Multilayer Perceptron and Bayesian Neural Network-Based Elastic Implicit Full Waveform Inversion.
IEEE Trans. Geosci. Remote. Sens.(2023)
摘要
We introduce and analyze the elastic implicit full waveform inversion (EIFWI) of seismic data, which uses neural networks to generate elastic models and perform full waveform inversion. EIFWI carries out inversion by linking two main networks: a neural network that generates elastic models and a recurrent neural network to perform the modeling. The approach is distinct from conventional waveform inversion in two key ways. First, it reduces reliance on accurate initial models relative to conventional FWI. Instead, it invokes general information about the target area, for instance, estimates of means and standard deviations of medium properties in the target area or, alternatively, well-log information in the target area. Second, iterative updating directly affects the weights in the neural network rather than the elastic model. Elastic models can be generated in the first part of the EIFWI process in either of two ways: through the use of a multilayer perceptron (MLP) network or a Bayesian neural network (BNN). Numerical testing is suggestive that the MLP-based EIFWI approach in principle builds accurate models in the absence of an explicit initial model, and the BNN-based EIFWI can give the uncertainty analysis for the prediction results.
更多查看译文
关键词
multilayer perceptron,elastic implicit,bayesian neural network,inversion,waveform
AI 理解论文
溯源树
样例
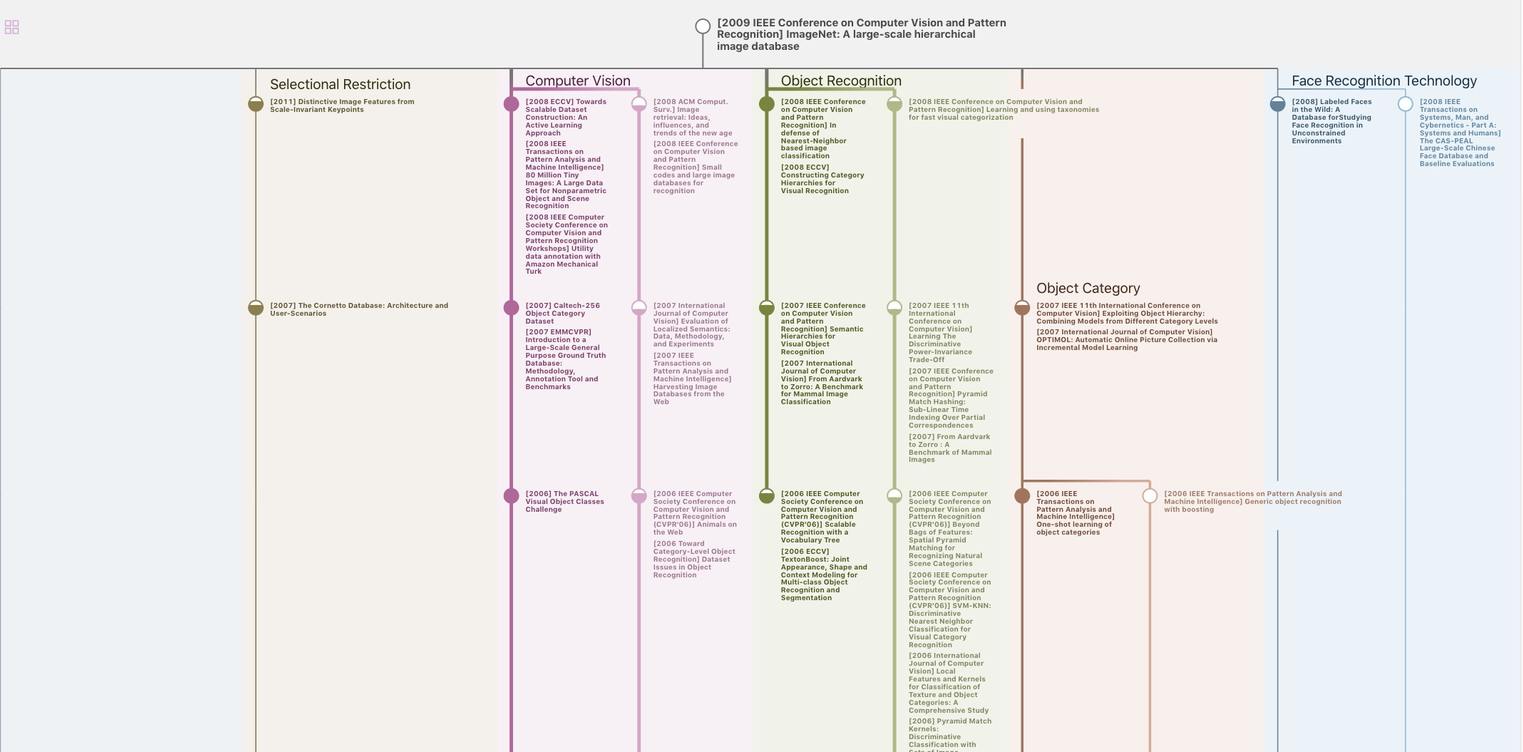
生成溯源树,研究论文发展脉络
Chat Paper
正在生成论文摘要