion model (USTSFM) with dual-task interactive learning to alleviate these problems. The proposed USTSFM has two branches: the spatio-temporal–spectral mapping (STSM) branch is to describe the temporal relationship, and the spectral super-resolution (SSR) branch is to model the spectral relationship. Moreover, the spatial–spectral interaction compensation block is designed to make the two branches compensate and benefit from each other. This intrinsically related and mutually facilitated strategy allows the USTSFM to sufficiently exploit the inherent spatial, temporal, and spectral relationship. In addition, a shared reconstruction module is meticulously designed for the two tasks, which not only reduces the parameters but also allows the supervised task to guide the convergence of the unsupervised task, boosting the stability of unsupervised training. The qualitative and quantitative results demonstrated that the proposed USTSFM has richer spatial details and more accurate predictions than the other state-of-the-art methods.
更多查看译文
关键词
remote sensing,dual-task,spatio-temporal-spectral
AI 理解论文
溯源树
样例
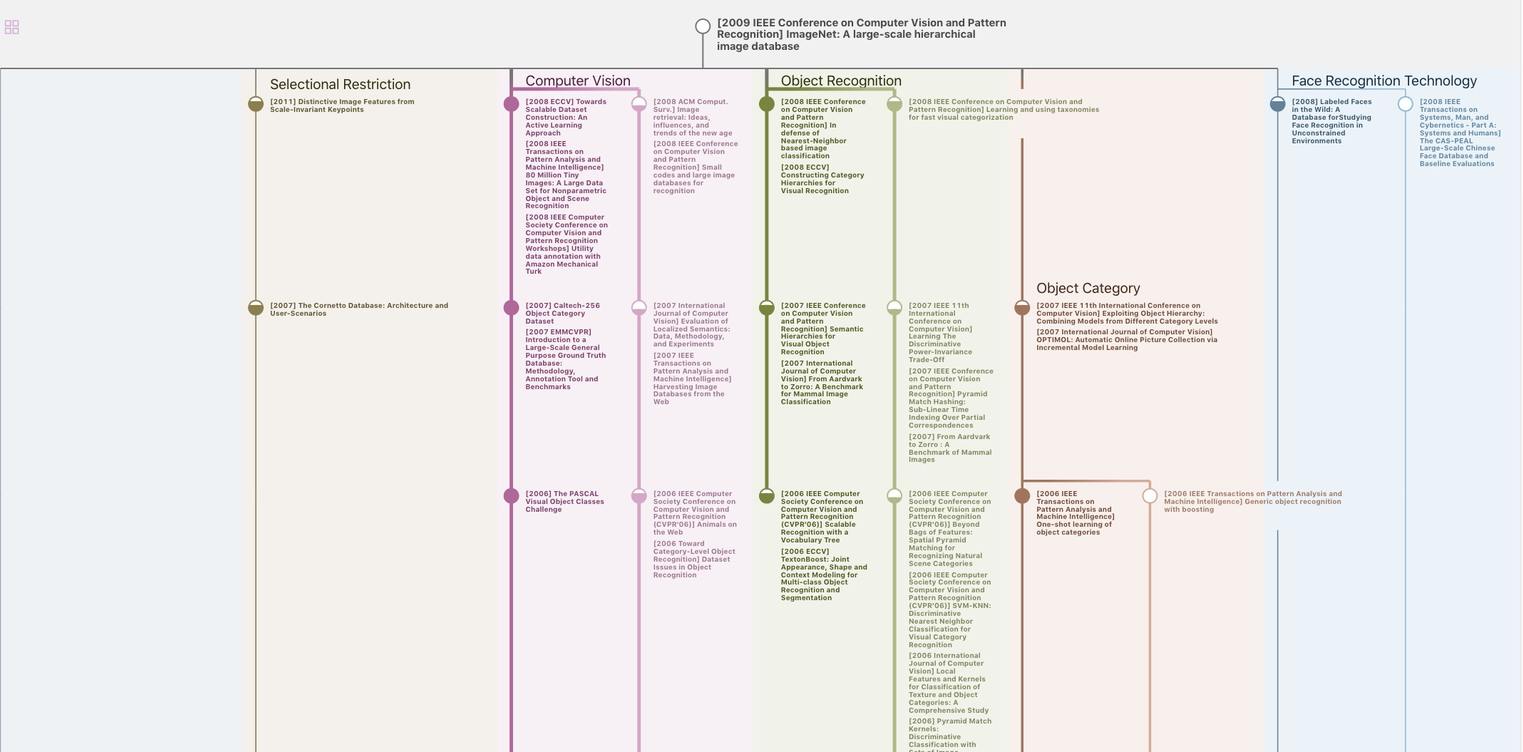
生成溯源树,研究论文发展脉络
Chat Paper
正在生成论文摘要