Federated Learning to Safeguard Patients Data: A Medical Image Retrieval Case.
Big Data Cogn. Comput.(2023)
摘要
Healthcare data are distributed and confidential, making it difficult to use centralized automatic diagnostic techniques. For example, different hospitals hold the electronic health records (EHRs) of different patient populations; however, transferring this data between hospitals is difficult due to the sensitive nature of the information. This presents a significant obstacle to the development of efficient and generalizable analytical methods that require a large amount of diverse Big Data. Federated learning allows multiple institutions to work together to develop a machine learning algorithm without sharing their data. We conducted a systematic study to analyze the current state of FL in the healthcare industry and explore both the limitations of this technology and its potential. Organizations share the parameters of their models with each other. This allows them to reap the benefits of a model developed with a richer data set while protecting the confidentiality of their data. Standard methods for large-scale machine learning, distributed optimization, and privacy-friendly data analytics need to be fundamentally rethought to address the new problems posed by training on diverse networks that may contain large amounts of data. In this article, we discuss the particular qualities and difficulties of federated learning, provide a comprehensive overview of current approaches, and outline several directions for future work that are relevant to a variety of research communities. These issues are important to many different research communities.
更多查看译文
关键词
safeguard patients data,learning
AI 理解论文
溯源树
样例
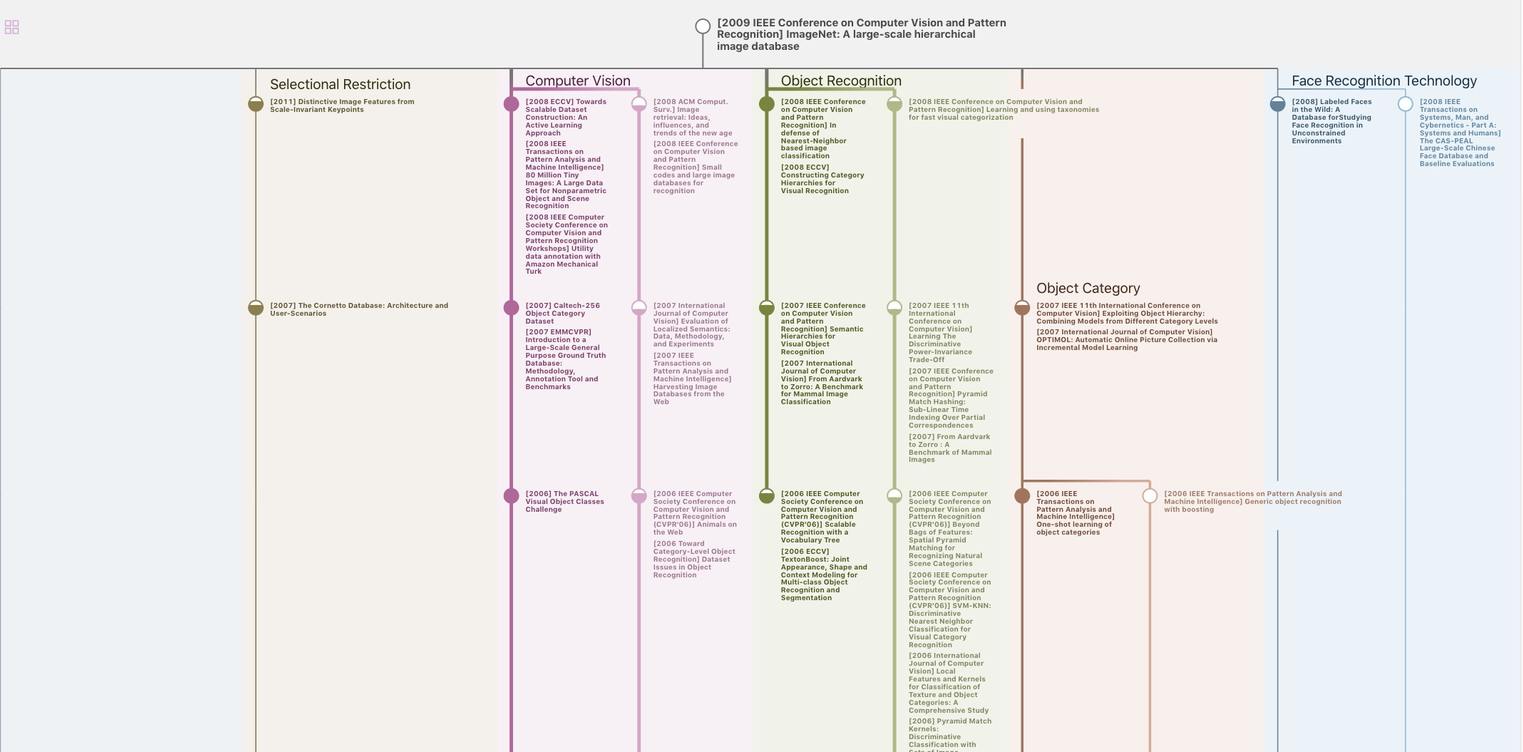
生成溯源树,研究论文发展脉络
Chat Paper
正在生成论文摘要